sgRNA Off-Target Prediction: A Comprehensive Exploration
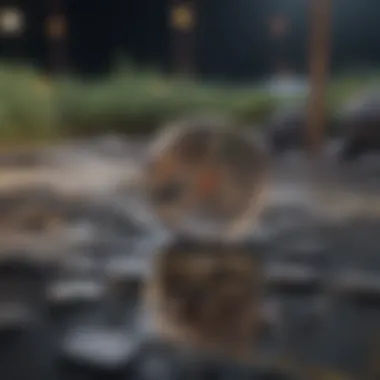
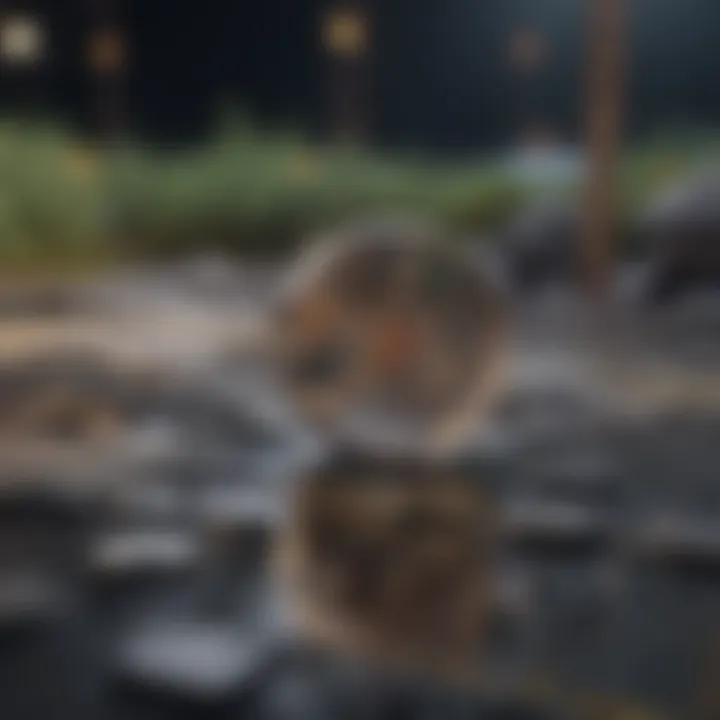
Intro
The exploration of off-target effects is intrinsic to the advancement of CRISPR technology. As the popularity of genome editing rises, it becomes increasingly crucial to understand how single guide RNA (sgRNA) can sometimes bind to unintended targets in the genome. Accurately predicting these off-target effects not only ensures the integrity of gene editing but also reflects on the broader implications of precision medicine and genetic engineering.
This article provides a thorough investigation into the methodologies and predictive tools relevant for off-target effects associated with sgRNA. We will examine the foundational concepts, explore current methodologies, and elucidate the challenges faced in this field, all while maintaining a focus on the significance of these findings for future scientific endeavors.
Key Findings
Major Results
One of the substantial findings in the sgRNA off-target prediction landscape is the variability in prediction accuracy among different tools. The established methodologies often yield divergent results, which complicates the process of selecting appropriate sgRNAs for specific genomic targets. Tools such as CRISPRtarget and CCTop are commonly referenced, each offering unique algorithms and scoring systems that are designed to analyze potential off-target sites based on sequence similarity.
Additionally, empirical studies show that empirical validation is frequently required to confirm predictions made by computational tools. These discrepancies carry significant implications, urging researchers to adopt a dual approach involving both computational predictions and laboratory validation.
Discussion of Findings
The findings emphasize a critical point: reliance solely on computational predictions can lead to inaccuracies. Researchers must exercise caution and consider the biological context in which gene editing occurs. Furthermore, as genome editing technologies evolve, the need for more refined predictive models becomes apparent. Continuous updates to databases and algorithms are essential to keep pace with emerging genetic information and techniques.
"Accurate off-target prediction remains a cornerstone of responsible CRISPR applications, influencing everything from basic research to therapeutic developments."
Methodology
Research Design
In exploring sgRNA off-target prediction, a mixed-methods approach is employed. This design incorporates both qualitative and quantitative analyses of existing prediction tools. By evaluating tool performance using empirical data collected from various research studies, we assess their efficacy in real-world applications.
Data Collection Methods
Data for this analysis is drawn from multiple sources:
- Peer-reviewed journals reporting empirical studies on CRISPR applications.
- Online databases, such as those maintained by en.wikipedia.org and britannica.com, which provide foundational information about sgRNA and off-target effects.
- Community discussions on platforms like reddit.com, allowing access to firsthand experiences from researchers who use these tools in their labs.
Each data source contributes to a multifaceted understanding of how off-target effects are predicted and validated, shaping the future landscape of this critical field in genetic engineering.
This comprehensive exploration will guide readers through the complexities of sgRNA off-target prediction, illuminating both the tools at our disposal and the necessity for precise methodologies.
Preamble to sgRNA and Genome Editing
The advent of genome editing technologies has revolutionized the fields of molecular biology and genetic engineering. Understanding single guide RNA (sgRNA) is fundamental for grasping the nuances of these technologies. The importance of sgRNA lies not only in its role in the CRISPR-Cas9 system but also in its ability to target specific genomic locations. The precision it offers in editing genes brings enormous potential for advancements in medicine, agriculture, and biological research.
The discourse on sgRNA entails various technical facets including its design, delivery, and the genetic modifications it evokes. Likewise, the increasing accuracy in gene editing techniques promises to minimize undesirable off-target effects, which can lead to unintended changes in the genome. A thorough comprehension of sgRNA is thus indispensable for practitioners and researchers alike, as it allows for informed decisions in experimental design and application.
Basic Concepts of sgRNA
sgRNA is a short sequence of RNA that complements a specific DNA sequence in the genome. Functionally, it guides the Cas9 protein to the right location for editing. The design of an effective sgRNA is paramount since its specificity determines the efficacy of the CRISPR system. Researchers typically consider factors such as the target sequence, secondary structures, and potential off-target sites during sgRNA design.
In practical applications, successful sgRNA should induce minimal off-target activities. High-fidelity sgRNA variants have been developed to enhance specificity and reduce unintended interactions.
Overview of Genome Editing Technologies
The landscape of genome editing is diverse, encompassing various technologies apart from CRISPR. Technologies such as Transcription Activator-Like Effector Nucleases (TALENs) and Zinc Finger Nucleases (ZFNs) also have significant applications. Each technique offers distinct mechanisms for introducing double-strand breaks in DNA, facilitating repair and modification.
The CRISPR-Cas9 system stands out due to its simplicity and efficiency. However, its widespread use brings challenges in ensuring precision, particularly concerning off-target edits.
Understanding Off-Target Effects
Understanding off-target effects is fundamental within the realm of genome editing technologies, particularly when employing single guide RNA (sgRNA) systems. In the context of CRISPR technology, off-target effects refer to unintended modifications that may occur in the genome, potentially leading to undesirable outcomes. This understanding forms the backbone of precision in genetic engineering, where the goal is to optimize on-target effects while minimizing off-target modifications. As the field advances, grasping the implications of these effects becomes critically important for researchers and practitioners alike.
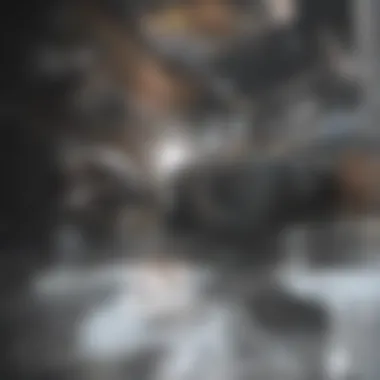
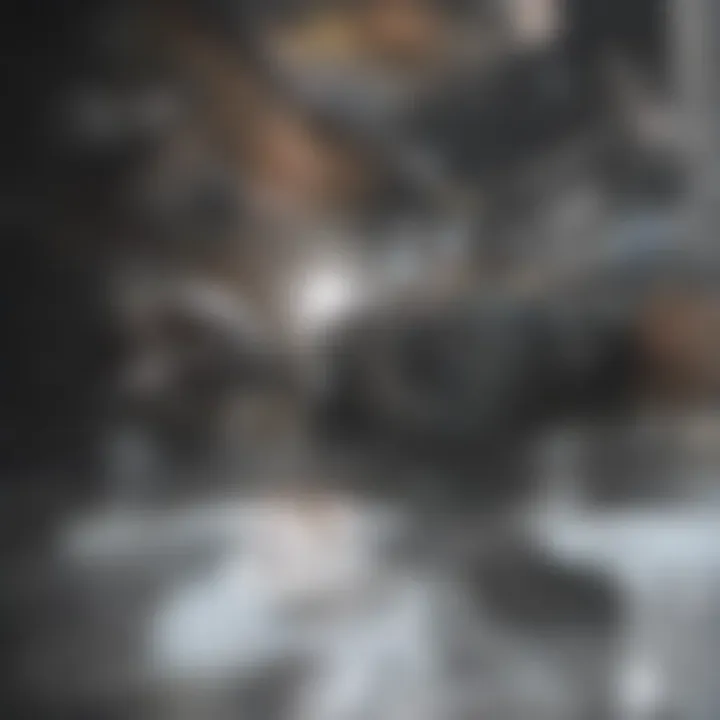
The significance of off-target effects transcends beyond mere technical challenges; it poses ethical and safety concerns in applications ranging from agricultural enhancements to therapeutic interventions in humans. Acknowledging and accurately predicting these effects can mitigate risks, ensuring that genetic modifications do not lead to unforeseen negative consequences. This section will delve into the definitions and classifications of off-target effects, laying a vital groundwork for understanding their implications.
Defining Off-Target Effects
Off-target effects are defined as unintended alterations that occur at non-targeted sites in the genome during the use of CRISPR-Cas9 or related technologies. These can arise due to the sgRNA binding to sequences that resemble the target sequence but are located elsewhere in the genomic landscape. The consequence of such binding can lead to cuts or edits, which can disrupt important genes or regulatory regions. It is crucial to distinguish between actual off-target effects and related phenomena such as multiple guide delivery to a target site which may not cause functional harm.
Research indicates that even minor mismatches between the sgRNA and the non-target sequence may still facilitate off-target edits. Hence, controlling for these effects requires a thorough understanding of not only the targeted sequence but also the overall genomic context. The precision of sgRNA design, therefore, becomes paramount in minimizing potential off-target issues.
Types and Categories of Off-Target Effects
Off-target effects can be categorized into several types based on the nature of the unintended modifications they provoke. Understanding these various categories can guide researchers in developing more efficient prediction strategies. Below are the prominent classifications:
- Sequence Mismatch Induced Edits: Occur when the sgRNA binds to similar but non-identical genome sequences, leading to unintended cleavages.
- Structural Variations: Result from larger genomic rearrangements triggered by the modification process, potentially complicating the expression of adjacent genes.
- Insertional Mutagenesis: Associated with the integration of foreign DNA sequences at off-target sites, which could disrupt local genomic architecture and gene expression.
- Altered Gene Regulation: Off-target effects that affect regulatory regions, leading to changes in gene expression patterns without altering the nucleotide sequence itself.
Knowledge of these types of off-target effects aids in constructing more sophisticated prediction models. They are pivotal to maximizing the safety and efficacy of CRISPR applications. Understanding the landscape of potential unintended consequences fosters a more responsible approach to genetic engineering endeavors.
Importance of Off-Target Prediction
The significance of off-target prediction in the context of sgRNA is profound, especially when considering the implications for genetic modification and therapeutic practices. Off-target effects occur when genomic alterations are made at unintended sites, leading to potentially harmful consequences. Therefore, precision in prediction becomes crucial. By accurately identifying possible off-target sites, researchers can enhance the safety and efficacy of genome editing technologies.
Predicting off-target effects allows for a decrease in unintentional gene modifications that can lead to adverse effects, including those that could manifest as genetic disorders or unintended phenotypic traits. In fields such as agriculture, off-target effects can significantly impact crop yields, pest resistance, or nutritional value. Addressing these predictions ensures that the desired traits are accurately expressed while minimizing unintended changes.
Many researchers are drawn to the promise of genome editing technologies, yet concerns regarding off-target effects can limit their application, particularly in clinical scenarios. Therefore, effective off-target prediction directly correlates with the advancement of genetic engineering and its acceptance in therapeutic contexts.
Implications for Genetic Engineering
In genetic engineering, off-target predictions play an essential role in guiding the development and application of editing tools. First, they provide a framework for risk assessment. Understanding where off-target modifications may occur helps in assessing whether the genetic changes could pose health risks or impact gene function adversely.
Moreover, predictive tools contribute to the rational design of sgRNAs. By evaluating the predicted off-target sites, scientists can select guide RNAs that have fewer potential interactions with unintended genomic loci. This strategic selection fosters a more efficient gene editing process and maximizes the likelihood of successful modifications.
- Risk Management: Identifying off-target effects early in the research process supports informed decision-making.
- Guide RNA Optimization: Enhancing sgRNA design reduces risks associated with off-target activities, which is critical for the future of safe genetic interventions.
Relevance in Therapeutic Applications
The relevance of off-target prediction extends to therapeutic applications, particularly within the scope of CRISPR-based gene therapies. Off-target effects in somatic cells could have dire consequences, such as the induction of oncogenes or the suppression of tumor suppressor genes. These unintended modifications could potentially negate the therapeutic benefits aimed by the precise editing of malignant alleles.
Predictions help in several therapeutic scenarios including:
- Clinical Trials: Rigorous off-target evaluation is a key factor in determining the safety profiles necessary for progressing to clinical trials.
- Personalized Medicine: Off-target assessments enable the tailoring of gene therapies to individual patient genotypes to maximize effectiveness while minimizing adverse effects.
"The ability to predict off-target effects can significantly increase the therapeutic window and promote the safe application of genome editing technologies."
Methodologies for sgRNA Off-Target Prediction
Understanding the various methodologies employed in sgRNA off-target prediction is essential. These methods not only gauge the precision of genome editing but also inform potential risks associated with off-target effects. As researchers strive for enhanced accuracy in genetic engineering, effective methodologies become the backbone of successful implementations.
Methodologies for predicting off-target effects can broadly be divided into computational approaches and experimental validation techniques. Each of these methodologies offers unique advantages and considerations that can significantly impact the efficiency of sgRNA designs.
Computational Approaches
Computational approaches leverage algorithms and databases to identify potential off-target sites. These methods often rely on sequence homology to predict unintended targets that may be modified during genome editing. Some common computational tools and techniques include:
- BLAST (Basic Local Alignment Search Tool): This widely used algorithm compares the sgRNA sequence against reference genomes to identify regions of high similarity.
- CRISPR Design Tools: Tools such as CHOPCHOP and CRISPRdirect allow researchers to design sgRNAs with minimized off-target effects. These tools rank sgRNA candidates based on predicted target specificity.
- Machine Learning Models: Advanced computational methods utilize machine learning to analyze large datasets, learning from known off-target data to refine predictions.
While these tools offer rapid predictions, they are not without limitations. High false positivity rates can arise due to sequence similarities across the genome. Thus, computational methods should be viewed as initial screenings rather than definitive solutions. The integration of multiple computational techniques can help enhance reliability, providing researchers with comprehensive data on potential off-target sites.
Experimental Validation Techniques
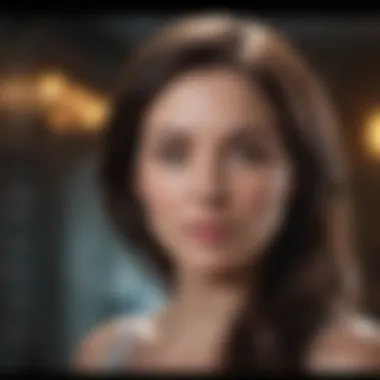
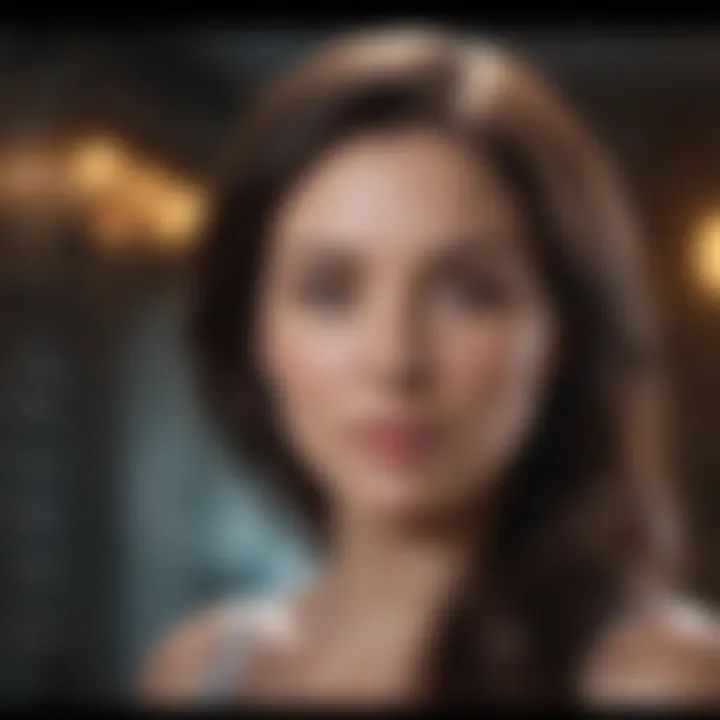
Experimental validation techniques serve as a critical follow-up to computational predictions. These methods allow researchers to directly assess whether predicted off-target effects occur in biological systems. Key experimental techniques include:
- Deep Sequencing: By sequencing edited genomes, researchers can identify unintended modifications at previously predicted off-target sites. This technique provides high-resolution data.
- CUT&RUN and CUT&Tag: These methods enable the identification of off-target interactions through the selective capture of genomic regions bound by the Cas9-sgRNA complex, followed by sequencing.
- Cell-based Assays: Functional assays can help evaluate the phenotypic consequences of off-target effects. Observing the resulting changes in cellular behavior provides insight into off-target impacts.
Despite the robustness of experimental techniques, challenges remain. Variability in biological responses and the complexity of the genome can lead to discrepancies between predicted and actual off-target effects. Therefore, a combined approach utilizing both computational and experimental methodologies is essential to maximize accuracy in sgRNA design.
In summary, while computational approaches offer a preliminary assessment of off-target risks, experimental validation is imperative for confirming predictions. Together, these methodologies form a comprehensive framework for sgRNA off-target prediction, enhancing the reliability of gene editing technologies.
Tools for Off-Target Prediction
Understanding the tools available for sgRNA off-target prediction is crucial for any researcher or practitioner involved in genome editing. These tools empower scientists to identify potential off-target sites that could lead to unintended consequences in gene modification. The effectiveness of a genetic experiment hinges significantly on the choice of prediction tools used.
These tools can analyze sgRNA sequences against a target genome, predict potential off-target sites, and evaluate the likelihood of unintended edits. Researchers often face the challenge of balancing accuracy with speed since predicting off-target effects can be computationally intensive. Therefore, it is essential to select tools based on the specific requirements of a project.
Overview of Prediction Tools
Several software tools have been created specifically for the purpose of off-target prediction. Some of these tools include:
- Cas-OFFinder: A versatile tool that allows users to identify potential off-target sites based on user-defined parameters.
- CRISPOR: This integrates various prediction algorithms and provides a user-friendly web interface, making it accessible for many scientists.
- Off-Spotter: Focused on identifying potential off-targets given sequence and structural features, which enhances prediction accuracy.
These tools typically use different algorithms, such as scoring systems and machine learning techniques, to estimate off-target likelihood based on the similarity of sgRNA sequences to the genome. Understanding the specifics of each tool helps in optimizing the accuracy of predictions.
Comparative Analysis of Popular Tools
When choosing a prediction tool, it is valuable to compare the available options based on several criteria:
- Accuracy: The ability of the tool to predict true off-target effects needs to be assessed through independent validation.
- Ease of Use: Since not all users may have extensive computational backgrounds, the user interface plays a role in tool selection.
- Computational Efficiency: Some tools are faster than others, processing large datasets in a more time-efficient manner.
- Comprehensiveness: Tools that integrate additional features, such as experimental validation, tend to provide more robust answers.
For example, while Cas-OFFinder is praised for its specificity, CRISPOR offers a one-stop solution that includes essential features to aid researchers in their decision-making.
Limitations of Existing Tools
Despite the advancements in sgRNA off-target prediction tools, several limitations persist:
- False Positives: Many tools have a tendency to generate false positives, highlighting potential off-target sites that may not be biologically relevant.
- Lack of Standardization: Different tools may employ varying algorithms, which can lead to inconsistent results across different platforms. This lack of standardization makes it challenging for researchers to trust a single tool's predictions.
- Data Dependency: The accuracy of predictions often relies heavily on the quality of the reference genome and the dataset used to train the models. Limited genomic data from certain organisms can pose a significant challenge.
"The reliability of sgRNA off-target prediction tools is fundamentally tied not only to their algorithms but also to the underlying biological assumptions they make."
In light of these issues, ongoing research and development must focus on refining these prediction methodologies to ensure greater accuracy and reliability in off-target assessments. Tools are evolving, but understanding limitations is critical for effective use.
Challenges in Off-Target Prediction
Off-target prediction in sgRNA applications is not a straightforward task. It presents multiple complexities that researchers must navigate for reliable outcomes. Understanding these challenges is critical. They affect the accuracy of gene editing and the overall success of genetic engineering projects. Identifying off-target effects is not just about evaluating sequences; it requires a multifaceted approach considering numerous variables.
Technical Hurdles
One of the predominant challenges is the technical limitations inherent in current prediction methods. Many algorithms lack sensitivity or specificity when it comes to identifying potential off-target sites. Different algorithms, like Cas-OFFinder or CRISPRseek, employ diverse strategies, and their performances vary significantly based on the target organism and the sgRNA used. Additional issues arise from the representation of genetic sequence data and computational resource constraints. For instance, long sequences may complicate analysis, leading to gaps in detection. Moreover, each new technology brings its own set of assumptions, which can skew results if not appropriately considered.
Biological Variabilities
Biological variabilities introduce yet another layer of complication. Individual genetic variation can lead to discrepancies in how sgRNA binds to various DNA sites. Factors such as chromatin accessibility, DNA secondary structures, and the physiological context of cells also play crucial roles. For example, the same sgRNA may operate distinctly in different cell types or under varying environmental conditions. This means that predictions made in one context may not hold true in another, reducing the relevance of certain findings. The interplay of these biological elements often results in uncertain off-target effects, making it challenging to develop universally applicable predictive models.
Data Integration Issues
Lastly, data integration poses considerable challenges in off-target prediction. The vast amount of data generated from various studies is often not comparable due to differences in methodologies and reporting standards. Combining genomic data from various sources while ensuring consistency and reliability is complex. This integration is essential for drawing comprehensive conclusions and refining predictive algorithms. Inadequate integration can result in misleading conclusions, limiting the utility of predictive tools in practical applications.
"The complexity of off-target prediction necessitates a thorough understanding of both technical and biological factors, as well as the seamless integration of diverse datasets."
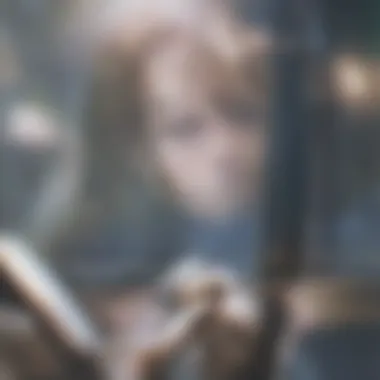
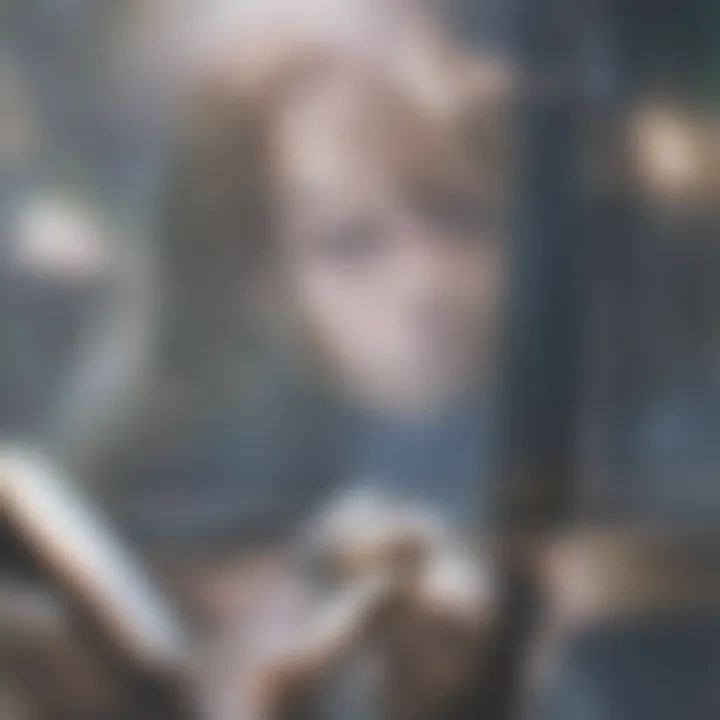
In summary, navigating the challenges associated with off-target prediction is essential for enhancing the efficacy of sgRNA technologies. Addressing these hurdles can pave the way for more accurate predictions and ultimately more successful applications in genetic engineering.
Future Directions in Off-Target Prediction
As genome editing technologies evolve, the quest for higher precision in predicting off-target effects is more crucial than ever. The future of sgRNA off-target prediction hinges on leveraging cutting-edge methodologies and technologies. Understanding these future directions not only enriches the academic discourse but also directly impacts practical applications in therapeutic settings and agricultural advancements. The focus is squarely on enhancing accuracy, reducing unintended modifications, and integrating novel predictive frameworks.
Emerging Technologies
Emerging technologies are reshaping the landscape of sgRNA off-target prediction. Here are some key advancements to watch:
- Machine Learning Algorithms: As the field of artificial intelligence progresses, machine learning models are being developed to analyze vast datasets for off-target predictions. These models can learn from existing data, refine predictions, and improve over time.
- Next-Generation Sequencing (NGS): NGS offers deeper insights into genomic variations. Utilizing this technology can enable researchers to validate off-target effects on a broader scale, increasing the reliability of predictions.
- CRISPR-Cas Variants: New CRISPR systems and their Cas proteins are continuously being discovered. Each variant has unique properties that can affect specificity. Understanding these differences can lead to better-targeted sgRNA designs with fewer off-target chances.
The integration of these technologies provides a promising outlook for enhancing the accuracy of off-target predictions, which is essential for the safe deployment of genome editing in biological systems.
Integrative Approaches for Prediction
An integrative approach to off-target prediction combines multiple methodologies to increase prediction accuracy. Here are some significant concepts to consider:
- Combination of Experimental and Computational Methods: This hybrid model helps bridge the gap between theoretical predictions and real-world outcomes. While computational tools can forecast potential off-target sites, experimental validation ensures findings are corroborated in vivo.
- Cross-Species Comparison: Analyzing sgRNA activity across different species can reveal conserved off-target sites. Insights gained from varied genetic backgrounds can refine predictions and offer broader applicability.
- Multi-Omics Integrations: Combining genomics, transcriptomics, proteomics, and epigenomics can lead to a holistic view of gene expression and modifications. Understanding complex interactions among these layers can aid in improving off-target prediction models.
In summary, these integrative approaches unlock a path toward more reliable predictions by considering multiple biological dimensions and leveraging various technological advancements.
The future of sgRNA off-target prediction lies in the synergy of new technologies and integrative methodologies, leading to safer genome editing applications.
Case Studies of Off-Target Predictions
Understanding off-target predictions through real-world examples provides valuable insights into their significance in genome editing. By analyzing specific case studies, researchers can identify best practices and recognize potential pitfalls. This exploration enhances our understanding of sgRNA applications and informs future endeavors in genetic engineering.
Success Stories in Research
Several notable research endeavors exemplify successful off-target predictions, resulting in advances in science and technology. One such success is the work done on mouse embryonic stem cells where an sgRNA targeting the Ccr5 gene was utilized. Researchers successfully demonstrated how precise targeting led to the desired gene edits while minimizing unintended modifications. This study highlighted the effectiveness of in silico prediction models, which allowed scientists to anticipate and avoid off-target sites. The accuracy of the predictions enabled the development of improved therapeutic strategies for diseases associated with that gene.
Another example comes from a study focused on human hematopoietic stem cells. Here, off-target effects were meticulously monitored as researchers aimed to correct a genetic disorder. The prediction tools used allowed for thorough screening, ensuring that only the intended cells showcased the required edits. This led to significant encouragement in the medical community towards using CRISPR for gene therapy applications.
These cases illustrate that successful off-target prediction can lead to genuine progress. They reinforce the necessity of robust prediction methodologies, underpinning the advancement of gene editing technologies.
Lessons Learned from Failed Predictions
Despite successes, there are also valuable lessons derived from unsuccessful off-target predictions. Understanding these failures is crucial for the evolution of sgRNA applications. One infamous case involved *the editing of the PTEN gene, which aimed to suppress cancer cell growth. Even with thorough predictions, unintended mutations occurred in unrelated genes, leading to adverse effects on cellular development and function. This situation emphasized the complexities of biological systems that can influence gene editing outcomes and the limitations of current prediction tools.
Additionally, another case revealed that even a single nucleotide mismatch in sgRNA could lead to off-target effects that were not predicted. In this instance, researchers targeting the genes involved in cystic fibrosis experienced unexpected complications due to misalignment with similar genetic sequences. Such failures underscore the need for enhanced precision in sgRNA design and the necessity of continual revision to predictive algorithms.
These lessons serve as reminders of the challenges inherent in the field. They compel researchers to refine their methodologies, ensuring that fuzzy predictions do not deter the pursuit of innovative advancements. Ultimately, acknowledging both successes and failures helps shape the future of genome editing, pushing forward the quest for precision in genetic engineering.
Ending
As we conclude our exploration of sgRNA off-target prediction, it is vital to underscore the importance of this topic within the realm of genome editing. Off-target effects can lead to unintended genetic modifications, which may have serious implications for both research and therapeutic applications. Therefore, understanding and accurately predicting these effects is crucial for driving future advancements in genetic engineering.
Recap of Key Insights
In reviewing the key points, we highlighted several important aspects:
- Off-target effects can occur due to sequence similarities between the intended target and other genomic regions, leading to unintended edits.
- Various methodologies exist for predicting off-target events, including computational and experimental approaches that enhance the reliability of sgRNA designs.
- Numerous tools are available for off-target prediction, yet each tool has its limitations, making it essential to use a combination of methods for optimal outcomes.
- The challenges faced in off-target prediction include technical hurdles, biological variabilities, and issues in data integration, which must be addressed in future research.
This analysis reveals the intricate nature of sgRNA off-target prediction, emphasizing the need for continued investigation and improvement in methodologies and tools available for researchers.
The Path Forward in sgRNA Applications
Looking ahead, the future of sgRNA applications holds promise with emerging technologies that can enhance prediction accuracy. Integrative approaches that combine both computational models and experimental validations may pave the way for more reliable off-target predictions.
Additionally, as the field progresses, collaboration across disciplines is crucial. By bringing together computer scientists, biologists, and genetic engineers, we can foster innovation in sgRNA design and implementation.
Finally, ongoing research must focus on improving tools that address the limitations identified, particularly in diverse biological contexts. This will ensure that sgRNA technologies can be safely and effectively applied in therapeutic environments, ultimately advancing the potential of gene editing for various applications.
The path forward in sgRNA applications is not solely defined by technological advancements but also by the collaborative efforts to refine our understanding of gene editing intricacies.