Mathematical Statistics: Foundations and Applications
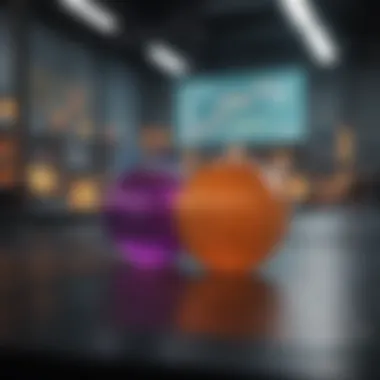
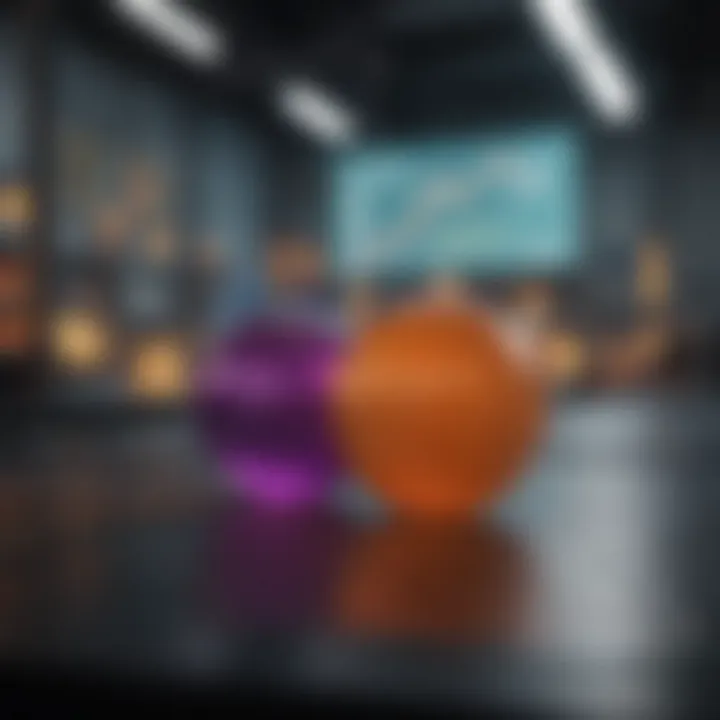
Intro
Mathematical statistics is a cornerstone of data analysis, playing a crucial role in various disciplines. It provides tools for understanding data, drawing inferences, and making predictions. In this article, we will explore the fundamental concepts of mathematical statistics and their practical applications. The goal is to highlight key techniques such as estimation, hypothesis testing, and regression analysis, which are essential for making data-driven decisions.
As we navigate through different sections, the relevance of these statistical methods in fields such as economics, health sciences, and engineering will become evident. Mathematical statistics transforms raw data into meaningful insights that facilitate informed decision-making.
Key Findings
Major Results
The exploration of mathematical statistics reveals several important findings:
- Estimation Methods: Techniques like point estimation and interval estimation help researchers make predictions about population parameters based on sample data. This is vital in determining the reliability of the estimates.
- Hypothesis Testing: A key aspect of statistical inference, hypothesis testing allows professionals to evaluate claims about populations. By defining null and alternative hypotheses, one can systematically decide whether to reject or accept assumptions based on sample evidence.
- Regression Analysis: This method offers insights into relationships between variables. It helps in understanding how one variable affects another, which is particularly valuable in fields like economics for modeling economic trends.
- Interdisciplinary Significance: The findings emphasize the necessity for a collaborative comprehension among various fields. The intersection of mathematical statistics with disciplines like health and engineering illustrates its broad applicability.
Discussion of Findings
The implications of these key results are profound. In health sciences, for instance, statistical methods aid in designing clinical trials and analyzing patient data for better healthcare outcomes. In economics, they assist in forecasting market trends, helping businesses and governments make strategic decisions.
Furthermore, advanced statistical techniques find relevance in engineering through quality control and reliability assessments. By utilizing statistical models, engineers can optimize processes and enhance product performance. In essence, the findings establish that mathematical statistics is not only pivotal but also transformative across diverse fields.
Methodology
Research Design
Data Collection Methods
Data collection was achieved through:
- Surveys: Gathering quantitative data from targeted participants to understand their perspectives on statistical applications.
- Case Studies: Analyzing specific instances where mathematical statistics have significantly influenced decision-making processes.
- Secondary Data Sources: Utilizing established databases and published studies, including resources on Wikipedia and Britannica, to enrich the research framework.
Understanding the practical applications of mathematical statistics is crucial for effective decision-making in today's data-driven world.
In summary, this article aims to provide a thorough examination of mathematical statistics and its wide-ranging applications, highlighting its significance in enhancing decision-making across various sectors.
Foreword to Mathematical Statistics
Mathematical statistics is a crucial discipline that offers a comprehensive framework for analyzing data and making informed decisions. Its importance extends beyond the mere collection of numerical information; it provides the tools needed to interpret that data meaningfully. This section serves as a gateway to understanding the core principles and applications of mathematical statistics, establishing a foundation for the subsequent discussions throughout the article.
Definition and Scope
Mathematical statistics can be defined as the branch of mathematics that deals with the collection, analysis, interpretation, presentation, and organization of data. Unlike descriptive statistics, which simply summarizes data, mathematical statistics employs mathematical theories to infer properties of a population based on a random sample.
The scope of this field is broad, covering various methodologies including estimation techniques, hypothesis testing, and the use of statistical models to explain phenomena. By applying mathematical principles alongside statistical theory, practitioners can derive conclusions that significantly affect fields such as economics, health, and engineering. The use of probability theory is also paramount, providing the necessary tools to quantify uncertainty and assess random variations in data.
This discipline plays an essential role in research, allowing for evidence-based conclusions that can improve decision-making processes and guide policies. As industries become increasingly data-driven, the relevance of mathematical statistics continues to expand, shaping careers in several scientific, engineering, and business contexts.
Historical Context
The roots of mathematical statistics can be traced back to ancient civilizations where counting and basic probability were first explored. However, it was in the 18th century that the field began to take shape with significant contributions from mathematicians like Pierre-Simon Laplace and Carl Friedrich Gauss.
The development of probability theory during this time laid the groundwork for subsequent advancements in statistical methodologies. The introduction of key concepts such as sampling distributions and the Central Limit Theorem further refined the discipline. By the 20th century, influential statisticians like Ronald A. Fisher formalized statistical methods and established principles of experimental design, which are still used today.
Understanding this historical context not only highlights the evolution of mathematical statistics but also its growing importance in various fields. The transition from theoretical development to practical application signifies how far the discipline has come and sets the stage for its future in the context of big data and analytics.
"Mathematical statistics enables the interpretation of data in a way that transcends the mere numbers, guiding actionable insights and informed decision-making."
In summary, the importance of mathematical statistics cannot be understated. It is a powerful framework that informs and enhances our understanding of the world, making it critical for students, researchers, educators, and professionals who seek to engage with data in a meaningful way.
Core Concepts of Mathematical Statistics
Mathematical statistics provides the essential building blocks for understanding complex data and making informed decisions based on that data. This section focuses on fundamental aspects such as probability theory and random variables, which are crucial for statistical analysis. Understanding these core concepts is vital for applying mathematical statistics to real-world scenarios, especially in fields like economics, health sciences, and engineering. These concepts enable professionals to interpret data correctly and make predictions, thus enhancing decision-making processes.
Probability Theory Fundamentals
Probability theory serves as the backbone of mathematical statistics. It allows statisticians to quantify uncertainty and model random events. Every statistical inference relies on probability to make reasonable conclusions about populations based on sample data. Knowing the laws of probability, including concepts such as independent events, conditional probability, and Bayes' theorem, is crucial. These fundamentals aid in calculating various probabilities needed for advanced statistical methods. Understanding probability distributions helps statisticians select appropriate models for different types of data, thereby enhancing the accuracy of their inferences.
Random Variables and Distributions
Random variables are a central idea in statistical analysis, allowing researchers to quantify outcomes of random phenomena. They can be classified into two main types: continuous and discrete.
Continuous Distributions
Continuous distributions represent the probabilities of a continuous outcome. One common example is the normal distribution, often found in nature and human behavior. The significance of continuous distributions lies in their applicability to measure variables that can take any value within a given range. One key characteristic is the smooth curve, known as the probability density function, which indicates the likelihood of a particular value. This feature makes it beneficial for modeling phenomena in fields ranging from finance to natural sciences.
However, continuous distributions can present some challenges. For instance, calculating probabilities involves integrating the density function over an interval, which necessitates a certain level of mathematical proficiency. Despite this, their widespread use in inferential statistics and data analysis underscores their vital role.
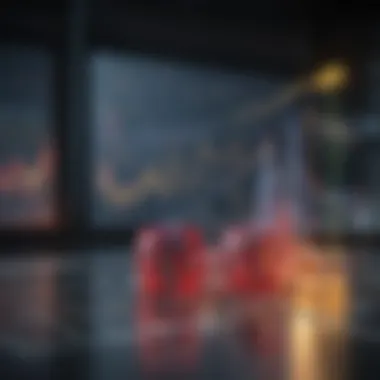
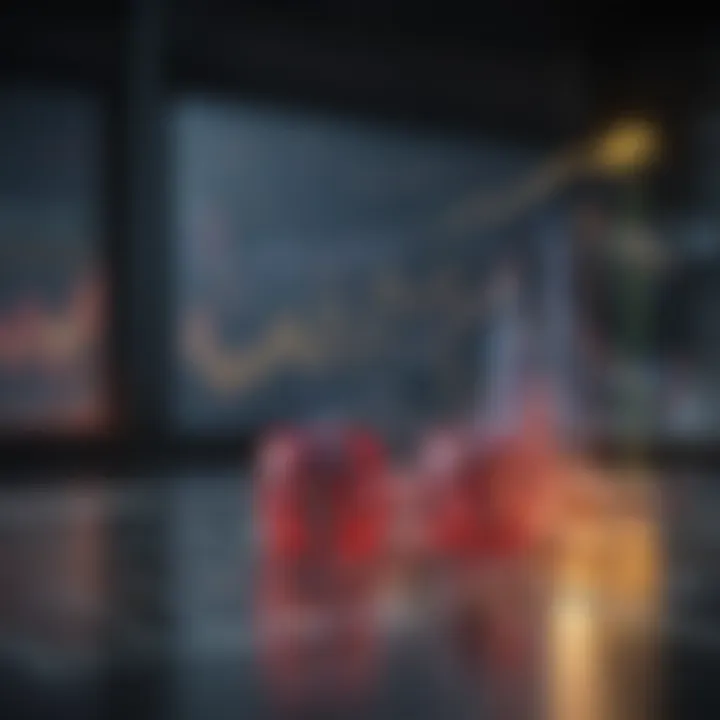
Discrete Distributions
Discrete distributions deal with countable outcomes, such as the number of successes in a series of trials. The binomial distribution is a classic example. The primary advantage of discrete distributions is that they provide a clear view of probability for each possible outcome, which is valuable in situations where events are distinct and limited. This clear framework allows for straightforward calculations of probabilities.
A unique feature of discrete distributions is that they can easily be illustrated using bar graphs, providing a quick visual interpretation. However, the limitation arises when dealing with large datasets where discrete outcomes may not be frequent enough, making interpretations less informative. Despite this, discrete distributions play a crucial role in various applications, including quality control and genetics, providing robust models when the data is inherently countable.
Estimation Techniques
Estimation techniques are crucial in mathematical statistics, serving as the foundation for making inferences about population parameters based on sample data. These approaches enable statisticians to derive conclusions and make predictive analyses with a certain degree of confidence. In many real-world applications, having reliable estimates is essential for informed decision-making.
In this section, we explore three primary estimation techniques: point estimation, interval estimation, and maximum likelihood estimation. Each of these plays a unique role in statistical analysis and comes with its own set of benefits and considerations.
Point Estimation
Point estimation refers to the process of providing a single value as an estimate of an unknown population parameter. For example, one might estimate the average height of a group of individuals based on a sample. The sample mean serves as the point estimator. This technique is advantageous because it simplifies the data to a single figure, making interpretations more straightforward.
However, reliance on point estimates can be hazardous. They do not account for variability in data or uncertainty around the estimate itself. Importantly, a point estimate could be misleading if the underlying assumptions are incorrect or if the sample size is too small to represent the population accurately. Hence, while point estimation is useful, it must be combined with other techniques to provide a fuller picture.
Interval Estimation
Interval estimation provides a range of values within which the population parameter is likely to fall, offering a more comprehensive view than point estimation. This range is defined by a confidence interval, which quantifies the uncertainty associated with the estimate. For instance, one might say, "We are 95% confident that the average height of the population lies between 170 cm and 180 cm."
This technique holds several benefits, including the ability to reflect the inherent uncertainty in estimates. Interval estimation also helps in assessing the precision of the estimate. However, it is vital to consider how the interval is constructed, including the selected confidence level. Different confidence levels yield different intervals, potentially influencing any decisions based on these estimates.
Maximum Likelihood Estimation
Maximum likelihood estimation (MLE) is a sophisticated method used to find the parameter values that maximize the likelihood of the observed data given a statistical model. Simply put, it identifies parameters that make the observed data most probable. This technique is widely used in various statistical analyses, including regression models and complex data structures.
MLEs have a few notable advantages. They often yield estimators that possess desirable properties such as consistency and asymptotic normality. However, MLE can be computed only under the assumption of the correct model specification; an incorrect model can lead to biased estimates. Therefore, ensuring the model accurately represents the underlying data is crucial for effective use of maximum likelihood estimation.
"Estimates are more valuable when they inform decisions and highlight uncertainties clearly."
In summary, understanding estimation techniques like point, interval, and maximum likelihood estimation is essential for professionals engaged in statistical analysis. Each technique carries unique strengths and challenges, underlining the importance of a well-rounded approach to data interpretation.
Hypothesis Testing
Hypothesis testing serves as a cornerstone of statistics, providing a systematic approach for making inferences about population parameters based on sample data. It enables researchers to determine whether their observations support a specific belief or theory. This aspect of mathematical statistics is particularly significant, given its role in various fields such as medicine, social sciences, and engineering. Understanding the principles of hypothesis testing is essential for validating research findings and making informed decisions.
The Concept of Null and Alternative Hypotheses
At the heart of hypothesis testing lies the concept of null and alternative hypotheses. The null hypothesis (H0) posits that there is no effect or no difference, serving as a default position in statistical testing. Meanwhile, the alternative hypothesis () represents what the researcher aims to prove or the existence of an effect.
An example illustrates this well. In a new drug study, the null hypothesis might state that the drug has no impact on patient recovery, while the alternative hypothesis would suggest that it does improve recovery rates. Researchers gather data to either reject or fail to reject the null hypothesis based on the evidence. This clear distinction between H0 and is crucial, as it guides the whole testing process and the ensuing interpretations of the results.
Type and Type Errors
Hypothesis testing involves risks of error. A Type I error occurs when the null hypothesis is incorrectly rejected, suggesting a significant effect where none exists. In our drug example, this error might lead to the false conclusion that the drug is efficacious when it is not.
Conversely, a Type II error happens when researchers fail to reject a false null hypothesis. In the context of our drug trial, this implies concluding that the drug is ineffective while it actually has a beneficial effect. Understanding these errors is crucial for evaluating the reliability of hypothesis tests and determining the appropriate alpha level, which dictates the likelihood of making a Type I error. Minimizing these errors should be a priority for any statistical analysis or research.
P-values and Significance Levels
P-values provide a metric for determining the strength of evidence against the null hypothesis. A p-value represents the probability of obtaining a test statistic at least as extreme as the one observed, assuming the null hypothesis is true. A smaller p-value indicates stronger evidence against H0.
Researchers often compare p-values to a predefined significance level (alpha), typically set at 0.05. If the p-value is less than alpha, the null hypothesis is rejected in favor of the alternative hypothesis. This threshold is fundamental for making decisions in hypothesis testing. However, it is crucial to interpret p-values carefully, as they do not measure the size of an effect or the importance of a result.
The reliability of p-values depends on sample size, study design, and the assumptions made, necessitating a thorough understanding.
In summary, hypothesis testing is an indispensable tool in mathematical statistics. It involves formulating null and alternative hypotheses, understanding potential errors, and calculating p-values to validate research findings. A firm grasp of these concepts is vital for students, researchers, educators, and professionals engaged in data analysis and interpretation.
Regression Analysis
Regression analysis plays a critical role in mathematical statistics, serving as a profound statistical tool for understanding relationships among variables. It allows researchers and practitioners to model the dependencies between a dependent variable and one or more independent variables. Through regression analysis, complex data structures can be simplified, permitting clearer conclusions about trends and behaviors inherent in datasets. This relevance is particularly pronounced in fields like economics, health sciences, and engineering, where informed decisions can significantly impact outcomes.
Simple Linear Regression
Simple linear regression is the most foundational form of regression analysis. It examines the linear relationship between two variables: a dependent variable and one independent variable. The objective is to model the dependent variable using a straight line represented by the equation:
[ y = mx + b ]
Here, (y) is the dependent variable, (x) is the independent variable, (m) is the slope of the line, and (b) is the y-intercept. The slope indicates how much (y) changes for a one-unit change in (x).
The application of simple linear regression is beneficial in scenarios such as predicting sales based on advertising expenditure or estimating the impact of study time on test scores. By providing a clear quantitative estimate, it helps stakeholders make better decisions based on the observed data trends.
Multiple Linear Regression
When the situation merits more complexity, multiple linear regression takes the stage. It extends simple linear regression by allowing multiple independent variables to affect the dependent variable. The model can be expressed as:

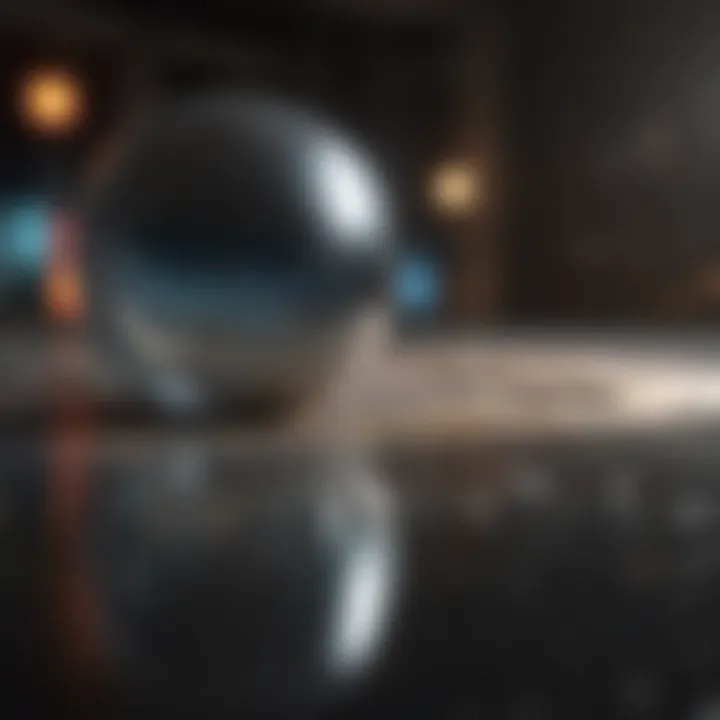
[ y = b_0 + b_1x_1 + b_2x_2 + + b_kx_k + \epsilon ]
Here, ( b_0 ) is the intercept, ( b_i ) are the coefficients for each independent variable, and ( \epsilon ) is the error term. This greater flexibility offers a more nuanced understanding of the interrelationships among the variables at play.
Multiple linear regression is widely used in various contexts, such as economic forecasting, where factors like interest rates, inflation, and consumer spending may all interact to influence the financial landscape. Understanding these relationships can yield predictive insights and formulate strategies for growth or intervention.
Model Diagnostics
Once regression models are fitted, it is crucial to assess their validity and reliability through model diagnostics. This involves checking for several key assumptions:
- Linearity: The relationship between variables should be linear.
- Independence: Residual terms should be independent of each other.
- Homoscedasticity: The residuals should exhibit constant variance at any level of the independent variable.
- Normality: The residuals should be normally distributed.
Utilizing diagnostics allows researchers to validate if the model fits well and if the assumptions hold. Methods such as residual plots, the Durbin-Watson test, and the Breusch-Pagan test can facilitate this examination. Inadequate model performance can lead to poor predictions, ultimately undermining decision-making efforts.
"Effective regression analysis is not just about fitting a line through data, but understanding the intricacies of the model and ensuring its assumptions are met."
Ultimately, regression analysis is a powerful statistical method that provides essential insights across many fields. By mastering the techniques within, one can enhance their ability to extract actionable knowledge from data.
Applications in Various Fields
Mathematical statistics plays a crucial role in diverse fields. Understanding its applications enables professionals to make informed decisions. This section explores how mathematical statistics is applied in key areas such as economics, health sciences, and engineering. Each area showcases unique elements and benefits, demonstrating the importance of statistical methods in everyday scenarios.
Applications in Economics
In economics, statistical methods help analyze data trends and patterns. Economists use these techniques to evaluate market behaviors and forecast economic conditions. For instance, regression analysis is often utilized to understand the relationship between different economic indicators. It can show how changes in interest rates impact consumer spending.
Furthermore, statistical analysis aids in policy-making. Governments rely on data to shape policies that affect economic health. By assessing data on employment rates or inflation, economists can recommend effective measures. Statistical models contribute significantly to scenario simulations, allowing economists to predict various outcomes based on different variables.
Applications in Health Sciences
Health sciences heavily depend on statistical methods for research and analysis. Clinical trials use these techniques to determine the effectiveness of treatments. Statistical tests identify whether results are significant, ensuring that findings are reliable. The use of nonparametric tests, for example, can provide insights into patient outcomes without assuming a normal distribution.
Statistical methods also play a role in epidemiology. Researchers analyze patterns of disease occurrence to develop public health strategies. By applying mathematical models, they can predict the spread of diseases and evaluate interventions. Statistical data informs decisions on resource allocation, helping health professionals respond effectively to health crises.
Applications in Engineering
In engineering, mathematical statistics is essential for quality control and reliability analysis. Engineers apply statistical methods to assess product performance and ensure standards are met. For example, process control charts monitor manufacturing processes over time, detecting variations to maintain quality.
Moreover, statistical methods help in risk assessment and management. In fields like civil engineering, probabilistic models estimate the likelihood of failures in structures. This analysis is vital for designing safe systems. Statistical software enables engineers to analyze complex data, reinforcing decision-making processes.
In summary, mathematical statistics is a powerful tool across various fields. Its applications not only enhance understanding but also contribute to better decision-making in economics, health sciences, and engineering. Understanding these applications is key for professionals seeking to leverage statistical methods for effective problem-solving.
Bayesian Statistics
Bayesian statistics represents a significant paradigm in the realm of statistical analysis. It departs from traditional frequentist methods by emphasizing the role of prior knowledge in determining probabilities. This approach is essential for understanding uncertainty in statistical inference and facilitating decision-making under uncertainty. Bayesian statistics allows practitioners to update their beliefs in light of new data, making it particularly useful in dynamic fields such as finance, biology, and technology.
Principles of Bayesian Inference
Bayesian inference revolves around Bayes' Theorem, which mathematically encapsulates the relationship between prior knowledge and new evidence. The core components consist of:
- Prior Probability: This reflects initial beliefs before observing any data. It is subjective and can be informed by previous studies or expert knowledge.
- Likelihood: This element represents the probability of observing the current data under different hypotheses or models.
- Posterior Probability: This is the updated probability after incorporating the evidence provided by the data, derived from Bayesβ Theorem as follows:
where (P(H | D)) is the posterior probability, (P(D | H)) is the likelihood, (P(H)) is the prior, and (P(D)) serves as a normalizing constant. This framework allows for the continuous revision of beliefs as more data becomes available, enabling robust statistical modeling.
Bayesian vs Frequentist Approaches
The distinction between Bayesian and frequentist statistics is pronounced and has far-reaching implications for how data analysis is conducted. Key differences include:
- Interpretation of Probability: Bayesian statistics interprets probability as a degree of belief, while frequentist statistics regards probability as a long-term frequency of events.
- Use of Prior Information: In Bayesian analysis, prior information is explicitly included and updated. In contrast, frequentist methods typically do not incorporate prior beliefs and focus solely on data observed.
- Inference Methods: Bayesian statistics often utilizes credible intervals and posterior distributions for inference, while frequentist statistics relies on confidence intervals and p-values.
Bayes' approach is increasingly favored in complex and high-dimensional problems where traditional methods may falter, especially when data is scarce or noisy.
Ultimately, understanding both Bayesian and frequentist approaches enriches the interpretation of statistical evidence and enhances analytical capabilities across various fields. Bayesian methods, in particular, are becoming more prevalent in areas that demand adaptability to new evidence and a nuanced understanding of uncertainty.
Nonparametric Statistics
Nonparametric statistics plays a vital role in statistical analysis, especially when the assumptions of traditional parametric methods do not hold. It is prevalent in various fields and provides flexible approaches for data analysis. Nonparametric methods are often preferred in cases where the sample size is small or the data do not meet the criteria required for parametric tests. This gives researchers and statisticians powerful tools to make valid inferences without the limitations imposed by strict assumptions.
Intro and Applications
Nonparametric methods are based on fewer assumptions about data distribution. They apply to nominal and ordinal data, making them versatile for various applications. One significant aspect of nonparametric statistics is its robustness; it remains effective even when data deviate from the normal distribution. Examples include the Wilcoxon rank-sum test, which is useful for comparing two independent samples when the assumption of normality is not met.
In health sciences, nonparametric tests assist in clinical trials where small sample sizes are common. In social sciences, researchers utilize these methods to analyze survey data, especially when responses are categorical. Nonparametric techniques also find application in manufacturing industries, providing reliable processes for quality control and reliability testing. Hence, they offer practical solutions across differing fields without the constraints of parametric models.
Common Nonparametric Tests
The landscape of nonparametric statistics is diverse, offering a wide range of tests suited for different data types and research questions. Some of the most common tests include:

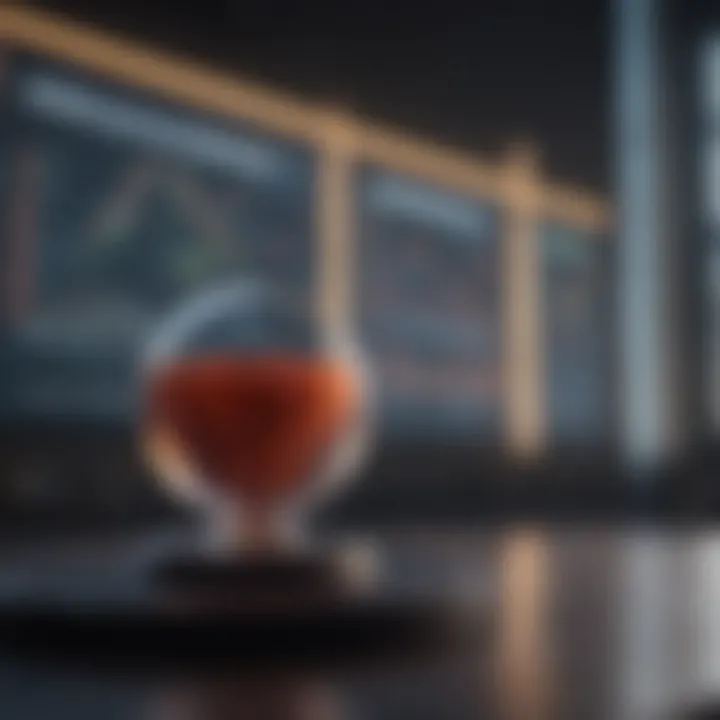
- Mann-Whitney U Test: This test assesses whether two independent samples come from the same distribution. It is particularly useful when dealing with ordinal data.
- Wilcoxon Signed-Rank Test: Used for paired samples, this test checks whether their ranks differ, making it an ideal choice for pre-test and post-test scenarios.
- Kruskal-Wallis H Test: A nonparametric alternative to ANOVA, it evaluates whether three or more independent samples originate from the same distribution.
- Friedman Test: This test determines whether there are differences in treatments across multiple test attempts, notably when dealing with repeated measures.
These tests provide significant advantages. They do not require a large sample size or a specific distribution shape. Thus, researchers can obtain valuable insights from data that traditional methods might discard. Despite their advantages, nonparametric tests can be less powerful than parametric tests when the assumptions of the latter are satisfied. Therefore, it is essential to choose the appropriate statistical method based on the data characteristics available.
Nonparametric tests are a crucial toolkit for statisticians, enabling analysis without strict distributional assumptions.
Statistical Software and Tools
Statistical software and tools are crucial in enhancing the efficiency of data analysis within mathematical statistics. With the growing amount of data across various fields, proper software solutions assist statisticians and researchers in deriving meaningful insights. These tools not only facilitate data manipulation and statistical analysis but also enable visual representation of data findings, which can be vital for effective communication.
Overview of Statistical Software
Various statistical software packages are available that cater to different needs. Some of the most popular ones include SPSS, R, Python with libraries like Pandas and NumPy, and SAS. Each of these tools comes with unique features.
- SPSS: Known for its user-friendly interface, it is widely used in social sciences for data analysis and manipulation.
- R: This is an open-source tool highly favored in academia for its extensive range of statistical techniques and packages. R allows for advanced analyses that can be tailored as per user requirements.
- Python: As a versatile programming language, Python, equipped with libraries such as SciPy and Matplotlib, provides robust support for modeling and visualization. Its grip on data science is growing.
- SAS: This software is extensively used in various industries, especially for advanced analytics, multivariate analysis, and business intelligence.
Each of these tools offers distinct advantages based on the complexity of analysis and ease of use, impacting the choice of software in different scenarios.
Data Visualization Techniques
Visualizing data is as important as analytical processing. Good visual representation helps to uncover patterns, trends, and outliers in data. Common techniques include:
- Graphs: Bar charts, line graphs, and scatter plots are essential for representing data trends and relationships visually.
- Heat Maps: These are particularly useful for presenting complex data in a more digestible format, ideally suited for large datasets.
- Box Plots: Useful in displaying the distribution of data points, presenting summary statistics at a glance.
Data visualization enhances comprehension and can lead to better insights from statistical analyses.
When utilizing data visualization software, it is essential to focus on clarity and relevance, ensuring that the visualizations effectively communicate the intended message. Tools like Tableau and Microsoft Power BI are excellent for creating compelling visual stories from data.
In summary, statistical software and tools play a vital role in modern statistical analysis. They not only improve the functionality and efficiency of statistical methods but also enable professionals and researchers to visualize complex data effectively.
Future Trends in Mathematical Statistics
In the current era, mathematical statistics is undergoing significant evolution, shaped by technological advancements and the increasing complexity of data. Understanding the future trends in this discipline becomes essential for students, researchers, educators, and professionals. It not only prepares them for the challenges ahead but also equips them with the tools to harness emerging technologies. The integration of big data and analytics, along with machine learning, is reshaping the landscape of data analysis and statistical inference. This section will explore these elements and the profound implications for statistical applications.
Big Data and Analytics
Big data refers to the vast volumes of structured and unstructured data produced from various sources. This includes social media, sensors, transactions, and more. The ability to analyze such massive datasets presents both opportunities and challenges in statistical methodology.
Benefits of big data in mathematical statistics include:
- Enhanced decision-making capabilities through data-driven insights.
- Improved predictive accuracy in various models.
However, there are considerations to keep in mind. Data quality can be inconsistent, leading to potential biases in analysis. Hence, statisticians must develop methods that ensure the integrity and validity of results drawn from big data. The versatility of big data analytics tools like Apache Hadoop and Spark allows statisticians to process and analyze large datasets more efficiently, an important development in statistical practice.
Moreover, the fusion of big data with traditional statistical techniques results in hybrid approaches, strengthening the reliability of interpretations and conclusions drawn. > "The rise of big data transforms how statisticians approach problems, facilitating deeper insights from previously unattainable datasets."
Machine Learning and Statistical Models
Machine learning has gained traction largely due to its robustness in handling complex data patterns that are challenging for traditional statistical methods. This intersection between machine learning and statistical models heralds a significant advancement in data analysis techniques.
Key aspects to consider in this area include:
- The utilization of algorithms that can learn from data, allowing for dynamic and responsive model development.
- The emphasis on predictive modeling, which improves with the incorporation of machine learning techniques, enhancing forecasts in various applied fields such as finance and healthcare.
Statistical models benefit from machine learning's capacity to adapt. For instance, regression techniques can be strengthened through the integration of algorithms that identify non-linear relationships, leading to more accurate estimations.
Education and training programs are now increasingly emphasizing the importance of machine learning in statistics. This prepares individuals to not only adapt to changing environments but also to lead the development of innovative statistical methodologies.
Closure
The conclusion of an article on mathematical statistics is crucial for synthesizing the information presented throughout. It highlights the primary insights gained from discussing estimation techniques, hypothesis testing, regression analysis, and the various applications across different fields. The main point is to reinforce the significance of statistical methods in making informed decisions and formulating effective policies.
In this article, we explored core concepts and their practical implications. We discovered how mathematical statistics serves as a backbone for data-driven analysis, facilitating clear interpretations and insightful predictions. These elements contribute directly to improved methodologies in disciplines such as economics, health, and engineering, which can lead to advancements in those areas.
The role of statistical methods extends beyond mere analysis. By utilizing these techniques, professionals can better understand trends, make accurate forecasts, and assess the impact of various factors on their fields. This nuanced comprehension allows for better strategies tailored to specific challenges.
"Statistical thinking will one day be as necessary for efficient citizenship as the ability to read and write" - H.G. Wells.
Ultimately, the conclusion serves to remind the reader of the essential role mathematical statistics plays. It also emphasizes the need for interdisciplinary approaches, ensuring that diverse perspectives contribute to the ongoing evolution of statistical practices.
Summary of Key Points
- Mathematical Statistics Importance: Vital for data interpretation and decision-making across various fields.
- Core Concepts: Included estimation techniques, hypothesis testing, and regression analysis.
- Practical Applications: Highlighted applications in economics, health sciences, and engineering.
- Interdisciplinary Necessity: Underlined the importance of combining insights from different fields to enhance statistical understanding.
Implications for Future Research
The insights gained from this article suggest several areas for future research. First, the intersection of mathematical statistics with emerging technologies, like big data analytics and machine learning, remains a key focus area. Future studies can explore the integration of these fields to enhance predictive accuracy and efficiency in analyses.
Moreover, as data becomes increasingly complex, developing more robust and flexible statistical methods will be essential. This includes refining current techniques and possibly creating new models tailored for intricate datasets.
Finally, further investigation into the ethical implications of statistical practices in data collection and interpretation will be vital. Understanding biases and ensuring transparency in statistical methodologies could promote greater trust in data-driven outcomes.
By addressing these elements, researchers can not only advance the field of mathematical statistics but also contribute to its adaptation in an evolving data landscape.