Understanding Multivariate Test Statistics in Research
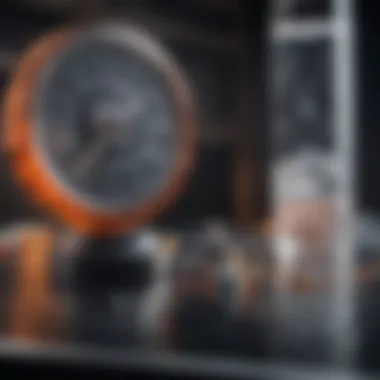
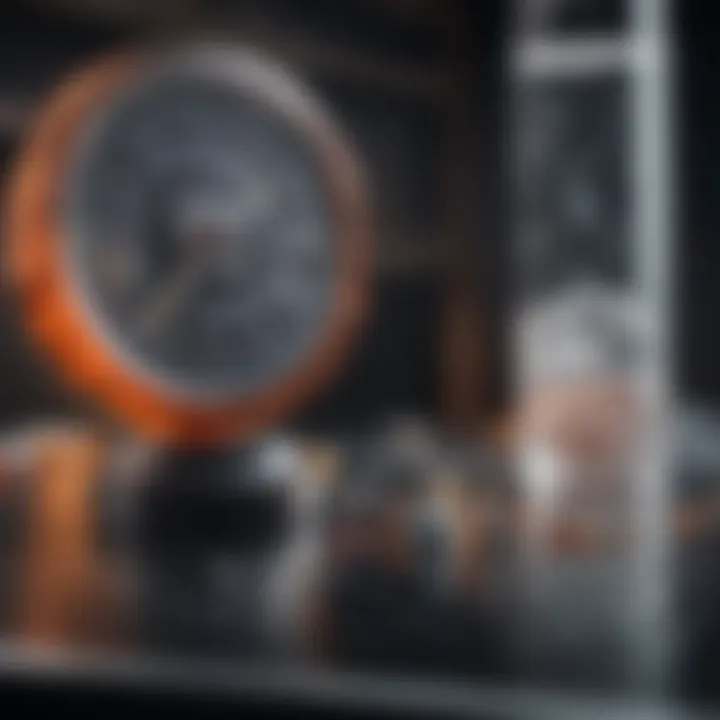
Intro
Multivariate test statistics provide essential tools for researchers, allowing them to analyze multiple variables simultaneously. This can be particularly vital in fields where complex interactions exist. Understanding these statistics is crucial for drawing valid conclusions and informing future research decisions. As methodologies evolve and expand, so too does the need for a solid grasp of their foundational principles and applications.
The significance of multivariate analysis cannot be understated, as it aids in uncovering patterns and relationships that may not be evident when examining a single variable. As researchers explore numerous variables, they must be cautious about the interpretations and methodologies employed to ensure accurate results. This article delves deep into these considerations, aiming to equip researchers and students with a comprehensive understanding of multivariate test statistics.
Key Findings
Major Results
Multivariate test statistics reveal critical insights in various research applications. They enable the simultaneous analysis of multiple variables, thus providing a richer context for data interpretation. One key finding is how these methods can enhance understanding across disciplines such as psychology, economics, and biology.
The ability to identify interactions between variables leads to more accurate models of real-world phenomena. For instance, in psychological research, evaluating how different traits interact can yield insights into complex behaviors. In economic studies, researchers often observe interdependencies between various economic indicators. The main results underscore the importance of adopting a multivariate approach in studies to avoid simplistic conclusions.
Discussion of Findings
Despite their benefits, multivariate test statistics come with challenges. Researchers must be aware of potential pitfalls like multicollinearity or overfitting. Understanding these issues is vital for maintaining the integrity of analyses. A primary discussion point focuses on the need for careful selection of variables to include in analyses, as irrelevant variables can distort results.
"Using multivariate statistics enables researchers to grasp the complexities of their data, yet it requires caution and discernment in methodology."
Moreover, the interpretation of results can sometimes be complicated. Researchers must navigate the findings with a knowledgeable perspective, understanding that correlation does not imply causation. Clear communication of results also becomes imperative to ensure that findings are effectively conveyed to stakeholders.
Methodology
Research Design
A prominent aspect of utilizing multivariate statistics is establishing a robust research design. This involves a clear definition of research objectives and hypotheses, which direct the choice of statistical tests. Depending on the research question, various multivariate statistical methods like MANOVA, canonical correlation, or factor analysis may be employed.
Data Collection Methods
The methods of data collection significantly influence the outcomes of multivariate analyses. Researchers can choose from surveys, experiments, observational studies, or existing databases. Ensuring that data is collected under carefully controlled conditions helps reduce biases and enhances the reliability of results. Tools like SPSS, R, or Python can aid researchers in performing these complex analyses efficiently.
Preface to Multivariate Test Statistics
Multivariate test statistics play a crucial role in research across various disciplines. Understanding this topic allows researchers to analyze multiple variables simultaneously, which provides deeper insights into complex datasets. Traditional univariate analysis only considers one variable at a time, often simplifying the complexities inherent in real-world data. In contrast, multivariate analysis captures the interplay between variables, which can reveal patterns and relationships that might go unnoticed in simpler analyses.
Definition and Overview
Multivariate test statistics encompass a range of statistical techniques designed to assess the relationships among three or more variables. These techniques allow researchers to determine whether the means of different groups are statistically different from each other when multiple outcomes are involved. For example, Hotelling's T-squared test and MANOVA are common approaches.
The term 'multivariate' refers to multiple variables or outcomes, and this is critical for researchers today, as many studies must consider various interrelated factors.
Using multivariate test statistics, a researcher can assess how various factors influence outcomes in fields such as health, marketing, and social sciences. This approach helps not only in hypothesis testing but also in dimension reduction, making it invaluable when dealing with high-dimensional data.
Importance in Research
The importance of multivariate test statistics cannot be overstated. The ability to analyze several variables together provides a more holistic understanding of the underlying phenomena. Here are several key points about their significance:
- Comprehensive Analysis: Multivariate techniques enable a multifaceted approach to data analysis, which is essential for capturing the complexity of real-world situations.
- Enhanced Predictive Power: By considering interactions between variables, researchers can improve predictive models. This advancement is crucial for fields that rely on forecasts, such as economics and healthcare.
- Reduction of Type I Errors: Multiple comparisons increase the risk of Type I errors, which occur when a statistically significant result is found by chance. Multivariate tests control for this risk by considering all tests at once, enhancing the validity of the findings.
- Better Resource Allocation: In applied research, understanding the relationship among various factors allows for more efficient allocation of resources, whether in clinical trials or marketing strategies.
In summary, mastery of multivariate test statistics equips researchers with tools to conduct sophisticated analysis, leading to more nuanced insights and decisions in their respective fields. Multivariate statistics provide a foundation for understanding procedural dynamics that single-variable analyses could easily overlook.
"Multivariate statistics are not just advanced tools; they are essential for navigating the complexity of modern research challenges."
By delving into the fundamentals of multivariate test statistics, researchers and students can better grasp how to apply these techniques and interpret their results effectively.
Theoretical Foundations
The concept of theoretical foundations in multivariate test statistics serves as a cornerstone for understanding advanced data analysis. It encompasses key statistical concepts and various forms of analysis, which are fundamental for researchers who wish to leverage complex datasets effectively. A solid grasp of these foundations enables researchers to choose appropriate methods for answering specific research questions and ensures the integrity of their conclusions. Understanding theoretical frameworks also aids in addressing the challenges faced when interpreting results. In essence, these foundations form the bedrock of sound research practices in any discipline that relies on statistical analysis.
Statistical Concepts
Hypothesis Testing
Hypothesis testing is a fundamental statistical process that evaluates assumptions about a dataset. It involves setting up a null hypothesis, which typically represents no effect or relationship, against an alternative hypothesis. The contribution of hypothesis testing to multivariate analysis is significant, as it provides a structured approach to determining whether observed effects are statistically significant. One key characteristic of hypothesis testing is its reliance on predetermined significance levels, often set at 0.05. This threshold allows researchers to make informed decisions based on the probability of falsely rejecting the null hypothesis.
The unique feature of hypothesis testing in multivariate contexts lies in its applicability to multiple dependent variables simultaneously. However, it has its own set of challenges. For instance, the interpretation of p-values can sometimes lead to misunderstandings about the strength and relevancy of the findings.
P-Values
P-values are essential in hypothesis testing, representing the probability of observing results at least as extreme as those in the sample, assuming that the null hypothesis is true. They provide insight into the strength of the evidence against the null hypothesis. A key characteristic of p-values is their direct relationship to statistical significance. P-values reinforce the credibility of findings, making them a popular choice in research reporting.
However, p-values have notable limitations. For instance, they do not measure the size of an effect or its practical significance. This can result in misleading conclusions when researchers focus solely on achieving p-values below the threshold without considering broader implications. It is crucial to supplement p-value analysis with additional statistical measures for comprehensive insights.
Confidence Intervals
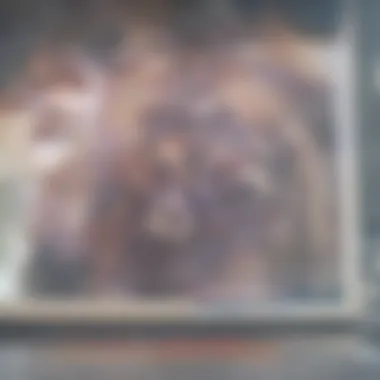
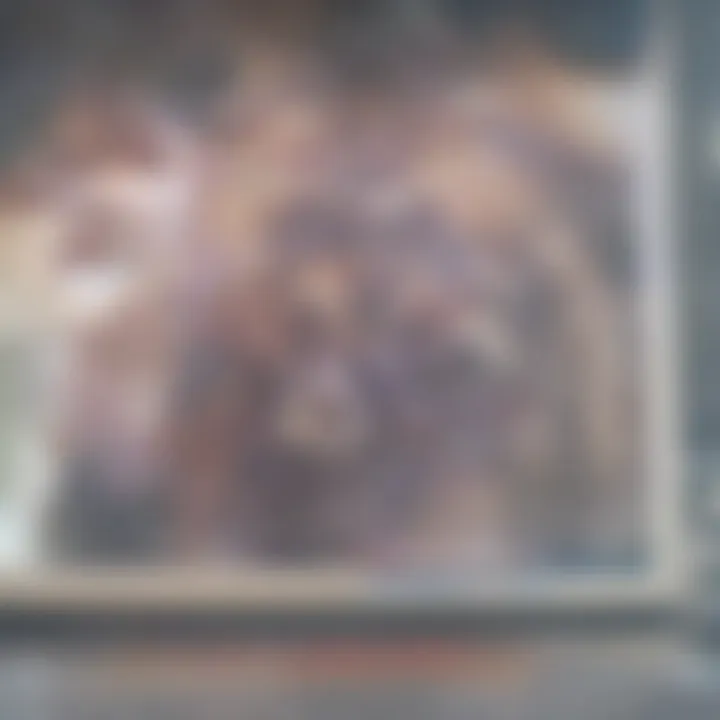
Confidence intervals provide a range of values that likely contain the true population parameter, offering valuable insight into the precision of an estimate. They contribute to multivariate test statistics by presenting a broader context around point estimates derived from sample data. A key characteristic of confidence intervals is their ability to indicate the level of uncertainty associated with an estimate. Researchers typically use a 95% confidence level, suggesting that if the same sampling process were repeated multiple times, approximately 95% of the generated intervals would capture the true parameter.
The unique feature of confidence intervals is their intuitive interpretation as they convey information about variability and potential error. While beneficial for conveying the reliability of estimates, they can also pose challenges. For example, confidence intervals do not account for biases in sample selection, potentially leading to incorrect assumptions about generalizability.
Types of Multivariate Analysis
MANOVA
Multivariate Analysis of Variance (MANOVA) is a technique used when researchers want to examine the impact of independent variables on multiple dependent variables simultaneously. This technique is essential in studies where outcomes are interconnected. One of the key characteristics of MANOVA is its ability to determine if the mean differences among groups on a combination of dependent variables are statistically significant. This makes it a preferred choice in various fields, including psychology and health sciences.
The unique feature of MANOVA is the capability to assess the effect of independent variables while controlling for others. Nevertheless, it requires certain assumptions, such as normality and homogeneity of variances, that if unmet can lead to inaccurate results.
Principal Component Analysis
Principal Component Analysis (PCA) is a statistical method used for reducing the dimensionality of data while preserving as much variance as possible. It helps in identifying underlying patterns in complex datasets. A crucial aspect of PCA is its ability to summarize large datasets by transforming them into principal components that account for variance. This method is popular for exploratory data analysis, allowing researchers to visualize complex relationships.
PCA's unique feature lies in its effectiveness at detecting multicollinearity in datasets, making it helpful for subsequent analyses. However, it is essential to note that PCA does not provide a solution for causality; rather, it illustrates associations.
Factor Analysis
Factor analysis is used to identify underlying relationships between observed variables. This analysis assists researchers in uncovering latent variables that may not be immediately apparent. A key characteristic of factor analysis is its focus on data reduction and interpretation, allowing researchers to simplify data structures.
The unique feature of factor analysis is its dual purpose in both exploratory and confirmatory contexts. It can reveal complexities in data but requires thorough understanding and correct application of its assumptions. Misuse of factor analysis can lead to erroneous interpretations and flawed conclusions.
Common Multivariate Test Statistics
Common multivariate test statistics form the backbone of analyzing complex datasets that involve multiple variables. The essence of this analysis lies in assessing how these different variables interact and influence one another. By employing these statistical tests, researchers can not only understand relationships among variables but also uncover underlying patterns in data that could otherwise remain hidden.
Multivariate test statistics play a crucial role in diverse fields such as health sciences, social sciences, and marketing research. Their importance stems from the ability to evaluate intricate relationships in datasets, which are often multidimensional. This helps in maintaining rigor in research while also bolstering the validity of results obtained. Properly interpreting these statistical outputs leads to insight that can drive further inquiry or improve decision-making.
Hotelling's T-Squared Test
Hotelling's T-squared test is a multivariate extension of the Student's t-test. It evaluates whether the means of multiple groups are different from each other across several variables. This test is particularly useful in scenarios where there are more than one response measurement for each observation.
For instance, when analyzing the effects of a treatment on multiple health indicators simultaneously, Hotelling's T-squared can efficiently test the hypothesis that the means of these indicators differ by treatment type. This simplification is invaluable in studies that collect multidimensional data, allowing researchers to derive conclusions without the need for univariate testing, which could inflate type I error rates.
Wilks' Lambda
Wilks' Lambda is another essential statistic used primarily in the context of multivariate analysis of variance (MANOVA). It measures the ratio of the error variance to the total variance, essentially providing a test for whether groups differ significantly. A smaller value of Wilks' Lambda indicates that the groups being compared are likely to be different across the measured variables.
This statistic is advantageous as it takes into account the correlation between dependent variables, making it important for evaluating the overall effect of a treatment or intervention across several dimensions. Wilks' Lambda helps in deriving insights from various fields like psychology and business, where multiple variables often necessitate a collective analysis for informed conclusions.
Bartlett's Test
Bartlett's Test is primarily concerned with testing the assumption of homogeneity of variances across different groups. This test is fundamental for many multivariate techniques, such as MANOVA and regression analysis.
Homogeneity of variance assumes that the populations from which samples are drawn have similar variances. Violating this assumption can lead to invalid conclusions. Bartlett's test provides a quick method to determine if the data meets this assumption, thus allowing researchers to proceed with confidence that their analytical methods are suitably applied.
In summary, understanding these common multivariate test statistics is vital for any researcher looking to explore relationships in complex datasets. They not only enhance the validity of findings but also offer a lens through which detailed and nuanced insights can be derived from statistical analyses.
"Multivariate test statistics allow researchers to unlock the potential of data and reveal hidden patterns."
By employing tests like Hotelling's T-squared, Wilks' Lambda, and Bartlett's Test, researchers ensure they can make informed decisions based on robust statistical approaches.
Methodologies in Multivariate Testing
Understanding the methodologies in multivariate testing is essential for conducting rigorous and valid research. These methodologies encompass various processes crucial for gathering, managing, and analyzing data. They ensure that the research is objective and can produce valuable, actionable insights. Employing sound methodologies can significantly affect the overall quality and reliability of the findings. Key elements in this realm include data collection techniques, data preparation and cleaning, and selecting the right statistical test.
Data Collection Techniques
Data collection is the first step in any research process and is particularly crucial for multivariate testing. Different approaches can be employed, depending on the research design and objectives.
- Surveys and Questionnaires: One of the most common methods. Researchers can gather data from a large population within a short time.
- Experiments: This method involves manipulating variables to observe outcomes. It can provide valuable insights into causal relationships among variables.
- Observational Studies: These allow researchers to collect data without manipulation. It can lead to more naturalistic findings, although it may introduce bias.
- Secondary Data Analysis: Researchers can utilize existing datasets for their analysis. This method saves time and resources but requires careful evaluation of the data quality.
A critical aspect of choosing the right technique involves understanding the trade-offs between cost, time, and the level of control over data collection. Each technique comes with its considerations on validity, reliability, and ethical implications.
Data Preparation and Cleaning
Once data is collected, it undergoes preparation and cleaning. This process ensures that the data is suitable for analysis, minimizing potential errors.
- Handling Missing Data: Researchers must decide how to deal with missing values. Common strategies include imputation or exclusion, depending on the situation.
- Data Transformation: Sometimes, raw data needs to be transformed. This could include normalization or standardization to make variables comparable.
- Outlier Detection: Identifying and managing outliers is important. They can skew results, leading to misleading conclusions. Appropriate methods include visualization techniques or statistical tests.
Data preparation is time-consuming but is a vital step that can significantly influence the analysis's accuracy and credibility. Researchers must remain thorough and systematic during this phase.
Selecting the Right Statistical Test

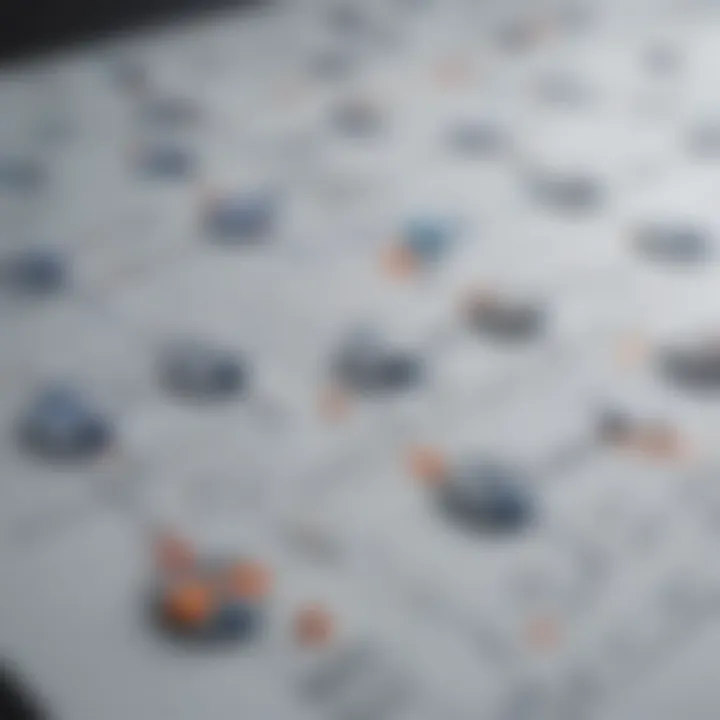
Choosing the appropriate statistical test is crucial in multivariate analysis. It directly impacts the conclusions drawn from the data. Factors to consider include:
- Data Characteristics: Different tests require specific data types (e.g., continuous, categorical).
- Assumptions of the Test: Each statistical test comes with its assumptions, such as normality or homogeneity of variance. Assessing these assumptions is vital for robust results.
- Research Questions: The nature of the research question often dictates the type of statistical test. For instance, if comparing multiple groups, MANOVA might be appropriate.
In summary, selecting the right statistical test largely revolves around understanding the data and research objectives. Incorrect choices can lead to invalid findings, underlining the importance of this step in the research process.
Applications of Multivariate Test Statistics
Multivariate test statistics play a vital role in research across various disciplines. Understanding these applications enhances not only the rigor of research design but also the reliability of conclusions drawn from complex datasets. As the volume of data increases, particularly in fields like health sciences, social sciences, and marketing research, the need for robust analytical methods becomes imperative. Multivariate test statistics facilitate the analysis of multiple dependent variables simultaneously, providing a richer understanding of relationships within the data. This section will elucidate the significance of these applications, highlighting benefits, considerations, and potential implications.
In Health Sciences
In health sciences, multivariate test statistics are essential in designing studies that involve multiple outcomes. For instance, when investigating the efficacy of a new drug, researchers may want to assess various health metrics simultaneously, such as blood pressure, cholesterol levels, and weight. By employing techniques like MANOVA, researchers can evaluate the drug's overall effect on all these variables at once, rather than limiting the analysis to single outcomes. This method not only increases statistical power but also helps control for Type I error rates associated with multiple comparisons.
Moreover, applying multivariate statistics allows researchers to control for confounding variables. For example, age, gender, and pre-existing health conditions can significantly impact patient outcomes. By accounting for these factors, researchers can isolate the effect of the primary treatment being studied. This leads to more accurate and generalizable findings, ultimately improving healthcare interventions.
In Social Sciences
The social sciences often deal with complex human behaviors that cannot be understood through univariate analyses alone. Multivariate test statistics help in capturing the intricacies of social phenomena. For instance, studies examining the relationship between socio-economic status, education level, and mental health outcomes benefit immensely from multivariate approaches.
Using techniques such as Factor Analysis, researchers can identify underlying factors that explain the patterns within the data. This can shed light on the influences of multiple variables on a single outcome, providing comprehensive insights into social issues. Moreover, Wilks' Lambda can be utilized in social science research to test hypotheses about group differences in multidimensional space. By applying these tests, researchers can derive actionable insights and contribute to social policy development.
In Marketing Research
In the realm of marketing research, understanding consumer behavior is crucial. Marketers need to analyze various factors, including demographics, purchasing behaviors, and brand perceptions, to create effective strategies. Multivariate test statistics enable this by allowing marketers to explore complex relationships between consumer attributes and their purchasing decisions.
For instance, Principal Component Analysis can be employed to reduce data dimensionality, simplifying insights while retaining the essential information needed for decision-making. Further, techniques like Hotelling's T-Squared Test can assess whether different consumer segments respond differently to marketing campaigns. By leveraging multivariate statistics, companies can optimize their approaches and target specific consumer groups more effectively.
"The integration of multivariate test statistics into research methodologies enhances not only the depth of analysis but also the applicability of findings across various fields."
In summary, the applications of multivariate test statistics in health sciences, social sciences, and marketing research underscore their importance. These methodologies allow for a nuanced understanding of complex data, leading to better decision-making and more impactful research findings.
Interpreting Multivariate Test Results
Interpreting the results of multivariate tests is crucial for extracting meaningful insights from complex data sets. This process goes beyond mere calculations; it involves contextualizing outcomes within the framework of the research questions posed. An understanding of how to evaluate results ensures researchers can draw valid conclusions and make informed decisions.
One specific benefit of mastering this skill is its application across various fields such as health sciences, social sciences, and marketing research. Each field requires a tailored approach to interpret the multivariate results correctly, taking into account the unique pressures and expectations in these environments. Assessing the relevance of findings can also guide future research directions.
In addition, considerations related to the statistical outputs can reveal the effectiveness of the methodologies employed in the study. This understanding helps in identifying potential shortcomings or strengths in the research design and its execution.
Understanding Key Outputs
Key outputs from multivariate tests often include statistical values such as F-values, p-values, and effect sizes.
- F-values indicate whether the variances across groups are significantly different. A higher F-value suggests that at least one group mean is different from others, raising further questions about specific group pair comparisons.
- P-values help determine the significance of the results. A p-value less than the chosen alpha level (commonly 0.05) indicates that findings are statistically significant; however, this does not equate to practical significance.
- Effect sizes quantifies the magnitude of difference, providing deeper insights beyond p-values alone. In practical terms, understanding effect sizes can inform researchers about the relevance of their findings in real-world applications.
When interpreting these outputs, it’s essential to consider the overall research context, as statistical significance might not imply meaningful real-world impact. Misinterpretation can lead researchers to make erroneous claims about their findings, undermining the study’s credibility.
Assessing Model Adequacy
Assessing model adequacy is a critical step following the interpretation of results. Researchers must determine whether the chosen model appropriately fits the observed data. This ensures confidence in the conclusions drawn from statistical tests.
Several techniques can assist in this evaluation:
- Residual Analysis: Examining residuals helps check whether the assumptions of the model have been met. Ideally, residuals should be randomly scattered with no apparent patterns.
- Goodness-of-Fit Tests: These tests provide quantitative measures indicating how well the model predicts the data. A model with good fit results will follow the actual data closely.
- Model Comparison: Sometimes, comparing multiple models can provide insights into which model best explains the data. AIC and BIC are common criteria used for this purpose.
By focusing on these assessments, researchers mitigate the risk of drawing invalid conclusions from baseless or misfitted models. The exploration of model adequacy promotes rigor and enhances the reliability of statistical analyses.
"The strength of your results is only as good as the model interpreting those results."
Ultimately, the process of interpreting multivariate test results is complex but essential. Mastery in this area empowers researchers, ensuring that their conclusions reflect true data insights while considering broader implications across their fields.
Challenges and Limitations
Challenges and limitations in multivariate test statistics are significant components that researchers must consider. Addressing these factors enhances the reliability and validity of their analyses. Not acknowledging these issues can lead to flawed conclusions, affecting the overall research quality. By exploring these challenges, researchers can develop strategies to mitigate their impact, ultimately strengthening the integrity of their findings.
Multicollinearity Issues
Multicollinearity occurs when independent variables in a regression model are highly correlated. This condition can distort the statistical results, making it difficult to discern the individual effect of each variable. When multicollinearity is present, coefficient estimates can become unstable and misleading. Researchers may face inflated standard errors, which in turn affect hypothesis testing. Thus, it is essential to check for multicollinearity before proceeding with the analysis.
Common methods to detect multicollinearity include:
- Variance Inflation Factor (VIF): A VIF above 10 is typically considered indicative of multicollinearity.
- Correlation Matrix: High correlation coefficients between independent variables suggest potential issues.
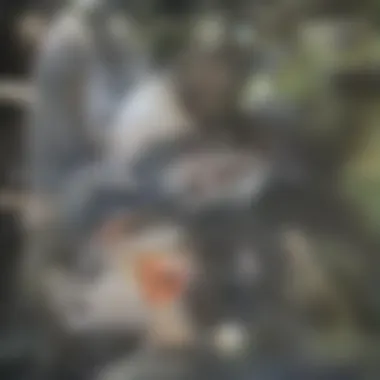
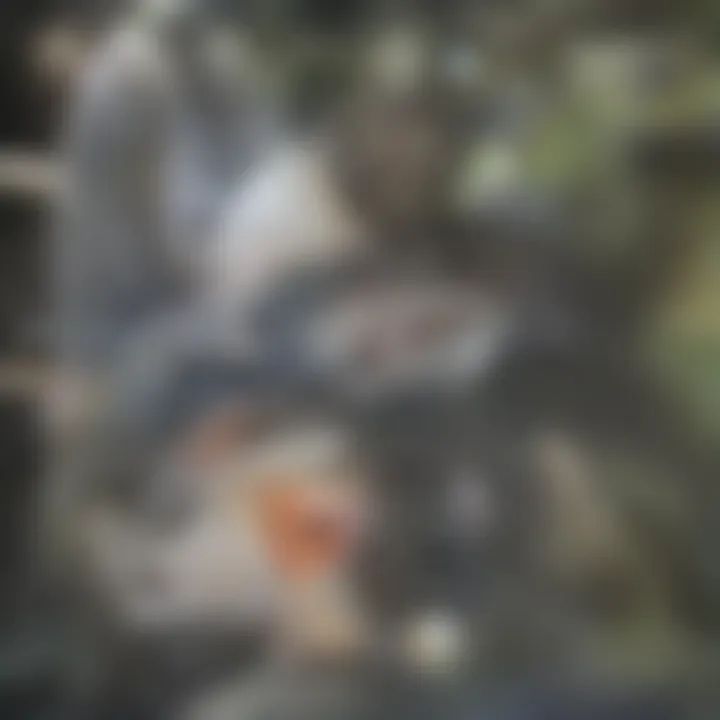
If multicollinearity is detected, researchers may consider techniques like combining correlated variables, using ridge regression, or eliminating one of the correlated variables.
Sample Size Considerations
Sample size plays a crucial role in the effectiveness of multivariate testing. Insufficient sample sizes can yield unreliable results and limit the generalizability of findings. Conversely, an excessively large sample size may fortify statistical significance without practical relevance. Thus, determining the appropriate sample size is vital.
Key factors influencing sample size include the:
- Desired power level: The probability of correctly rejecting a null hypothesis.
- Effect size: The magnitude of the relationship being examined.
- Number of variables: More variables may require larger samples for reliable estimates.
Researchers often use power analysis to calculate the needed sample size prior to data collection. This can prevent both underpowered and overpowered studies, ensuring credible findings.
Misinterpretation of Results
Misinterpretation of results in multivariate testing can occur due to various reasons including misunderstanding statistical measures or over-reliance on significance values. For instance, many researchers mistakenly equate statistical significance with practical significance. A statistically significant result does not always indicate that the effect is large enough to matter in practical terms.
Common pitfalls include:
- Ignoring effect sizes: Effect sizes provide a better understanding of the practical significance of findings.
- Overgeneralizing results: Researchers might incorrectly apply their findings beyond their study sample or context.
To mitigate misinterpretation, thorough training in statistical methods is essential. Additionally, clear reporting and discussion of results help clarify findings, guiding proper interpretation among readers.
Addressing challenges and limitations in multivariate test statistics is not merely an academic exercise. It is a practical necessity for enhancing research quality and ensuring effective communication of findings.
Best Practices for Implementation
Implementing multivariate test statistics effectively in research is crucial to yield reliable and valid results. Adopting best practices ensures that the findings have substantial value and can be trusted by peers in the academic community. This section focuses on important aspects to consider when implementing multivariate statistics.
Conducting Pilot Studies
Pilot studies are essential prior to launching comprehensive research analysis. They serve as preliminary tests for methodology, allowing researchers to evaluate the feasibility and refine data collection methods. By conducting a pilot study:
- Researchers can identify any unforeseen challenges in data collection.
- The appropriateness of chosen statistical tests can be verified.
- Researchers can get an initial sense of variances and covariances.
Pilot studies help ensure that larger-scale analyses are built on solid foundations. This exploratory phase can reveal whether adjustments in design or method are necessary before full implementation.
Reporting Standards
Adhering to reporting standards is another vital component in effectively implementing multivariate test statistics. Clear and transparent reporting improves the reproducibility of studies. Essential elements to consider include:
- Comprehensive Data Description: Clearly outline datasets, including variable types, sample sizes, and any preprocessing steps taken. This enables others to interpret and replicate your work accurately.
- Result Interpretation: Provide sufficient context for the statistical outputs. Discuss significance levels and the implications of findings on the larger field of study.
- Use of Established Guidelines: Follow recognized guidelines, like those from the American Psychological Association or CONSORT for clinical trials. Doing so enhances the credibility of research.
Adherence to reporting standards allows for better scrutiny of methods and findings, leading to more robust scientific discourse.
Future Trends in Multivariate Statistics
The landscape of multivariate statistics is continually evolving, influenced by advancements in technology and shifting research paradigms. Understanding future trends in this discipline is crucial for researchers and practitioners who aim to stay at the forefront of analytical techniques. These trends not only signify the growing incorporation of novel methodologies but also highlight the necessity for researchers to adapt to an increasingly complex data environment. Embracing these trends enhances the robustness and applicability of multivariate analyses, ensuring that they meet current demands in research.
Integration of Machine Learning Techniques
One of the most significant trends is the integration of machine learning techniques into traditional multivariate analyses. As datasets grow in size and complexity, conventional statistical methods can struggle to provide satisfactory insights. Machine learning offers powerful tools that can automate, enhance, and refine the analysis process.
By combining multivariate statistics with machine learning, researchers can uncover patterns that may not be readily apparent through traditional methods. For example, algorithms such as Random Forest or Support Vector Machines can assist in classifying data points while simultaneously accounting for multiple dependent variables.
This integration also opens doors for improved predictive modeling. Researchers can harness techniques like decision trees and neural networks in combination with multivariate frameworks to improve accuracy and reliability in forecasts. However, this trend requires careful consideration of model assumptions and the interpretability of the outputs. The findings from such analyses can be more difficult to communicate effectively to stakeholders lacking technical expertise.
Emerging Applications
The applications of multivariate test statistics are expanding rapidly across various fields, driven largely by technological advancements and growing interdisciplinary collaboration. One area of notable focus is in health sciences. Here, researchers are using multivariate techniques to analyze patient data comprehensively, enhancing diagnosis and treatment decisions. Integrative approaches that consider genetic, clinical, and environmental data are now being developed, leading to personalized medicine strategies.
In social sciences, multivariate analyses play a crucial role in deciphering complex behavioral data. With emerging tools, researchers can explore various factors affecting human behavior comprehensively, providing deeper insights than single-variable analyses ever could.
Furthermore, the business sector is witnessing a surge in the use of multivariate statistics for marketing research, especially in understanding consumer behavior through multi-dimensional datasets. Companies analyze customer preferences, buying patterns, and demographic information collectively to tailor marketing strategies that resonate more effectively with their audience.
The integration and application of advanced multivariate techniques ensure researchers can tackle complex real-world problems with actionable insights, proving invaluable across diverse fields.
This intersection of multivariate statistics with machine learning and the emergence of new applications not only enhances the field's analytical capacity but also invites a new era of methodological evolution that is likely to shape future research directions.
Finale
The conclusion serves as a critical component of any research by summarizing and synthesizing the key points discussed throughout the article. It reinforces the significance of multivariate test statistics within research disciplines. In understanding this multifaceted area, researchers can make informed decisions about applying these statistical techniques to their work.
The summary of key insights is not just a recap but an opportunity to reiterate the essential concepts and findings highlighted earlier. This reinforces knowledge and aids recall for the reader, especially students and professionals who might revisit the material in the future. By clearly stating the findings, conclusions drawn from various methodologies, and potential pitfalls, the reader leaves with a coherent understanding of how to navigate the complexities of multivariate analysis.
Additionally, emphasizing the importance of continued research is vital. The field of multivariate statistics is ever-evolving, with new techniques and applications emerging regularly. It encourages researchers to stay updated with advancements, fostering a culture of lifelong learning. There is also a collective responsibility among scholars to refine methodologies, improve interpretations, and contribute to the broader understanding of statistical practices in research.
In summary, the conclusion wraps up the discussion while spotlighting the ongoing journey of research in this area. It makes clear that thorough knowledge of multivariate test statistics will not only enhance individual performance in research but also contribute to the body of knowledge in various fields.
"Research is a continuous journey, where every finding leads to new questions and areas to explore."
This approach underscores the necessity for scholars to engage actively with the topic and to push boundaries in their respective fields by applying these complex statistical methods thoughtfully and accurately.