Understanding Data Provenance: Importance and Applications
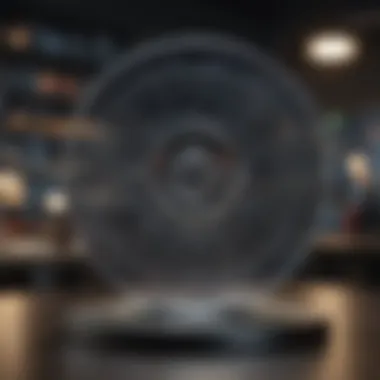
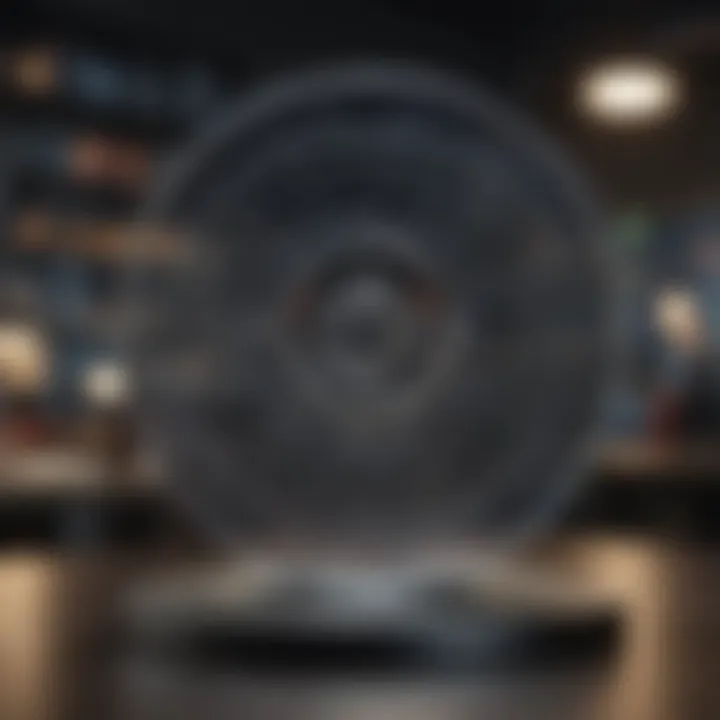
Intro
Data provenance is an essential aspect of data management that serves to clarify the journey of data from its origin to its final use. It encompasses all changes that occur to the data along the way, including transformations, movements, and storage. In today’s data-saturated environment, the importance of tracking data provenance cannot be overstated. As we leverage vast amounts of information across various disciplines—such as research, healthcare, and finance—the ability to trace the lineage of data provides accountability and enhances decision-making.
Understanding the principles behind data provenance equips students, researchers, and professionals with the knowledge needed to manage data responsibly. It aids in verifying the integrity of data, ensuring that processes comply with legal and ethical standards, and can significantly improve the reproducibility of results in scientific research.
This article delves into key facets of data provenance, highlighting its definition, significance, the methodologies used to track it, various use cases, and the technological frameworks that support these processes. By exploring these areas, we aim to illuminate how data provenance contributes to informed decision-making and robust data management practices.
Key Findings
Major Results
The concept of data provenance leads to several critical findings:
- Enhanced Transparency: Documentation of data origins allows users to understand where the data comes from, thereby increasing trust in the information presented.
- Accountability: Establishing a trail for data usage ensures that responsible parties can be identified, which is vital in regulatory environments.
- Reproducibility of Results: In research, being able to track data provenance supports the reproducibility of findings by allowing others to access the same data and processes used.
Discussion of Findings
The findings point toward a growing recognition of the need for comprehensive data governance models that integrate data provenance tracking. For instance, in healthcare, the ability to track patient data from entry to analysis improves not only patient outcomes but also the efficacy of healthcare systems. Similarly, in finance, the ability to trace the lineage of financial transactions aids in detecting fraud and ensuring compliance with regulations.
In sync with these findings, businesses and organizations are increasingly adopting advanced data provenance technologies. This trend underlines the necessity for consistent data management practices that align with ethical standards and regulatory frameworks.
Methodology
Research Design
This article employs a qualitative research design to explore the multifaceted topic of data provenance. It synthesizes existing literature, case studies, and technological frameworks that focus on data lineage tracking.
Data Collection Methods
Data were collected from various sources, including:
- Peer-reviewed articles from reputable journals that discuss data governance and provenance.
- Case studies from sectors such as research, healthcare, and finance that illustrate practical applications.
- Insights from relevant online resources and databases, including en.wikipedia.org and britannica.com.
Through these methods, a deeper understanding of data provenance is constructed, presenting a well-rounded perspective that caters to an audience seeking higher understanding.
Intro to Data Provenance
Data provenance provides several significant benefits:
- Transparency: By documenting the origin and alterations of data, organizations can promote transparency in their operations. Users can see how data was obtained and modified, leading to increased trust between stakeholders.
- Accountability: When data processes are well-documented, it becomes easier to identify who is responsible for potential inaccuracies. This accountability is essential in sectors like healthcare and finance, where data accuracy is paramount.
With these points in mind, it is clear that data provenance is not merely a technical requirement. It encompasses broader implications for ethics and governance in data management. Addressing these elements contributes to a deeper understanding of its impact on various fields.
Defining Data Provenance
Data provenance, at its core, refers to the history of data. It outlines the origins of datasets, including how they were created, who created them, and what processes they underwent. This definition encompasses both the generation of data and its subsequent transformations, providing a holistic view of a dataset's life cycle.
The significance of defining data provenance correctly cannot be overstated. Confusion often arises from unclear terminology in data management. By clearly articulating what data provenance means, organizations can formulate better strategies for data handling, ensuring that individuals involved in data operations are aligned with best practices and standards.
Importance of Data Provenance
Enhancing Data Integrity
Data integrity refers to the accuracy and reliability of data throughout its lifecycle. Provenance provides a clear narrative of data's journey, ensuring that stakeholders can validate data sources and their transformations. When data is traceable, it significantly reduces the risks of errors and fraud, which can have serious consequences, especially in sectors like healthcare and finance where decisions are data-driven. More so, if any data issues arise, tracing back the lineage allows for prompt identification and resolution. Thus, safeguarding the integrity of data is not only a matter of good practice but a necessity in maintaining trust and accountability.
Facilitating Regulatory Compliance
Many industries face strict regulations regarding data management. Data provenance helps organizations comply with these regulations by demonstrating how data is collected, processed, and disseminated. For instance, organizations within the financial sector must adhere to regulations like the General Data Protection Regulation (GDPR) to protect user privacy. Provenance documentation allows firms to show compliance, mitigating potential legal repercussions. By maintaining a transparent data trail, businesses can also provide auditors with the necessary information, should audits occur. Therefore, regulatory compliance is not just about meeting legal requirements; it's about demonstrating a commitment to ethical data practices.
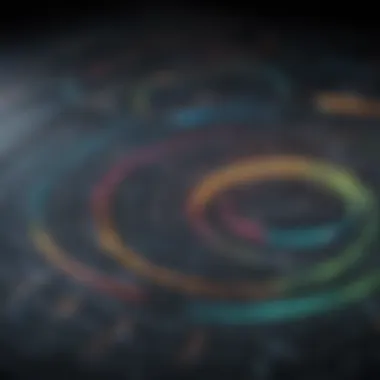
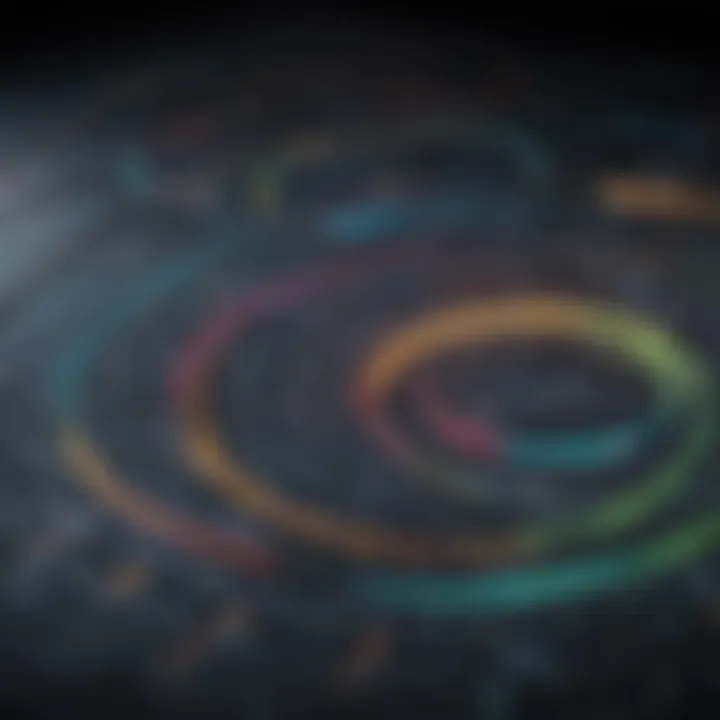
Supporting Reproducibility in Research
Reproducibility is a cornerstone of scientific research. Researchers often build upon previous studies to corroborate findings or explore new avenues. Data provenance ensures that others can trace the data and methods used in experiments. This transparency is vital for validating research results, especially when data is shared across global institutions. Without a clear account of data sources and processing methods, reproducibility suffers, thus undermining scientific integrity. Provenance effectively empowers scholars and institutions to replicate findings or challenge existing narratives, fostering innovation and growth in academia.
Increasing Data Trustworthiness
Trust is a critical component for any data-driven decision-making process. Without trust, the effectiveness of data diminishes. Provenance contributes to building this trust by offering visibility into the processes that shape the data. When users can access comprehensive provenance information, they develop greater confidence in the data they utilize. For institutions managing sensitive data, such trust can also lead to stronger relationships with clients and stakeholders. By providing assurance that data has not been tampered with, organizations can enhance their reputation and credibility within their sectors.
In summary, the importance of data provenance cannot be overstated. It enhances integrity, facilitates compliance, supports reproducibility, and builds trust among users. Each of these elements is interconnected and plays a vital role in the overall effectiveness of data governance.
Core Components of Data Provenance
Understanding the core components of data provenance is essential for grasping how data's journey impacts its validity and applicability. Provenance acts as a key driver behind data governance and management. This section will focuss on two main elements: provenance metadata and data lineage. Both play crucial roles in ensuring transparency and understanding of data sources and transformations.
Provenance Metadata
Provenance metadata refers to the information that describes the history of a data item. It involves documenting the creation, usage, and transformations of the data and can include details like authorship, generation process, and changes made over time. The key benefits of provenance metadata are as follows:
- Traceability: It provides a clear way to trace back the life of data from its original source to its current state. This is critical in fields where accuracy is paramount, such as healthcare or finance.
- Improved Data Management: By maintaining detailed metadata, organizations can enhance their data management practices, leading to better data quality and reduced risk of errors.
- Facilitates Auditing and Compliance: Organizations can effectively prove compliance with regulations when they have robust metadata tracking. Regulatory bodies often require that organizations document their data handling processes.
Overall, provenance metadata ensures that data is not only retrievable but also interpretable in context.
Data Lineage
Data lineage tracks the flow of data as it moves through various processes. It provides a visual representation of data transformations from source to destination. Understanding data lineage offers several advantages:
- Insight into Data Relationships: It reveals how different data points relate to each other. This is particularly valuable in complex systems where multiple datasets are integrated.
- Impact Analysis: Organizations can analyze the effects of changes in data sources or transformations. This helps in making informed decisions about modifications in data processes.
- Debugging and Troubleshooting: When issues arise, knowledge of data lineage allows for quicker identification of problems. Understanding where data originated and how it was modified is essential when diagnosing errors.
In summary, both provenance metadata and data lineage underpin the concept of data provenance. They work together to create a comprehensive view of the data lifecycle, enhancing trust and accountability in the data employed by various organizations.
Methods of Tracking Data Provenance
Tracking data provenance is crucial for understanding how data has evolved over time. Provenance encompasses various stages: from generation to storage, processing, and eventual sharing. Methods used to track provenance can significantly influence data management practices, enhance data quality, and improve transparency. Organizations must consider the chosen tracking method based on their specific needs, scalability, and data types.
Manual Tracking
Manual tracking involves human intervention to document data origin, movements, and changes. It can take many forms: spreadsheets, logs, and lab notebooks. This method has benefits and drawbacks.
Benefits:
- Simplicity: Easy to implement in small projects.
- Flexibility: Allows customization to fit unique workflows.
- Control: Facilitates personal oversight of the data process.
Drawbacks:
- Error Prone: Human mistakes can lead to inaccuracies.
- Time Intensive: Recording data manually can be slow.
- Limited Scalability: Becomes impractical as data volume increases.
In certain contexts, especially with sensitive or small datasets, manual tracking can be effective. However, reliance on human accuracy is a double-edged sword that can introduce risk.
Automated Systems
Automated systems utilize software to track data provenance in real-time. They capture metadata about data sources, transformations, and access logs without manual input.
Benefits:
- Efficiency: Saves time and reduces labor costs.
- Consistency: Maintains uniformity in how data is tracked.
- Scalability: Easily accommodates large data sets and complex systems.
Drawbacks:
- Setup Complexity: Initial configuration can be complicated.
- Potential for Malfunction: Software failures may lead to lost provenance data.
- Cost: May require significant investment in technology.
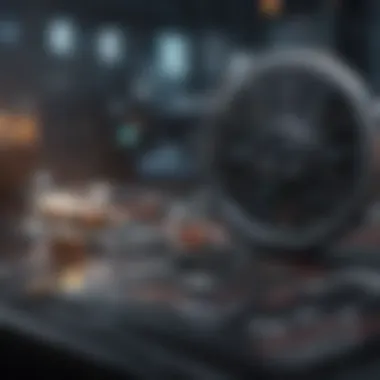
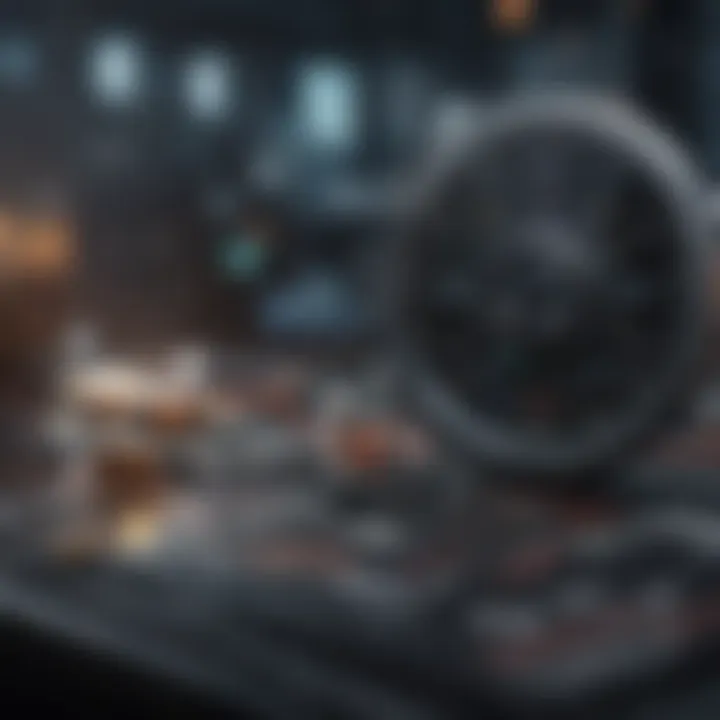
Implementing automated systems can streamline data management significantly but comes with its own challenges. Organizations must weigh these factors carefully.
Hybrid Approaches
Hybrid approaches combine elements of manual and automated tracking. This allows for flexibility and practicality in capturing data provenance. Organizations can adopt a system that fits their specific workflow better.
Benefits:
- Best of Both Worlds: Offer the advantages of manual and automated systems.
- Customization: Adaptable to the complexity of various tasks.
- Resilience: Can mitigate the risk associated with either method alone.
Drawbacks:
- Complexity in Integration: Integrating both methods can be challenging.
- Increased Training Needs: Staff may require training for both systems.
- Overhead Costs: More resources may be needed for maintenance.
Use Cases in Different Domains
Data provenance plays an essential role in various fields by clarifying the history of data, enhancing its reliability, and ensuring compliance with regulations. The implementation of data provenance brings specific benefits tailored to each domain, facilitating informed decision-making and effective data management. By understanding these diverse use cases, stakeholders in healthcare, finance, research, and supply chain can leverage data provenance to drive better outcomes.
Healthcare Applications
In healthcare, data provenance is crucial for tracking patient information, clinical data, and treatment histories. Healthcare systems deal with sensitive data, requiring strict adherence to privacy regulations such as HIPAA in the United States.
- Data Integrity: Provenance metadata helps in retaining the integrity of clinical data. By maintaining a clear record of how data is collected, processed, and analyzed, healthcare professionals can ensure accurate patient care.
- Error Reduction: Tracking the origin of data assists in identifying errors in treatment paths or data entries. This can lead to improved patient outcomes and minimized adverse events.
- Regulatory Compliance: Organizations like hospitals must follow regulations that require transparency in patient data management. Provenance aids in demonstrating compliance during audits and inspections.
Financial Sector Use Cases
In the financial realm, data provenance is vital for maintaining trust and accountability. The rapid evolution of technology allows for improved tracking of financial transactions and audits.
- Fraud Detection: By tracing the lineage of financial data, institutions can detect anomalies and potentially fraudulent activities. This proactive approach to risk management is paramount in protecting assets and customer information.
- Audit Trails: Provenance creates clear audit trails, which are necessary for regulatory compliance in finance. It enables firms to provide detailed reports to regulators, enhancing their credibility and reputation.
- Risk Management: Financial organizations benefit from improved risk assessment by analyzing the history and processes behind their data, allowing them to make better-informed decisions.
Research and Academia
In the realm of research, data provenance ensures the reproducibility and credibility of studies. Modern research relies heavily on data analysis, making origin tracking increasingly important.
- Reproducibility: Provenance allows researchers to document every step of their methodologies. This is vital for allowing other researchers to replicate studies, which is central to scientific validation.
- Data Sharing: Provenance metadata aids in sharing datasets between institutions while ensuring that proper attribution and usage rights are respected. This supports collaboration and innovation.
- Funding Accountability: Research institutions often rely on grants that require transparency in data utilization. Provenance helps demonstrate how funds have been employed effectively in data collection and analysis.
Supply Chain Management
In supply chain management, data provenance ensures that products are traceable from their origins to their final destinations. This is increasingly important in today's globalized market.
- Transparency: By tracking the movement and transformation of products, companies can enhance transparency, which is necessary for consumer trust. Customers increasingly want to know the origins of products.
- Compliance with Standards: Different industries have their regulations regarding safety and quality assurance. Provenance helps organizations adhere to these standards by providing necessary documentation for audits.
- Efficient Recall Processes: If a defect occurs, knowing the precise lineage of products allows companies to conduct effective recalls. This minimizes potential harm and financial loss.
The various use cases of data provenance across sectors not only demonstrate its versatility but also highlight its necessity in modern data management practices. By implementing data provenance, organizations can significantly enhance transparency, accountability, and trust in their respective domains.
Technological Frameworks Supporting Data Provenance
The increasing complexity and volume of data in various domains necessitate robust frameworks that can effectively manage data provenance. This section dissects the technological measures that underpin data provenance, underscoring the benefits and considerations relevant to each. Emphasizing these frameworks enhances the understanding of how data integrity, trust, and accountability can be achieved throughout the data lifecycle.
Blockchain Technology
Blockchain technology plays a pivotal role in maintaining the integrity of data provenance. Its inherent characteristics of decentralization and immutability foster a transparent environment where data origin and its transformations can be recorded securely. The distributed nature means that once information is recorded, it cannot be altered retroactively. This is especially beneficial in fields like finance and healthcare, where trust is paramount. Transactions on a blockchain can be traced back to their origins, thus providing a chain of custody that is auditable by relevant stakeholders.
Blockchain can also facilitate smart contracts. These automated agreements allow for conditions to be set regarding data usage, ensuring that the terms are met only when all parties agree. This introduces an additional layer of governance around data sharing, leading to increased compliance with regulations.
Provenance-aware Databases
Provenance-aware databases are specialized systems designed to track the origins and changes of data as it is manipulated or transferred. They maintain detailed metadata that documents every transaction involving the data. This level of tracking aids in understanding the complete history of any data artifact, which is crucial for verifying authenticity and reliability.
The implementation of provenance-aware databases can considerably enhance data quality. Users can quickly retrieve information about where data originated, how it was processed, and any modifications made along the way. Moreover, these databases can facilitate debugging and error detection, as stakeholders can trace back specific data states that may have led to erroneous outcomes.
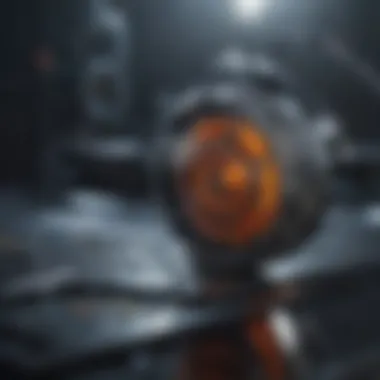
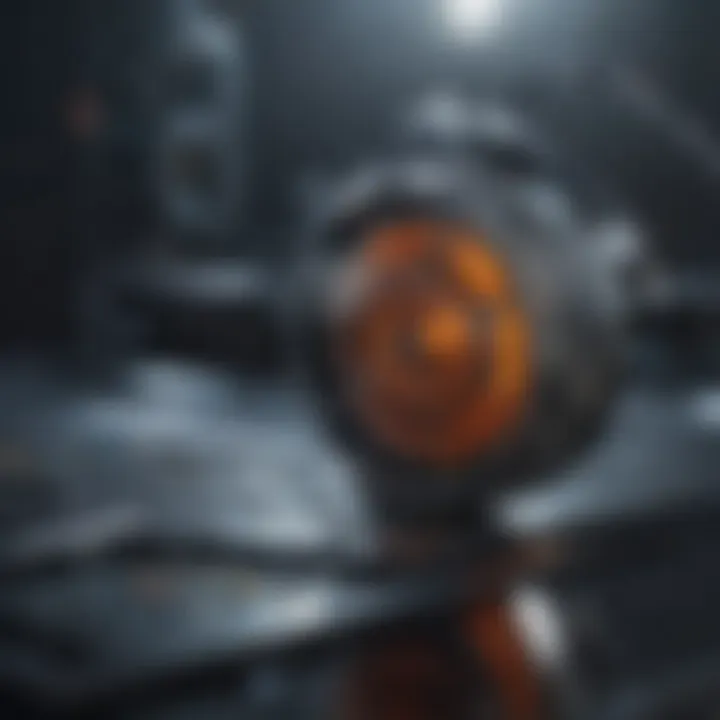
Organizations can choose between different types of provenance-aware systems. Some are integrated within existing database management systems, while others are standalone solutions. The choice depends on the specific needs of the organization, including the volume of data processed and the complexity of data transactions.
Data Provenance in Cloud Environments
With the rise of cloud computing, data provenance takes on additional significance. Cloud environments often involve multiple stakeholders and platforms, making it harder to maintain a clear track of data ownership and transformations. By adopting data provenance strategies in cloud settings, organizations can ensure that they manage data contextually, even when it is distributed across various services.
Cloud providers may offer built-in capabilities for tracking data provenance. These tools allow organizations to monitor how their data is handled throughout its lifecycle, from creation to deletion. Such oversight is critical for meeting compliance requirements and enhancing operational efficiency.
"Effective data provenance frameworks are not just a benefit, but a necessity in today’s data-driven landscape."
Challenges in Implementing Data Provenance
Data provenance is essential for effective data governance, but the implementation process is fraught with challenges. Addressing these obstacles is critical to harnessing the full potential of data provenance. By understanding the key issues involved, organizations can better prepare to integrate provenance frameworks into their data management practices.
Data Privacy Concerns
One of the foremost challenges in data provenance is ensuring data privacy. As organizations track data from its origin to its final usage, sensitive information may be exposed unintentionally. This risk is significant in fields like healthcare and finance, where personal data is often involved.
Regulatory adherence is crucial. Laws such as the General Data Protection Regulation (GDPR) in Europe impose strict guidelines on how data must be handled. Failing to account for these laws can lead to severe penalties. Thus, organizations must implement stringent data access controls and anonymization techniques while still ensuring effective tracking. This balance can be difficult to achieve, often requiring complex solutions that blend technology with policy.
Scalability Issues
As organizations grow, so does the volume of data they handle. Scalability is another significant challenge in implementing data provenance systems. Many traditional systems can struggle to process large datasets efficiently, creating bottlenecks that hinder real-time data analysis.
A solution requires careful investment in technology. Many organizations may need to migrate to cloud-based platforms that offer scalable solutions. Moreover, they must ensure that provenance tracking does not degrade performance. This can involve adopting high-throughput systems and architectures that maintain performance even when scaling.
Interoperability Challenges
Interoperability arises when different systems have difficulties communicating with one another. Organizations often use diverse data sources, which may not have compatibility with existing provenance structures. The lack of standardization in data formats and storage can create significant barriers to visibility across systems.
To tackle this issue, organizations should prioritize the adoption of standardized protocols. Integrating provenance-aware databases can also enhance data collaboration across platforms. Without addressing interoperability, organizations risk creating silos in their data management strategies, which negates the benefits of robust provenance tracking.
It's essential to recognize that the successful implementation of data provenance requires overcoming various hurdles that make it both a complex and critical undertaking.
Future of Data Provenance
The future of data provenance stands at a pivotal intersection of innovation and necessity. As the world increasingly relies on data as an essential asset, understanding how this data is generated, managed, and utilized becomes critical. The rise of big data analytics, coupled with growing concerns over data privacy, security, and regulatory compliance, underscores the need for robust data provenance practices.
Advancements in technology are not only shaping the ways in which data provenance is tracked and managed, but also how it is conceptualized within various industries. Growing demands for transparency and accountability in data handling emphasize the significance of data provenance as a principle for effective data governance. Data provenance will likely evolve to encompass more sophisticated tracking methods that can handle vast datasets while ensuring that users retain control over their data.
Emerging Trends
Several trends are shaping the future of data provenance. These include:
- Increased Focus on Privacy: With regulations like GDPR and CCPA, the necessity for data provenance is highlighted. Companies will invest more in solutions providing transparent data trails that demonstrate compliance.
- Integration with Data Ethics: Ethical considerations surrounding data usage will be more prominent, demanding robust provenance tracking to ensure responsible handling of data.
- Rise of Decentralized Systems: Blockchain technology is gaining traction for its potential to provide immutable data records. This allows for enhanced tracking of data provenance across decentralized networks.
- Real-Time Provenance Tracking: As organizations strive for agility, real-time tracking solutions will become vital, allowing instant insights into data lineage and accessibility.
The convergence of these trends signifies that data provenance will not only be a technical discipline but also a fundamental aspect of ethical data usage.
The Role of Artificial Intelligence
Artificial intelligence is set to play a transformative role in the realm of data provenance. AI can automate processes related to data tracking, sorting, and analysis, significantly enhancing efficiency. Some key aspects of AI's impact on data provenance include:
- Automated Metadata Generation: AI can facilitate the generation of provenance metadata, allowing organizations to capture critical details with minimal human intervention.
- Enhanced Data Quality Control: Machine learning algorithms can identify anomalies and inconsistencies in data lineage, leading to improved accuracy and reliability.
- Predictive Analytics: AI can analyze historical provenance data to forecast future trends and inform strategic decision-making.
- Natural Language Processing: Using NLP, AI can analyze documentation and communications related to data sources, further enriching the provenance narrative.
In summary, the future of data provenance will intertwine with technology's trajectory, particularly with AI, as organizations prioritize both compliance and innovative data management practices.
Concluding Thoughts
The discussions surrounding data provenance unveil its undeniable significance in today's data-driven world. As data continues to proliferate across various sectors, understanding its origins and lifecycle becomes crucial for ensuring reliable outcomes. This section emphasizes the relevance of data provenance, focusing on specific elements that define its importance.
The Imperative of Data Provenance
Data provenance serves as a backbone for effective data governance and informed decision-making throughout diverse industries. Its fundamental role can be summarized through several key benefits:
- Transparency: It enhances visibility into data's origins, movements, and transformations. When users know where data comes from, they can make more discerning decisions on its use.
- Accountability: Provenance establishes ownership and accountability. Knowing who generated or modified data can mitigate issues related to data misuse or errors.
- Trustworthiness: By documenting data's history, organizations foster increased trust among users. Trust is essential, particularly in sensitive fields such as healthcare and finance.
- Compliance: Regulatory frameworks often demand thorough documentation of data sources and transformations. Provenance supports adherence to these regulations, diminishing risks for organizations.
- Reproducibility: In research contexts, the ability to reproduce results hinges on clear data provenance. This metric allows researchers to validate findings and build on each other's work effectively.
As we move forward into an era dominated by data, embracing the principles of data provenance is imperative for students, researchers, educators, and professionals alike. Adequate emphasis on these aspects will lead to improved practices and a more resilient data landscape.