Understanding Data Lineage in Governance Practices
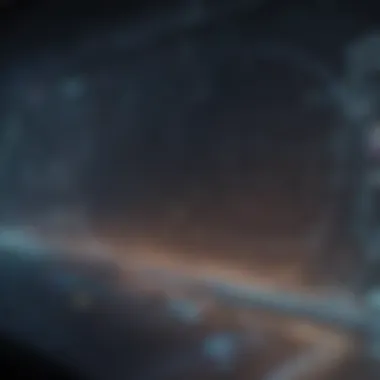
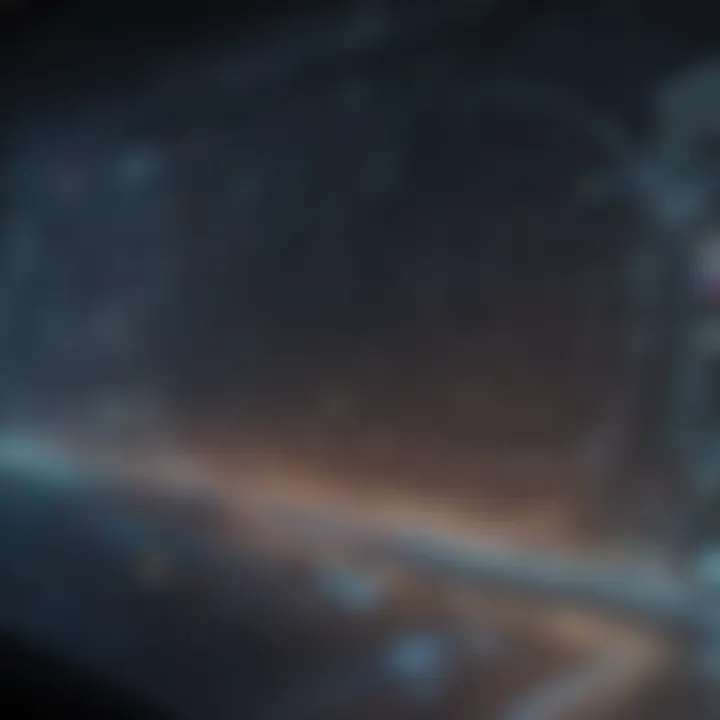
Intro
In today's data-driven world, the concept of data lineage is slowly becoming a cornerstone of effective data governance. It’s not just about collecting data—it's about knowing where that data came from, how it has changed over time, and where it is utilized. This knowledge is crucial for any organization that aims to maintain data integrity, comply with legal regulations, and ensure proper data stewardship.
Understanding the flow of data through its various transformations helps organizations make informed decisions, manage risks, and improve overall data quality. In this segment, we shall address the integral findings regarding data lineage, emphasizing its significance within governance frameworks.
Key Findings
Major Results
- Visibility: Organizations that implement rigorous data lineage practices gain enhanced visibility into their data streams. This transparency can be vital for quickly identifying sources of errors or discrepancies.
- Compliance: Regulatory bodies increasingly require detailed data lineage documentation. The ability to trace data origin and alterations supports compliance with standards such as GDPR and HIPAA.
- Data Quality: By tracking data transformations and flows, organizations can ensure higher data quality, reducing instances of inaccurate or outdated data affecting business decisions.
- Operational Efficiency: Clear data lineage mapping can streamline processes by identifying redundant data flows and optimizing resource allocation within IT systems.
Discussion of Findings
The findings above underline a crucial reality: with the explosion of data, having an efficient data governance structure is no longer an option; it's a necessity. In particular, data lineage serves as the skeleton of this structure.
Without a clear understanding of how data moves and transforms, organizations can find themselves in deep waters. Imagine tackling a compliance audit without being able to illustrate data paths—an impossible task! Such challenges underscore the necessity of data lineage in today's regulatory climate.
Moreover, the impact of poor data management can ripple outwards, influencing not just internal operations but also client relationships and market positioning. A single data mishap might tarnish an organization's reputation; thus, establishing resilient data lineage practices is not just advantageous but essential.
Methodology
Research Design
This examination of data lineage is built on a mixed-methods approach that combines qualitative case studies and quantitative analyses. By investigating various organizations that have effectively implemented data lineage practices, we can derive insights applicable across diverse sectors.
Data Collection Methods
Data for this study was derived through:
- Interviews: Engaging with data governance experts and practitioners provided firsthand accounts of challenges faced and solutions implemented.
- Surveys: Collecting responses from organizations on their current data lineage practices yielded valuable statistics.
- Document Analysis: Examining existing data lineage frameworks and regulatory documentation offered context on best practices.
"Data lineage is to data governance what a roadmap is to a traveler—without it, one risks getting lost in the complexity of information."
In summation, the interplay between data lineage and data governance reveals a critical path for organizations navigating the complexities of data management today. As we navigate this article, we'll explore more nuanced aspects, tools, and the pressing challenges faced in the field.
Preface to Data Lineage
In the world of data governance, understanding data lineage stands as a cornerstone for effective data management. It goes beyond simple data tracking; it encapsulates a framework that reveals not only where data originates but also how it ebbs and flows through various systems, ultimately arriving at its final state. With the growing complexity of data systems and the increasing reliance on data-driven decision-making, having a clear picture of data lineage becomes essential.
Achieving insight into data lineage enables organizations to identify data sources, trace data transformations, and understand the various processes that influence data integrity. This is crucial for maintaining data quality, where simply knowing the source of the data is not enough; organizations should also grasp how the data has changed over time to draw accurate conclusions.
Moreover, in today's regulatory environment, where compliance is paramount, organizations that prioritize data lineage can better navigate the labyrinth of legal requirements. It fortifies their positions when subjected to audits, ensuring they can demonstrate the lifecycle of data from its origin through to its final use.
Definition and Significance
Data lineage refers to the ability to track and visualize the flow of data through its entire lifecycle—from its source to its ultimate destination. It shows how data is transformed, derived, and ultimately used, providing a comprehensive overview of the flow of data within a system.
Why is this significant? First and foremost, understanding data lineage can dramatically enhance data quality. When companies have a holistic view of their data's journey, they can implement better controls and validation checks to ensure that the data being utilized is both relevant and accurate. Furthermore, it aids in the identification of potential issues early in the data processing stages, allowing for timely corrections.
Additionally, data lineage plays a critical role in compliance. Organizations are often required to adhere to stringent regulations regarding data use, and a clear mapping of how data is handled can simplify demonstrating compliance during audits. Companies that disregard this may find themselves on shaky ground, susceptible to legal ramifications.
In focusing on these advantages, it’s apparent that robust data lineage practices are not just an additive luxury; they are fundamental to the fabric of effective data governance, ensuring organizations can trust their data and derive actionable insights from it.
Historical Context
The concept of data lineage isn't something that hatched overnight. Historically, as organizations began to grapple with vast amounts of data, the necessity to track data became apparent. Early databases showed rudimentary lineage visuals, but these were often limited and difficult to interpret.
In the 1990s, as businesses began to recognize the value of data as a strategic asset, the strategies surrounding data management evolved significantly. The proliferation of data warehousing offered a more structured approach, driving the demand for tools that could map data paths. Vendors began developing solutions which aimed at visualizing this complexity, thus laying the groundwork for contemporary data lineage tools.
Fast forward to today—data lineage has become a vital part of any data governance framework, influenced by technological advancements such as cloud computing and big data analytics. With the volume and variety of data growing exponentially, having a strong grasp of data lineage is no longer optional; it’s practically a mandate for those wishing to navigate the modern data landscape successfully. By appreciating the historical journey of data lineage, it becomes clear how integral it is within the broader discussion of data governance.
Core Components of Data Lineage
Understanding the core components of data lineage is key to grasping how data moves through various systems and applications. This segment serves as a foundation for navigating the complexities of data governance, especially in environments rich with diverse data sources and various transformations. Each layer of data lineage offers insights into data management practices, ensuring quality and compliance, which are crucial for any organization.
Data Origin
Data origin refers to the initial point where data is collected or created. This can range from transactions in financial systems to inputs captured via IoT devices. The significance of identifying data origin cannot be overstated. It establishes accountability and traceability, which becomes vital during audits or when rectifying issues arising from data inconsistencies.
The movement into comprehensive data governance starts by documenting who collected the data, how it was captured, and under what circumstances. For instance, consider a scenario in a retail setting: if a customer transaction data is recorded, understanding not just the transaction itself but also the device used for capturing that data (like a cash register or e-commerce platform) can help attribute potential errors to specific systems.
- Establishing trust in data quality
- Ensuring compliance with regulations
- Facilitating easier troubleshooting and historical audits
Data Movement
Data movement encapsulates how data traverses between systems, databases, and applications after its inception. It is critical to monitor this flow to ensure data integrity in transit. Data movement can occur through various means, such as batch processing, streaming, or even direct system-to-system transfer.
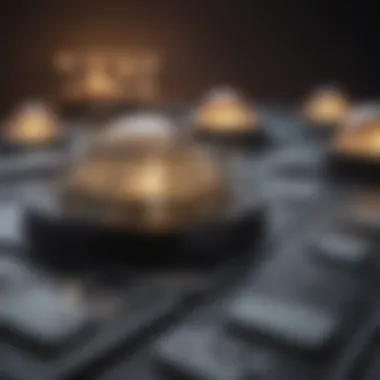
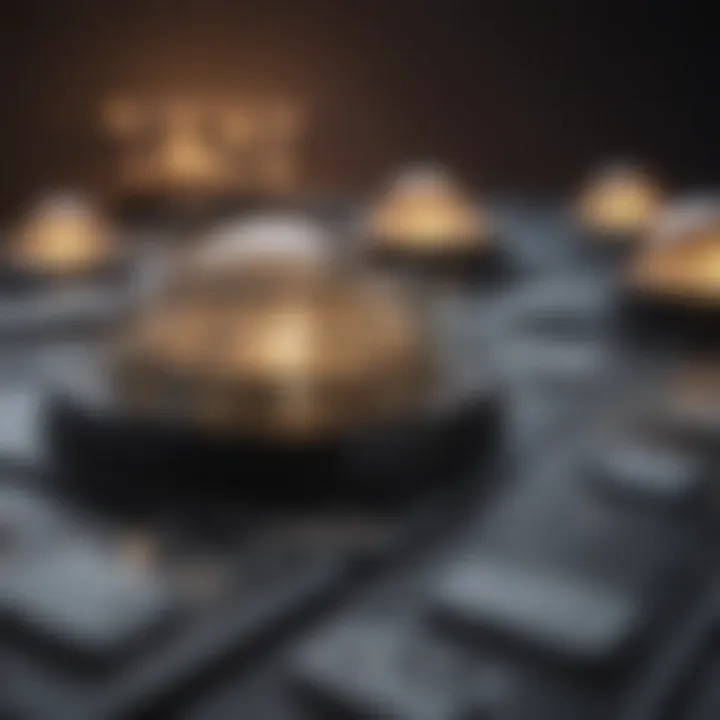
Each movement feeds into the bigger picture of data lifecycle management. When data changes hands, tracking those movements becomes essential for understanding its state at any given moment.
For example, a hospital might collect patient information through various applications like Electronic Health Records (EHR) systems, which are often interfaced with other health management software. If a health auditor needs to verify treatment data, they should be able to follow the transition of data from the point of collection through to its storage in multiple applications.
"Accuracy in understanding data movement plays a direct role in regulatory compliance and operational efficiency."
Transformation Processes
Transformation processes inject complexity into data lineage. They involve the modifications data undergoes during its lifecycle, which includes anything from data cleaning and aggregation to analyses and reporting. These processes can alter the data structure, format, or even its semantics, making them pivotal focal points in lineage tracking.
For instance, if raw sales data from a point of sale system is transformed into reports for business intelligence, it's crucial to document the algorithms or manipulations applied during this transformation. Without this clarity, end-users may misinterpret the meaning or significance of the reports generated.
Key benefits of understanding transformation processes include:
- Enhancing transparency in how decisions are made
- Supporting accurate data analysis through credible sources
- Meeting compliance requirements by documenting transformations for audits
In summation, the components of data lineage—data origin, movement, and transformation processes—are interlinked in a way that reinforces the entire conceptual framework of data governance. Each piece of this puzzle is essential for fostering a culture of data awareness and sound governance practices that can evolve with the changing landscape of data management.
Importance of Data Lineage in Data Governance
In the realm of data governance, the importance of data lineage cannot be overstated. It serves as the backbone for understanding how data flows through an organization, from its origin to its ultimate destination. When organizations implement effective data lineage practices, they gain a clear, comprehensive view of their data landscape, which helps to ensure the integrity and quality of their data assets.
For data professionals, having a solid grasp of data lineage is essential for multiple reasons. It enables better decision-making and enhances transparency within the data management process. Many institutions today operate under a cloud of uncertainty when it comes to their data. This lack of clarity can lead to errors, compliance violations, and inflated risks, ultimately jeopardizing an organization’s credibility and reliability in the eyes of stakeholders.
A well-documented lineage can bring forth a variety of specific benefits:
- Traceability: Stakeholders can track how and where data originates, making it much easier to pinpoint issues when they arise.
- Data Quality: Understanding the various transformations and movements allows organizations to maintain high standards of data integrity.
- Regulatory Compliance: Knowing the data path is crucial for adhering to data governance regulations that mandate documentation and accountability.
- Enhanced Data Management: Clear visibility into data flows enables organizations to optimize processes, eliminate redundancies, and improve overall efficiency.
In short, effective data lineage in data governance is akin to having a detailed map in an unfamiliar territory; it offers essential insights that empower data stakeholders to navigate the complexities of today’s data landscape.
Enhancing Data Quality
Data quality is a cornerstone of effective governance, and data lineage plays an instrumental role in enhancing it. When organizations faithfully document their data flows and transformations, they’re better equipped to identify anomalies or errors that could potentially impact the end quality of their datasets. For instance, if a dataset's integrity comes into question, tracing back through the lineage allows data managers to identify where the fault occurred—a crucial advantage in prompt remediation.
Furthermore, good practices in data lineage encourage regular audits. By establishing a routine for reviewing data lineage records, organizations can proactively address quality issues before they escalate into compliance concerns or operational hiccups. Regular audits also promote a culture of accountability, ensuring that every action taken on data is backed by thorough documentation.
Regulatory Compliance
Regulatory bodies are increasingly emphasizing the importance of transparency in data handling. Laws such as the General Data Protection Regulation (GDPR) and the California Consumer Privacy Act (CCPA) have stringent requirements for organizations regarding data provenance and accountability. A robust data lineage framework helps organizations demonstrate compliance with these regulations by providing a clear trail of where data comes from, how it’s used, and the transformations it undergoes along the way.
In case of audits or investigations, having a systematic record of data lineage can save organizations from hefty fines and damaged reputations. It’s like having an insurance policy that reassures stakeholders of the organization’s commitment to ethical data practices. Moreover, with the rise of cross-border data flow, understanding lineage becomes pivotal—not just for compliance but for building trust among users who demand more transparency.
Risk Management
Managing risks related to data is becoming more critical as organizations increasingly rely on data for strategic decisions. Inadequate data governance can expose organizations to various risks—from data breaches to wrong business decisions stemming from poor data quality. By employing data lineage practices, organizations can better mitigate these risks.
Identifying vulnerabilities in data processes and flows allows for timely interventions. For example, if a significant transformation process is found to be flawed, organizations can act quickly to rectify it before the inaccurate data propagates through systems, leading to larger issues down the line. Additionally, having a clear understanding of data lineage enhances incident response capabilities; when problems arise, teams can quickly trace affected data back to its source, allowing for a more effective response.
Data lineage is not merely a technical necessity; it’s a strategic advantage in today’s complex and evolving data ecosystem.
Data Lineage Models
Understanding data lineage models is central to grasping the entire framework of data governance. These models function as blueprints that illustrate how data flows through various states—from its inception to its final use. Each lineage model serves distinct purposes, offering insights into data handling that can be leveraged for improving quality, compliance, and ultimately paving the way for solid decision-making processes.
Physical Lineage
Physical lineage pertains to the actual data stored on physical systems—think servers and databases. This model provides a granular look at where data lives, highlighting its origins, the various pathways it traverses, and its final resting places. It’s akin to cartography for data, mapping out all the nooks and crannies where information is kept.
The significance of physical lineage lies not just in tracking, but ensuring data availability and performance. For instance, understanding how data replicates across cloud services or on-premise servers can illuminate potential bottlenecks that might affect retrieval times. Furthermore, it aids in resource allocation, illuminating areas that may require more robust infrastructure to handle data loads efficiently.
Logical Lineage
On a different rung of the ladder, logical lineage abstractly represents how data is transformed and utilized across different systems. This model focuses less on the physical characteristics and more on data connections, workflows, and the logical mapping of data elements. For instance, consider a scenario where sales data from multiple regions is consolidated into a unified report; logical lineage would track how all those disparate pieces come together logically, revealing the integration processes and transformation applied.
Understanding logical lineage becomes vital when interpreting data flows for insights. By seeing data in a more abstracted form, organizations can manipulate and analyze it to derive meaningful conclusions. Additionally, it allows for ensuring that business rules are consistently applied, which is critical for governance and compliance checks. Logical lineage also tends to bridge the gaps between technical and non-technical stakeholders, fostering collaboration by making data processes more comprehendible.
Business Lineage
Business lineage dives into the world of business interactions, showing how data informs decisions within an organization. It emphasizes the context of data usage, illustrating how information translates into actionable insights. For example, how does customer feedback translate into product development decisions? Business lineage reveals that journey.
The importance of business lineage can't be overstated. It encapsulates the who, what, and why of data movement and transformation. Properly documenting this lineage helps organizations understand the value derived from data, ensuring alignment between actual data use and strategic objectives. By engaging stakeholders in identifying how data serves their needs, organizations can create a more data-driven culture, ultimately improving decision-making.
Understanding the nuances of these models equips organizations with a comprehensive view of their data landscape, enabling them to make informed, strategic choices.
Tools and Technologies for Monitoring Data Lineage
In the realm of data governance, the tools and technologies available for monitoring data lineage play a pivotal role. As organizations continue to amass vast amounts of data, the necessity of comprehensively tracking its journey becomes more pronounced. This not only aids in ensuring data quality but also in compliance with various regulations. Identifying where data originates, how it transforms, and how it flows through different systems empowers businesses to make informed decisions and mitigate risks associated with data mismanagement.
ETL Tools
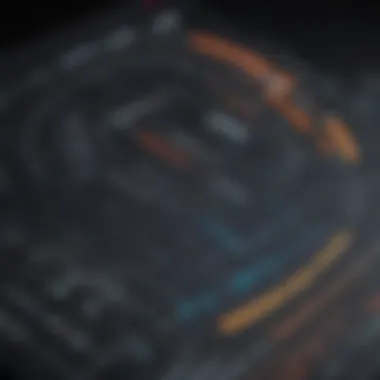
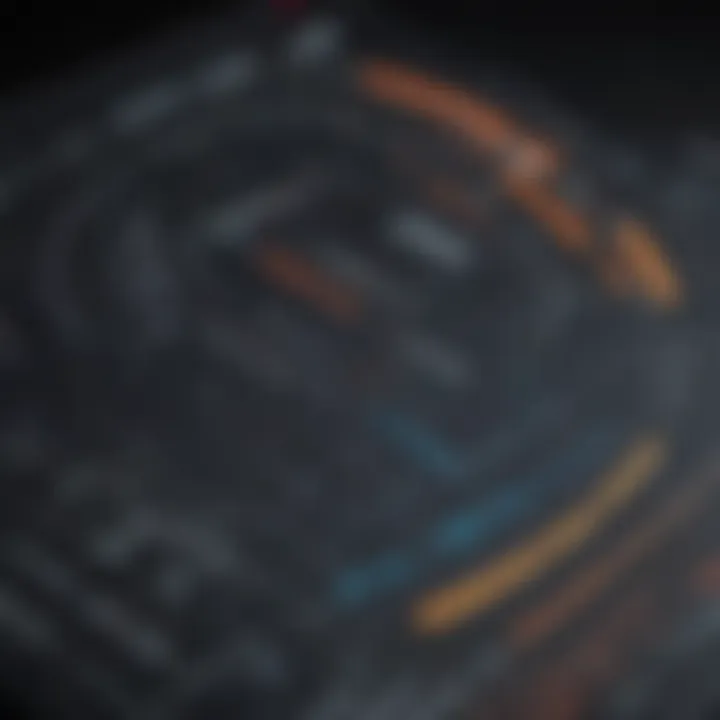
Extract, Transform, Load (ETL) tools are critical in managing the stages of data processing. These tools help in capturing data from multiple sources, preparing it, and storing it in a target database. By utilizing ETL processes, organizations can establish a transparent path of data movement. The critical aspect here is that an effective ETL tool provides clear visibility into the steps taken to transform and load data.
- Benefits of ETL tools include:
- Streamlined data processing
- Enhanced data integrity
- Simplified tracking of data flow
For instance, Informatica PowerCenter comes to mind as a tool that offers robust features for monitoring data lineage, helping organizations not only to manage data extraction but to analyze the lineage intricacies involved in the transformation process.
Data Catalogs
Data catalogs serve as comprehensive inventories of data assets across an organization. They provide a systematic way to organize, manage, and discover data. Having a good data catalog enables teams to understand the lineage of data more intuitively, as it often includes metadata that describes data sources, formats, and usage throughout the data pipeline.
- Key features of data catalogs are:
- Search and discovery capabilities
- Metadata management
- Data stewardship tools
With solutions like Alation, businesses can create a self-service environment where users can discover data while also tracing its lineage. This not only promotes data usage but also increases accountability among users regarding data quality.
Metadata Management Solutions
Metadata management solutions are crucial for maintaining a consistent understanding of data lineage. They provide the context needed to interpret data accurately. Metadata encompasses data about data, capturing information like its origin, transformations, and the context in which the data was generated.
- The significance of metadata management includes:
- Enabling data discovery
- Facilitating compliance and audits
- Supporting data governance frameworks
For example, solutions such as Collibra shine in this area, helping organizations link data assets and their lineage. As data flows through various systems, maintaining a metadata repository allows teams to ensure that they comprehend the changes and derivations data undergoes.
"Understanding the journey of data is as vital as protecting the data itself. - Unknown author"
To sum up, these tools and technologies are not just companions in the data governance journey; they are essential pillars supporting the structure of modern data management practices. As businesses navigate their data landscape, leveraging ETL tools, data catalogs, and metadata management solutions can uncover valuable insights and enhance overall data governance.
Challenges in Implementing Data Lineage
Implementing data lineage is not a walk in the park. While the benefits are abundantly clear, the hurdles that come with it can be quite the challenge. This section dives into the intricacies of the roadblocks faced by organizations, focusing on three main aspects: data complexity, integration issues, and cultural resistance. Understanding these challenges is crucial for laying down a solid framework for data lineage, thereby enabling more effective governance of data assets.
Data Complexity
Data within organizations often exists in a labyrinth of formats, locations, and structures. This jumble is what we call data complexity. The sheer volume of data and its diverse forms—from structured databases to unstructured documents—pose a significant challenge for lineage implementation. Data types and formats may change over time as businesses evolve, leading to a more intricate landscape.
- Diverse Sources: Data is often sourced from various platforms like CRM systems, IoT devices, cloud storage, and even legacy systems. Each source contributes to a broader complexity that needs careful mapping.
- Evolving Data Structures: Data in a business doesn't just sit still; it undergoes modifications, merges, and purges constantly. Keeping track of these changes for lineage purposes can be daunting.
Furthermore, understanding how data moves and transforms across these differing systems requires not just technical prowess but also a comprehensive documentation process. Without it, organizations can find themselves in murky waters regarding data accuracy and traceability.
Integration Issues
Integration issues are like the unexpected potholes in a well-traveled road. When it comes to data lineage, stitching together disparate data sources into a coherent lineage view can be challenging.
- System Compatibility: Not every system plays nicely with others. Differences in technology stacks, data formats, and interfaces can create friction during integration.
- Siloed Data: Often, data is kept siloed within departments, making it tough to have a unified view across the organization. These silos can prevent organizations from understanding how data flows throughout the entire ecosystem.
Moreover, the absence of robust integration frameworks may lead to incomplete data lineage tracking. This disjointed approach can hinder data governance initiatives, making it quite difficult to ensure compliance and quality.
Cultural Resistance
Perhaps one of the most underestimated hurdles is the human factor—cultural resistance within the organization.
- Change Aversion: Employees may be hesitant to adapt to new processes or technologies. Many professionals are already comfortable with the status quo, and change can feel like a daunting mountain to climb.
- Lack of Leadership Buy-in: If leadership doesn't back the initiative, it becomes challenging to shift mindsets across the organization. A lack of clear communication from the top about the importance of data lineage can create barriers to acceptance.
In essence, fostering a culture that embraces data governance requires ongoing training, consistent messaging, and emphasis on the value of data lineage. Leaders need to champion these changes actively, not just during the rollout phase, but as part of an ongoing commitment to effective data management.
The journey to robust data lineage is fraught with complexities, but addressing these challenges head-on is an investment in the organization's future.
In tackling these challenges, organizations may not only improve their data lineage processes but also create a stronger foundation for overall data governance, leading to more informed decision-making and strategic clarity.
Best Practices for Establishing Data Lineage
Establishing effective data lineage is more than just a checkbox on a compliance list; it is a strategy that underpins the integrity and usefulness of data across any organization. When you get it right, the advantages ripple through the data governance frameworks and beyond—improving data quality, facilitating compliance, and streamlining processes. Let’s break down some of the best practices that can be actionable in a variety of settings.
Defining Clear Policies
The foundation of a robust data lineage strategy is the development of clear, structured policies. Organizations should articulate their expectations clearly regarding data management. Without a well-defined policy, confusion may reign, leading to inconsistencies in data handling.
- Document Responsibilities: Employees need to know their roles in managing data. It's critical to specify who is responsible for monitoring data lineage and establishing protocols.
- Establish Data Standards: This involves deciding how data is classified and managed at various stages of its lifecycle. Standards ensure that everyone is on the same page.
In essence, policies serve as a guiding light for your organization’s data initiatives. When established thoughtfully, these policies can transform how data flows and evolves.
Engaging Stakeholders
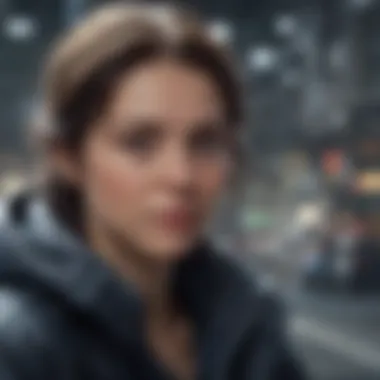
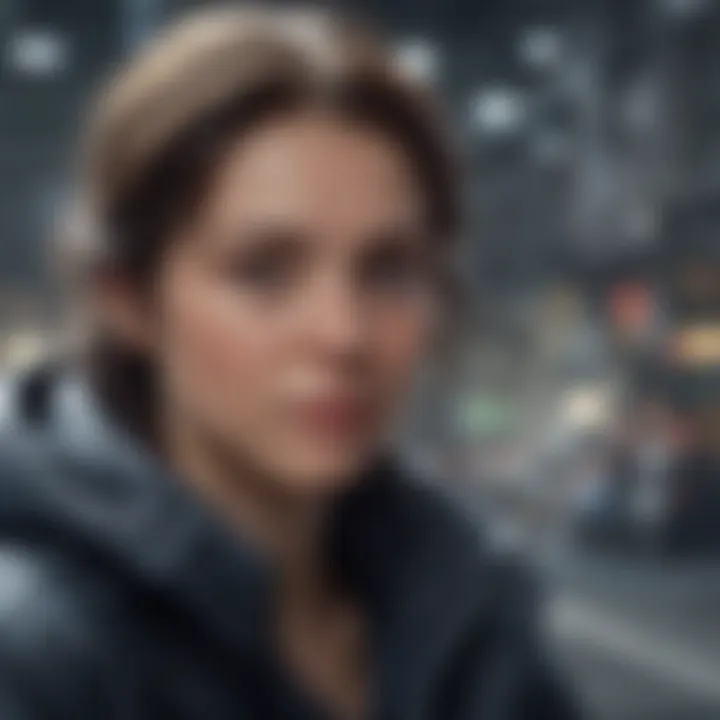
Data lineage isn’t solely a tech concern; it resonates with multiple departments and stakeholders across an organization. Engaging these individuals fosters a collaborative environment where data governance can flourish.
- Identify Key Stakeholders: Who are the data stewards? Which departments heavily rely on data insights? Identifying these players early on is vital.
- Educate and Train: Regular workshops or training sessions can bridge knowledge gaps. When stakeholders understand why data lineage matters, they become invested in maintaining it.
- Solicit Feedback: Encourage open lines of communication. Stakeholders often have invaluable insights that can help refine data lineage practices further.
Engaging stakeholders not only reinforces culture but also ensures that data lineage initiatives receive buy-in across the board. The buzz can help legitimize the efforts undertaken.
Incorporating Automation
In an age overflowing with data, manual tracking of data lineage is a recipe for errors and fatigue. Enter the realm of automation—an indispensable ally.
- Implementation of Tools: Utilize specialized software that automatically tracks data flows. Such tools can simplify the monitoring of changes and movements.
- Real-Time Updates: Automation allows for real-time lineage tracking, keeping your data governance strategies current and effective. No more lagging behind; it’s all immediate.
- Focus on Historical Data: Automated systems often come equipped with capabilities to present historical data lineage as well. This can be crucial for audits and compliance checks.
Automation doesn’t remove the human element but enhances it. By alleviating the burden of repetitive tasks, it allows professionals to focus on strategic initiatives that drive organizational growth.
"By defining policies, engaging stakeholders, and automating processes, organizations can truly harness the power of data lineage. It's like painting a clear picture on a once muddy canvas."
Incorporating these best practices isn’t just beneficial; it’s essential. A well-structured approach can turn data lineage from a mundane necessity into a powerful governance tool. Companies that grasp this will undoubtedly find themselves ahead of the curve.
Case Studies on Data Lineage
Explaining data lineage through practical examples is invaluable. These case studies illuminate the real-world application of theoretical concepts, bridging the gap between knowledge and practice. Understanding how various industries implement data lineage allows professionals to grasp its importance and the nuances involved. In this section, we will delve into three distinct case studies from different sectors – finance, healthcare, and telecommunications. This analysis is not merely an academic exercise; it showcases the potential benefits and challenges faced during implementation.
Financial Sector Implementation
In the financial sector, proper data lineage is paramount. Regulatory agencies expect financial institutions to maintain rigorous standards regarding data accuracy and reporting. A prominent bank identified discrepancies in transaction records during an audit. Without a clear data lineage, the source and movement of these data points remained opaque.
To tackle this issue, the bank invested in a robust data lineage system. They began mapping out data from its origin – customer transactions – through various systems, capturing transformations, and tracking downstream reporting creations. The outcome was twofold: it enhanced data quality while ensuring regulatory compliance. By employing automated monitoring tools, the bank could rectify issues in real-time, reducing the risk of errors and improving customer trust.
Healthcare Data Management
Healthcare relies on accurate data for patient management and regulatory compliance. A renowned health network faced significant hurdles in data tracking while integrating multiple electronic health record systems. Disparate data sources meant it was virtually impossible to trace the history of patient data across systems. This raised concerns, especially regarding patient safety and data privacy laws stipulated by HIPAA.
To address these challenges, the healthcare network adopted an integrated data lineage approach. They developed a centralized database, implementing a metadata management solution that outlined how data flowed through various systems. This system allowed healthcare professionals to visualize the entire patient data lifecycle, ensuring traceability and honing in on data integrity. Thus, the network not only improved compliance but also streamlined operations, contributing to optimal patient care.
Telecommunications Case Study
The telecommunications industry is notorious for handling vast swathes of data, with customer interactions, network performance, and service issues spanning multiple platforms. A telecommunications provider recognized that frequent service interruptions were causing customer churn but lacked visibility into the root causes of these interruptions.
To combat this, they established a comprehensive data lineage framework. By analyzing and visualizing data movement across their network, the provider could pinpoint service outages back to specific network changes. This intelligence led to better decision-making and timely responses to customer complaints. Moreover, internal teams could see the effect of changes in real-time, preventing similar issues from arising again.
"With clear data lineage, you can connect the dots between raw data and action points, allowing for proactive measures rather than reactive fixes."
In all three case studies, each organization learned that investing in data lineage solutions wasn’t just about compliance but also about enhancing overall operational efficiencies. They turned complex data challenges into opportunities for growth while setting a commendable precedent for industry best practices.
Future Trends in Data Lineage
Understanding the future trends in data lineage is crucial for professionals seeking to stay competitive in an ever-evolving data landscape. As organizations become increasingly reliant on data, the ability to trace and manage its movement and transformation has never been more important. Data lineage not only supports regulatory compliance but also plays a vital role in data quality management. Hence, as future developments unfold, adapting and evolving with these trends is essential to ensure substantial benefits.
AI and Machine Learning Constraints
The integration of Artificial Intelligence (AI) and Machine Learning (ML) in data lineage is one area experiencing rapid growth. Organizations are beginning to utilize these technologies to automate lineage tracking processes, bringing efficiency that was previously much harder to achieve.
However, there are several constraints to consider:
- Data Quality: AI and ML algorithms are driven by the quality of data fed into them. Poor quality or erroneous data can lead to misleading lineage results. It’s like getting a bad apple in a barrel; the rest may look fine but can spoil quickly.
- Interpretability: Many AI models act as a black box, making it challenging to understand how inputs affect outputs. When dealing with data lineage, this lack of transparency can hinder trust in the system. If stakeholders can’t see how data was manipulated or transformed, skepticism can arise.
- Technical Skills Gap: There’s a widening gap in technical skills. While AI tools can enhance lineage tracking, the lack of qualified personnel who can interpret these models may leave organizations in a tough spot.
Adopting AI and ML in lineage tracking should come with strategic planning and training to harness their full potential effectively, keeping in mind these constraints for a balanced approach.
Integration with Big Data Technologies
Another significant trend is the integration of data lineage with Big Data Technologies. As companies amass huge volumes of structured and unstructured data, understanding the complexities of these ecosystems becomes crucial. Here’s how integrating data lineage with big data technologies can be beneficial:
- Real-time Processing: Big data platforms like Apache Hadoop and Spark enable real-time data processing. With integrated lineage capabilities, organizations can monitor how data changes in real time, giving them immediate insights into data integrity and flow.
- Enhanced Data Governance: By embedding lineage frameworks within these technologies, organizations can enforce data governance policies much more effectively. This allows for swift identification of data quality issues or compliance problems.
- Scalability: Big data technologies are inherently scalable. As data grows, lineage tools need to scale likewise. Proper integration allows organizations to keep pace without significant investment in new tools or resources.
In summary, these trends are reshaping the landscape of data lineage. By embracing the potential of AI and Machine Learning while also focusing on integration with Big Data Technologies, organizations can better track their data's lifecycle and ensure compliance, thereby enhancing overall data governance practices.
Ending
In the complex realm of data governance, the conclusion serves as a crucial anchor, tying together the various threads discussed throughout the article. Understanding data lineage is not just a matter of semantics or technicalities; it has real implications for organizations striving to maintain integrity and compliance in their data management practices. By grasping the importance of data lineage, organizations can proactively address issues of data quality, regulatory requirements, and operational risks.
Summary of Key Points
Data lineage is pivotal in the following areas:
- Enhancing Data Quality: By establishing clear pathways for data flow and transformation, organizations can identify anomalies, errors, or inconsistencies promptly, enhancing trust in their data.
- Regulatory Compliance: With increasing scrutiny from regulators, maintaining data lineage aids in tracking data's origins and transformations, ensuring compliance with rules and standards.
- Risk Management: By understanding where data comes from and how it has changed, businesses can better manage risks associated with inaccurate or misleading data.
The exploration of various models of data lineage—from physical to logical and business lineage—showcases the multi-dimensional nature of data tracking.
The Path Forward
The journey ahead for data lineage is both exciting and challenging. Organizations must look at emerging trends like AI and machine learning to streamline data lineage processes further. Adopting these technologies can lead to more robust systems that automatically track data lineage with minimal human intervention.
Additionally, integrating data lineage practices into existing frameworks can be more effective if all stakeholders are engaged from the get-go. Creating an organizational culture that values data lineage will lead to sustainable practices that adapt to future data governance strategies.
As organizations continue to dive deeper into the digital age, ensuring a clear understanding of data lineage is not just a nicety but a necessity. It lays the groundwork for responsible data usage, fostering an environment where data-driven decision-making thrives.