Interview Data Analysis Methods Explained
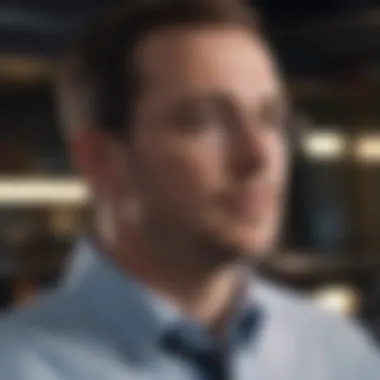
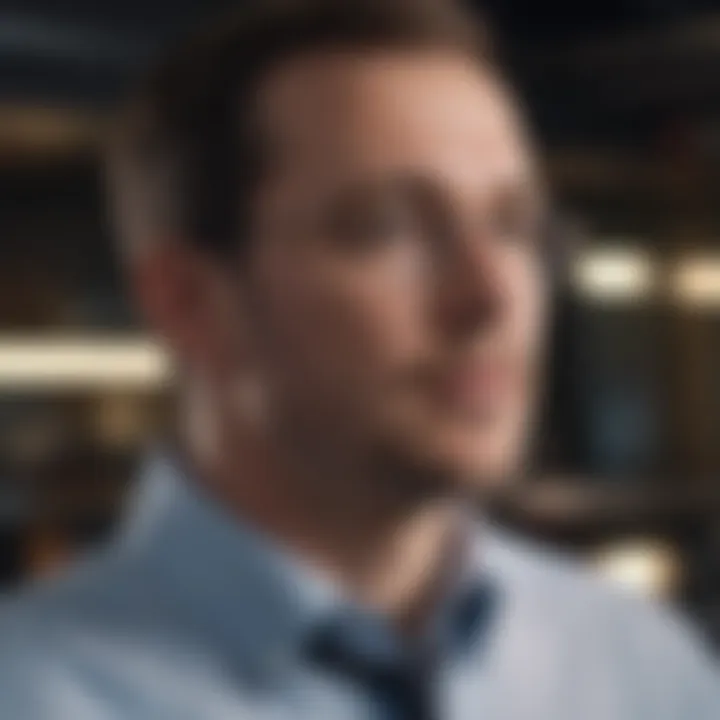
Intro
In the realm of qualitative research, delving into the intricacies of interview data analysis methods is a pursuit of paramount importance. A thorough understanding of these methodologies not only enhances the research quality but also significantly contributes to the reliability of findings. Steering through coding, thematic analysis, and grounded theory, we uncover a tapestry of insights awaiting discovery. Examining these methods sheds light on how researchers can navigate the often murky waters of qualitative data, ensuring that their conclusions are both meaningful and well-founded.
Key Findings
Major Results
A keen analysis of various interview data analysis techniques reveals several key insights:
- Robustness of Coding: Coding serves as the backbone for many qualitative analysis methods. It dissects interview transcripts into manageable parts, allowing for the identification of patterns and themes. This process enhances the clarity of the data and empowers researchers to draw significant conclusions.
- Thematic Depth: Thematic analysis stands out by allowing for a rich, holistic understanding of narratives gathered during interviews. It emphasizes not just what is said but how it resonates with individual experiences and broader societal contexts.
- Grounded Theory’s Empirical Roots: Grounded theory thrives on data-driven exploration. This method allows researchers to construct theories directly from the data collected, which ensures that findings are relevant and nuanced.
Discussion of Findings
Each analysis method has its strengths and weaknesses:
- Coding allows for systematic categorization but may risk oversimplification if not approached thoughtfully.
- Thematic analysis can yield deep insights, yet it can sometimes drift into subjective interpretations if clear criteria are not established.
- Grounded theory offers a flexible framework, yet its reliance on emergent findings may leave researchers grappling with conceptual clarity if not carefully documented.
Understanding the implications of each method is crucial. It shapes the interpretive lens through which researchers understand their data, ultimately influencing the outcomes presented in their studies.
Methodology
Research Design
A well-structured research design is integral for effective qualitative analysis. This entails not merely choosing a method but also considering how it aligns with the research goals. For instance, exploratory studies might benefit from grounded theory, while those aiming to derive specific themes might opt for thematic analysis. Aligning the design with the objectives ensures coherent outcomes and strong foundations for data interpretation.
Data Collection Methods
Choosing the right data collection methods is pivotal as well. Common approaches include:
- Semi-structured Interviews: These strike a balance between guided questioning and open-ended responses, facilitating rich conversations while allowing flexibility.
- Focus Groups: Often yielding a dynamic atmosphere for discussion, focus groups can bring forth varying perspectives, enriching the dataset.
- Recording and Transcription: Ensuring accurate records of interviews is essential for subsequent analysis. Poorly transcribed data can cloud understanding and lead to significant misinterpretations.
Foreword to Interview Data Analysis
Interview data analysis is a pivotal aspect of qualitative research, serving as the foundation for distilling insights from human experiences and narratives. Understanding and interpreting data gathered from interviews can seem daunting at first, but its significance cannot be overstated. This section aims to elucidate the importance of employing structured methodologies when analyzing interview data, ultimately enhancing the richness and validity of findings.
Understanding Qualitative Research
Qualitative research stands apart from quantitative approaches, focusing on the depth of information rather than numerical data. It seeks to provide nuanced insights into participants' thoughts, feelings, and behaviors. By employing methods such as interviews, researchers are able to explore complex subjects that often do not fit neatly into statistical frameworks. This approach is crucial for grasping the intricacies of human experience and obtaining in-depth interpretations of social phenomena.
When delving into qualitative research, it's imperative to recognize its exploratory nature. Unlike a survey that asks closed-ended questions, qualitative interviews invite participants to share their stories freely. This non-prescriptive format encourages a rich dialogue that often reveals unanticipated patterns or themes. It not just broadens understanding but captures the emotional context surrounding the data, which can lead to more meaningful conclusions.
Importance of Interview Methods
The methodology surrounding interviews is crucial since it dictates the effectiveness of data collection and analysis. Interview methods vary significantly, each tailored to specific research objectives. For instance, structured interviews lend themselves to consistency, producing reliable data by using the same questions for every participant. In contrast, semi-structured interviews allow for flexibility, letting researchers probe deeper into certain responses while still following a general guide. Finally, unstructured interviews provide the freedom to explore a participant's narrative without constraints, producing rich data but demanding more skill from the interviewer.
Key Considerations:
- Clarity in Purpose: As a researcher, having a clear purpose helps in choosing which interview type will yield the most relevant data.
- Skill Development: Conducting interviews requires a specific skill set, including active listening and the ability to create a comfortable atmosphere for participants.
- Data Integrity: The method chosen impacts the quality of data collected. A well-defined interview structure can help prevent biases and lead to legitimate findings.
Moreover, being aware of the potential obstacles in interviews is essential. Interviewers must tread carefully around sensitive topics, maintain neutrality, and actively practice reflexivity. The way an interviewer poses questions can unwittingly influence a respondent’s answers, leading to skewed data. Thus, understanding these nuances is key for effective analysis.
"The way we ask questions can shape the stories we hear."
In essence, comprehensive analysis of interview data is not merely about crunching numbers or interpreting words; it is about understanding the stories behind them. By establishing a robust framework for interview methods, a researcher sets the stage for insightful analysis that can greatly advance knowledge in various fields. As this article unfolds, we will further explore the different aspects of interview data analysis, ensuring a holistic understanding that equips researchers, educators, and professionals alike with the tools necessary for impactful qualitative research.
Types of Interviews in Research
Understanding the types of interviews is paramount for researchers delving into qualitative data analysis. Different interview formats serve various research needs, shaping the richness of data collected and influencing the subsequent analysis. In essence, the selected type of interview plays a pivotal role in guiding the focus and depth of inquiry.
Structured Interviews
Structured interviews are often likened to a tightly controlled script in a play. The questions are predetermined, and the interviewer adheres to this script, ensuring consistency across all respondents. This method is particularly valuable for larger sample sizes, where uniformity is key. The structured format lends itself well to quantifiable data, making comparisons easier and enabling researchers to draw statistically relevant conclusions.
However, it can stifle the natural flow of conversation. Participants might feel boxed in, limiting their ability to express unexpected thoughts or ideas. This rigidity often results in data that's comprehensive but may lack depth. For those looking to gather straightforward, comparable data, structured interviews are an effective approach, albeit at the risk of losing some of the nuanced perspectives that participants might offer.
Semi-Structured Interviews
In contrast, semi-structured interviews strike a balance between structure and flexibility. Think of it as a dance with a partner—you have a framework, a series of prepared questions, but there's also room for spontaneity and exploration. This interviewing style allows researchers to prompt deeper discussions, digging into areas the participant may choose to elaborate on.
The beauty of semi-structured interviews lies in their adaptability. If a respondent introduces an intriguing concept, the interviewer can pivot and follow that line of questioning, enriching the data collected. This method not only fosters a more conversational atmosphere but also draws out richer narratives and insights. It’s this interplay that often leads to more profound conclusions, resonating strongly in qualitative research.
Unstructured Interviews
Unstructured interviews take flexibility to another level. With no predetermined questions, this type of interview is akin to a free-flowing chat. The interviewer starts with a broad topic, allowing the participant to guide the conversation. This format is particularly beneficial when exploring complex issues, where structured formats might inadvertently narrow a researcher's focus.
Yet, the freedom inherent in unstructured interviews can lead to challenges. The lack of consistency makes data analysis more intricate, as researchers grapple with varying responses that may not fit neatly into categories. It’s critical for researchers employing this method to be adept at active listening and quick thinking, to ensure they capture essential details in a cohesive manner.
"In qualitative research, the choice of interview type can determine the richness of the data collected and ultimately, the research outcomes."
In summary, the methodology behind interview types—structured, semi-structured, and unstructured—embodies a spectrum of data collection strategies. Each possesses its strengths and weaknesses, demanding careful consideration by researchers to align with their specific objectives. Understanding these nuances ensures that the chosen method not only facilitates effective data collection but also contributes to meaningful insight in qualitative research.
Coding Techniques in Interview Data Analysis
In the realm of qualitative research, coding serves as a crucial step that transforms raw data from interviews into organized, meaningful information. Its significance cannot be overstated; effective coding techniques provide researchers with a pathway to uncover patterns, themes, and insights from complex narratives. With various coding strategies at the researcher's disposal, each method offers different lenses through which to interpret interview data. This section delves into the essential coding techniques, highlighting their respective roles and benefits while also addressing considerations when implementing these methods.
Open Coding
Open coding represents the starting point of the coding process, a stage where the researcher engages directly with the data. This technique involves examining the data line-by-line, identifying concepts and categories that emerge from participants' responses. Think of it as sifting through a treasure chest—each pearl of wisdom can eventually coalesce into themes that illuminate broader contexts.
This method encourages a vigorous exploration of ideas without preconceived notions dictating the process. An advantage of open coding is its adaptability; it enables researchers to remain responsive to unexpected insights, allowing new and relevant topics to arise from the data itself. Here are some common elements associated with open coding:
- Identifying Key Concepts: Key phrases and terms that resonate with the research question often surface during open coding.
- Generating Initial Categories: As concepts solidify, initial categories begin to form, paving the way for deeper analysis.
- Thorough Documentation: Keeping meticulous notes during this phase is paramount, as it serves as the foundation for future analytical stages.
However, it demands careful attention. The danger lies in the possibility of missing out on essential nuances if researchers rush through this phase.
Axial Coding
Once open coding has set the stage, axial coding comes into play, offering a more structured approach to the knowledge unearthed. This technique aims to reassemble the disjointed pieces identified during open coding, connecting categories and subcategories in meaningful ways. It’s akin to reuniting family members after a long separation—everyone has a role, and acknowledging those connections reveals the heart of the narrative.
By focusing on the relationships between various categories, axial coding helps develop a more nuanced understanding of the data. Researchers can distinguish not only what is significant but also how these significant aspects interrelate, leading to richer insights. Elements to consider in axial coding include:
- Defining Relationships: Researchers outline how categories interconnect, which can help in understanding the data's overall structure.
- Refining Categories: This phase may prompt researchers to reassess and adjust the categories created during open coding, cutting some and merging others for clarity.
- Detailed Comparisons: Using axial coding, researchers can compare different categories, revealing the nuances and intersections among them.
While axial coding streamlines the analysis, the careful concern must be given to remaining faithful to the original data, avoiding the temptation to over-interpret relationships unwarrantedly.
Selective Coding
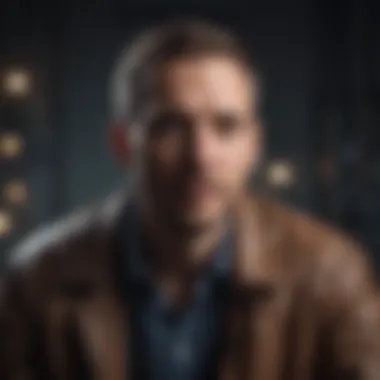
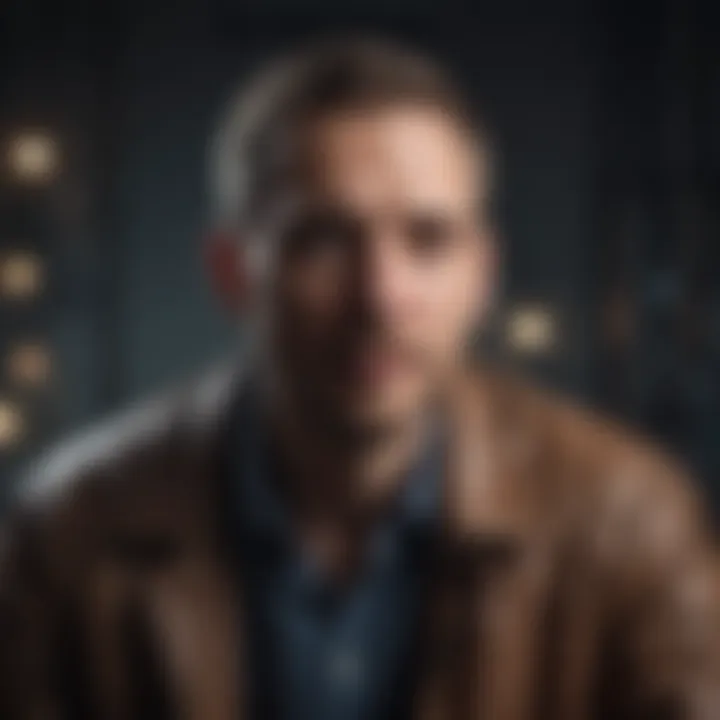
The final phase in coding techniques is selective coding, a process that allows researchers to drill deeper into the core themes identified throughout the previous stages. This method focuses primarily on the heart of the data—selecting central themes that consistently capture the essence of interviews and participants' meanings. Think of it as the fine-tuning stage where the melodies of the narrative come together in harmony.
Researchers begin to formulate and refine a story that captures the overarching narrative from the various themes and categories identified in the previous steps. Elements that enhance selective coding include:
- Focusing on Core Themes: Researchers isolate themes that resonate the most with their research questions and objectives.
- Building a Narrative: Stories evolve from patterns, allowing researchers to narrate findings that are both coherent and compelling.
- Verification against Data: Ensuring that selected themes are grounded in the data remains vital, as this balances narrative construction with empirical evidence.
Selective coding represents the culmination of the analytical process, and here, greater thought is demanded. The researcher’s responsibility is to convey a truthful and comprehensive representation of participants' perspectives, while also addressing any discrepancies or contradictions that may arise.
Using these coding techniques effectively can enhance the richness of interview data analysis, enabling researchers to extract valuable insights that can inform both theory and practice in their fields.
Thematic Analysis
Thematic analysis stands as a pillar in qualitative research, deftly encapsulating the essence of analyzing interview data. It provides a roadmap to uncover patterns, themes, and insights that often lie beneath the surface of spoken narratives. By honing in on key themes, researchers can illuminate vital perspectives that inform broader discourses related to their study area. This section aims to venture through the nooks and crannies of thematic analysis, elucidating its significance, methodologies, and potential drawbacks in the realm of interview data analysis.
Understanding Thematic Analysis
Thematic analysis represents a systematic approach to identifying, analyzing, and reporting themes within qualitative data. At its core, it’s about recognizing recurring patterns that speak to participants’ experiences, perceptions, and motivations. This method is flexible, allowing researchers to derive insights from a range of qualitative data types, making it particularly valuable in interviews, focus groups, or textual analysis. Rather than merely transcribing what was said, thematic analysis asks researchers to engage deeply with the content, promoting an understanding that transcends simple summarization.
A notable strength of thematic analysis is its accessibility. It’s straightforward enough for beginners yet also robust enough to provide rich, nuanced findings for seasoned researchers. Consequently, it serves as an ideal starting point for learners venturing into qualitative analysis, fostering confidence while offering foundational skills that can later be applied to more complex methodologies.
Steps in Thematic Analysis
To embark on thematic analysis, researchers generally follow a series of structured steps:
- Familiarization with the data: It’s imperative to immerse oneself in the data. Reading and re-reading transcripts allows the researcher to become intimately acquainted with the information.
- Generating initial codes: Next, scholars should identify features of the data that are pertinent to the research question. This involves coding segments of data, highlighting important points or ideas.
- Searching for themes: Once codes are established, the next phase is collating them into potential themes. This involves grouping similar codes and identifying overarching concepts that they represent.
- Reviewing themes: It is essential to check whether the themes accurately reflect the data set. At this juncture, researchers may revise, refine, or even discard themes based on how well they encapsulate the data.
- Defining and naming themes: After solidifying themes, succinct definitions should be crafted. Researchers need to clarify what each theme represents, ensuring they encapsulate the important insights without confusion.
- Producing the report: Finally, the researcher compiles a comprehensive report that narrates the analytical journey. This should illustrate the prevalence of themes along with engaging quotes from participants that showcase their voices.
"The essence of thematic analysis lies not just in identifying patterns, but in weaving those threads into a meaningful tapestry of understanding."
Benefits and Challenges of Thematic Analysis
Thematic analysis offers a plethora of benefits, making it a favored choice among qualitative researchers:
- Flexibility: Its adaptable nature allows researchers to adjust the approach based on the goals and context of the study.
- Rich, descriptive data: By capturing expressed themes, researchers can provide in-depth insights that enhance understanding.
- User-friendly: Especially beneficial for newcomers to qualitative research, it allows for a less intimidating entry point into analysis.
However, despite its advantages, thematic analysis is not without challenges:
- Subjectivity: The interpretation of themes can largely depend on the researcher’s perspective, which can introduce bias if not managed carefully.
- Time-consuming: The iterative process, from coding to theme development, can be quite labor-intensive, demanding significant time investment.
- Overemphasis on themes: There's a risk that researchers may focus too narrowly on themes, potentially downplaying alternative interpretations or nuances in the data.
In summary, thematic analysis serves as a crucial tool in qualitatively analyzing interview data, facilitating a rigorous examination of individual experiences and viewpoints. Nevertheless, researchers must navigate its intricacies with care, balancing the rich insights gained with an awareness of subjective influences in interpretation.
Grounded Theory Approach
Grounded theory is a powerful methodology that is often employed in qualitative research, particularly in the analysis of interview data. This approach is uniquely designed to develop theories based on the data itself, rather than testing existing theoretical frameworks. It helps researchers make sense of complex social phenomena by allowing themes and patterns to emerge organically from the information gathered. The significance of grounded theory cannot be overstated, as it promotes a deeper understanding of the subject matter that is directly aligned with individuals' experiences and perspectives.
Grounded theory's iterative process also encourages a level of reflexivity that can lead to more nuanced conclusions. This is particularly important in the field of qualitative research, where the subjective nature of data collection and analysis often plays a crucial role. Researchers benefit from this approach by establishing a framework that is not only rooted in data but also highly adaptable, which is essential in a constantly evolving landscape of social science inquiry.
Conceptual Framework of Grounded Theory
At its core, grounded theory is about building a conceptual framework that is firmly anchored in empirical data. This method starts with data collection, often through interviews, and continues through coding and analysis, creating a cycle where each stage informs the next.
In grounded theory, you follow a structured approach that includes:
- Initial Data Collection: Gathering qualitative data via interviews, observations, or surveys.
- Coding: This involves open coding initially, where you break down data into discrete parts, followed by axial coding, which helps in identifying relationships among the codes. Finally, selective coding allows you to hone in on the core categories.
- Development of Theories: The ultimate goal is to generate theories that give insight into the phenomena represented in your data.
This framework provides the flexibility to adapt as new data is collected, ensuring that the analysis remains relevant and grounded in the actual experiences of participants.
Methods of Data Collection and Analysis
When adopting a grounded theory approach, selecting appropriate methods for data collection and analysis is critical for achieving its intended purpose.
Data Collection Methods:
- Interviews: Semi-structured or unstructured interviews allow for deep exploration of participants' experiences, leading to richer datasets.
- Focus Groups: This method brings together multiple individuals, which can stimulate discussion and reveal diverse perspectives, adding depth to the data.
- Observations: Field observations can complement interview data, shedding light on the contextual factors influencing the subjects of study.
Once the data is collected, analysis unfolds through a series of iterative steps:
- Initial Coding: This step involves breaking down the data into manageable pieces, tagging significant segments.
- Focused Coding: Here, the researcher identifies the most frequent and significant codes and begins to construct categories.
- Memo Writing: Throughout the process, writing memos helps in documenting thoughts, insights, and emerging patterns, which aids in the analysis.
Overall, the grounded theory approach stands out for its dynamic interplay between data collection and analysis, allowing for the development of theories that are rigorously derived from the data itself. In a world where the contexts of social behavior frequently shift, this method equips researchers with a responsive and insightful approach to understanding interview data.
Content Analysis
Content analysis stands as a pivotal methodology in the field of qualitative research, especially when it involves dissecting interview data. This method allows researchers to transform unstructured data into meaningful insights, thus enabling a deeper understanding of human behavior and social phenomena. Through the lens of content analysis, one can identify patterns, themes, and sentiments that emerge from the conversations held during interviews.
The utility of content analysis extends beyond mere data examination; it equips researchers with the ability to categorize and quantify information, thereby offering a dual lens of qualitative richness and quantitative clarity. This approach to data interpretation is not just a good-to-have in research but rather an essential compass guiding scholars to navigate the complex landscape of human experience.
Some key aspects of content analysis include:
- Clarity and Structure: By defining key themes, categories, and subcategories, researchers can create a structured view of complex qualitative data.
- Flexibility: Content analysis can be applied across various types of data including text, images, and audio, making it quite versatile in multiple research contexts.
- Replicability: The systematic nature of this approach enhances the reliability and validity of findings, making it easier for future researchers to interpret the same data with similar frameworks.
In the context of this article, examining content analysis provides crucial insights into how researchers can extract valuable information from interviews, leading to enhanced understanding and innovations in their respective fields.
Defining Content Analysis
Content analysis can be understood as a systematic approach to analyzing communication content. This can range from interviews to publications, social media posts, and beyond. At its core, content analysis seeks to quantify and analyze the presence of certain words, themes, or concepts within the data. Erving Goffman once stated that we often define ourselves through our narratives, and through content analysis, we can unveil these narratives in a structured manner.
To perform content analysis, researchers typically follow these basic steps:
- Selection of Material: Identifying the data corpus, be it from interview transcripts or other sources.
- Development of Categories: Creating a coding scheme based on research questions and objectives.
- Coding Process: Segmenting the text and assigning it to corresponding categories.
- Analysis of Overall Patterns: Interpreting the patterns that emerge based on the coded data.
This method serves to bridge the gap between raw data and actionable insights, shedding light on how certain ideas, behaviors, and sentiments are expressed through interviews.
Quantitative vs. Qualitative Content Analysis
When diving into content analysis, it’s paramount to distinguish between two frameworks: quantitative and qualitative content analysis. While both approaches aim to unveil insights from the data, they employ contrasting methods and yield different outcomes.
- Quantitative Content Analysis focuses on counting and measuring data. This method seeks to quantify the presence of specific words, phrases, or concepts. It often includes:
- Qualitative Content Analysis, on the other hand, emphasizes understanding the deeper meaning behind the content. It involves:
- Statistical analysis of the findings to create graphs or charts.
- Objective measures that subject the data to replicable codifying rules.
- Identifying underlying themes or patterns in the data that may not be immediately visible through numbers alone.
- Providing context to the data by interpreting the meanings and implications behind certain expressions or narratives.
Both methodologies offer valuable insights. They can even be employed together for a richer analysis, combining numerical data's objectivity with qualitative depth.
"The holistic approach is key; sometimes numbers alone can’t tell the full story."
By comprehensively understanding both quantitative and qualitative approaches, researchers are better equipped to select the method that aligns best with their research goals and the nuances of the data they are analyzing.
Narrative Analysis
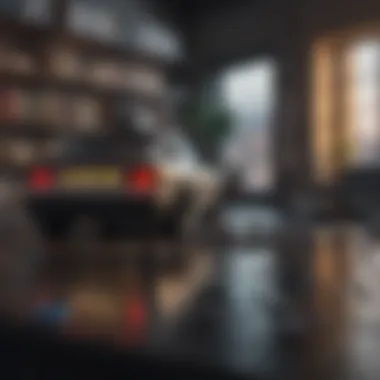
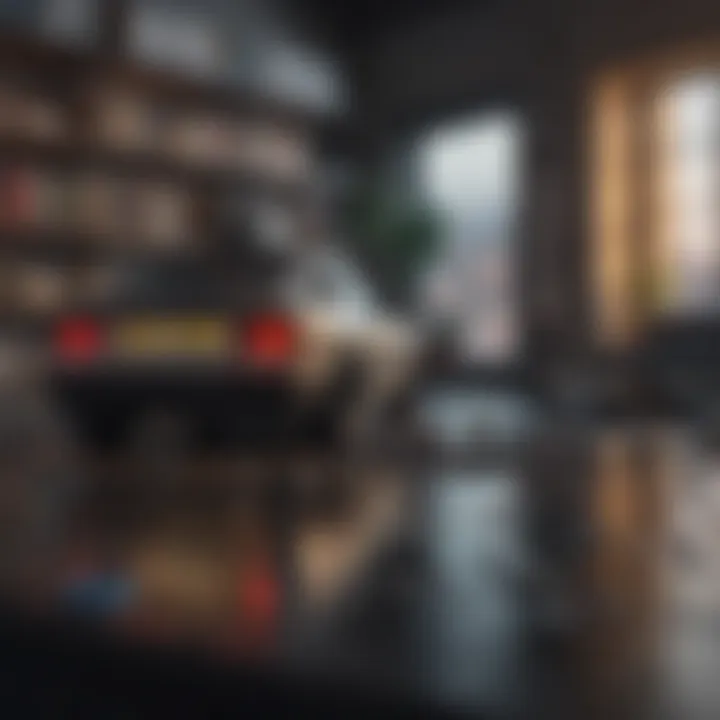
Narrative analysis serves as a crucial component in the realm of interview data analysis, providing researchers with the tools needed to understand and interpret the rich stories shared by participants. This approach does not merely catalog individual experiences but seeks to explore the deeper meanings and implications behind those narratives. By focusing on how people construct meaning through their stories, researchers can unearth layers of context often overlooked by other methodologies.
In essence, narrative analysis enables a layered understanding of data. It allows for a differentiated exploration of themes, plots, and characters within the interview content, much like a well-crafted novel. Researchers can analyze not just what is said, but how it is said - the emotions expressed, the language utilized, and the overarching narrative structures that participants impose on their experiences. The importance of this method lies in its ability to humanize data, transforming raw information into compelling stories that resonate with broader audiences.
Exploring Narrative Analysis
Diving deeper into the mechanics of narrative analysis, it becomes essential to recognize its origins and intentions. Rooted in disciplines such as sociology, anthropology, and psychology, this method prioritizes the subjective experience. Here’s a breakdown of some fundamental aspects of narrative analysis:
- Chronology: The sequence of events plays a pivotal role in shaping narratives. Researchers analyze how participants construct time within their stories, whether they emphasize certain events over others.
- Characterization: Central figures within a narrative, whether they be individuals or institutions, help shape the perspectives offered. Understanding the representation of these characters can reveal biases or broader societal narratives.
- Themes: Key motifs often emerge from narratives, providing insight into shared cultural or psychological elements present among various participants.
Through these lenses, researchers can effectively interpret complex data. They can distill insights that might otherwise remain obscured - emerging patterns can inform not only future research but also inform theoretical frameworks.
Application of Narrative Analysis Methods
Applying narrative analysis methods requires a systematic approach. One popular technique involves the selection of specific narratives from interviews and conducting a thematic analysis of the stories. Here’s a structured outline on how to effectively implement narrative analysis:
- Data Collection: Conduct interviews focusing on open-ended questions that allow participants to tell their stories freely. This maximizes narrative richness.
- Transcription: Transcribe the interviews verbatim. Pay close attention to the nuances in speech, pauses, and emotional punctuation, as these can offer deeper insights.
- Identification of Key Narratives: Identify significant stories within the broader dataset. This might involve selecting narratives that showcase extremes of experience, or those that highlight unique perspectives.
- Analysis: Engage with these narratives through coding themes, exploring character development, and mapping out plots. Use tools like mind maps or flowcharts to visualize connections between different narratives.
- Interpretation: Finally, synthesize the results. Reflect on how these narratives contribute to existing literature and what new insights they bring to the table.
"Narrative analysis is not just about collecting stories; it is an invitation to walk alongside individuals in their lived experiences."
In summary, narrative analysis emerges as a pivotal strategy within qualitative research, enriching the fabric of interview data by transforming it into potent stories. Its applications are varied, but the core aim remains the same: to capture the essence of human experience through the lens of narrative.
Pragmatic Approaches in Data Analysis
In the realm of qualitative research, pragmatic approaches offer a flexible framework that transcends the boundaries of conventional methodologies. They bridge the gap between qualitative and quantitative data, enriching the analysis by allowing researchers to draw from diverse pools of evidence. This section explores the significance of pragmatic approaches in data analysis, focusing specifically on how they enable effective integration of different data forms, respond to research questions more comprehensively, and enrich the overall understanding of the phenomena being studied.
Combining Qualitative and Quantitative Data
Combining qualitative and quantitative data is not just a trend; it's becoming essential for capturing the complexities of modern research. Pragmatic approaches, when applied properly, can yield a richer narrative that neither method could provide in isolation.
Benefits of this integration include:
- Holistic Understanding: By employing both qualitative insights and quantitative metrics, researchers can gain a deeper understanding of the subject matter. For example, a study on customer satisfaction can leverage survey data (quantitative) alongside face-to-face interviews (qualitative), painting a fuller picture of customer experiences and expectations.
- Enhanced Validity: Using mixed methods can bolster the credibility of findings. Qualitative data helps explain the 'why' behind the numbers, supporting and contextualizing quantitative results. A common practice might involve conducting focus groups to explore responses from a structured survey, which can illuminate underlying feelings that statistics alone cannot convey.
- Flexible Methodology: When researchers adopt a pragmatic approach, they are not confined to rigid methods. This flexibility allows for adaptation based on specific research needs, making it easier to pivot as new insights emerge during the analysis.
Moreover, researchers must be mindful of certain considerations when combining data types:
- Cohesive Framework: Developing a coherent analytical framework is vital. Mismatched methodologies can lead to confusion and disjointed findings. A clear structure helps ensure that each data type contributes meaningfully to the overall analysis.
- Data Triage: Deciding which data sets hold more weight necessitates careful evaluation. Researchers must continually ask questions about the relevance and applicability of each data type to their specific investigation.
In summary, pragmatic approaches to data analysis, particularly through the combination of qualitative and quantitative data, significantly enhance a researcher's ability to interpret complex phenomena. This integration not only enriches the data analysis process but also equips researchers with insights that might otherwise remain undiscovered.
"Utilizing mixed methods empowers researchers to harness the strengths of both qualitative and quantitative research, enabling them to engage more fully with their subject matter." - Anonymous
By bearing these aspects in mind, researchers can effectively navigate the nuanced landscape of qualitative research while presenting their findings in a manner that is both insightful and accessible.
For further reading on qualitative and mixed-method approaches, consider exploring resources such as Wikipedia as well as popular discussions on platforms like Reddit.
This is where the magic happens in qualitative research; through pragmatic approaches, the true depth of human experience can be illuminated.
Ethical Considerations in Interview Data Analysis
When tackling the subject of interview data analysis, it’s crucial to navigate the ethical landscape with care. The analysis of interview data involves direct engagement with individuals, their thoughts, experiences, and vulnerabilities. Hence, the ethical considerations that arise are not merely an afterthought but a foundational pillar of responsible research. Good practices in ethics not only enhance the credibility of the research but also protect the participants involved, creating a fair balance between inquiry and compassion.
In the realm of qualitative research, ethical considerations can significantly shape the insights derived from interviews. By ensuring ethical integrity, researchers foster trust, encouraging openness among participants. This trust is vital as it lays the groundwork for richer, more nuanced data. The primary ethical concerns often boil down to informed consent and the maintenance of privacy — two themes that will be explored more deeply in the following sections.
Furthermore, ethical deliberations are not restricted to the initial stages of data gathering; they extend through the entire research process, influencing analysis, interpretation, and dissemination. For instance, the reflexivity in research underpins the ethical stance of the researcher. In other words, being aware of one’s own biases and perspectives can profoundly impact the authenticity of the data interpretation. As we explore informed consent and privacy, as well as reflexivity, remember that being ethical in research is tantamount to being respectful toward the participants and their narratives.
"Ethics is not just a set of rules; it’s about building a relationship based on trust and respect."
Informed Consent and Privacy
Obtaining informed consent serves as a cornerstone in any ethical research framework. This process involves ensuring that participants fully understand what their participation entails. They should be aware of how their data will be utilized, who will have access to it, and potential risks involved. It's paramount that consent is not just a formality; it should be a genuine agreement made without any coercion. Leaving no room for ambiguity allows participants to feel more in control of their narratives.
In practice, informed consent can take various forms. While written consent is widely accepted, verbal consent may be appropriate in some contexts, particularly in cultures where traditional practices favor informal agreements. Researchers need to assess the situation and adapt accordingly, always prioritizing clarity and thoroughness.
Privacy concerns intertwine closely with informed consent. Researchers must ensure that participants' identities remain confidential. This can involve anonymizing data, coding responses, or utilizing pseudonyms to shield individual identities. Keeping participant information secure extends beyond data collection; researchers should also consider data storage and eventual publication of their findings. Here, ethical dilemmas may arise regarding what to share and what to withhold. A balance must be struck, ensuring transparency while safeguarding participants.
Reflexivity in Research
Reflexivity is about the researcher’s ability to reflect on their role in the research process, encompassing their personal biases, assumptions, and interactions with participants. This aspect is fundamental to maintaining ethical standards in qualitative research, as it acknowledges that the researcher is not merely an observer but also a participant in the construction of knowledge.
Self-awareness during the research process allows for a nuanced understanding of how one’s own background, beliefs, and experiences can distort interpretation. For instance, two researchers might analyze the same interview data but come to different conclusions based on their individual frames of reference. Engaging in reflexivity helps to unveil these biases and encourages researchers to consider alternative interpretations, ultimately enriching the research outcome.
Being reflexive also calls for maintaining an ongoing dialogue with participants. Rather than treating the interview as a one-off event, researchers can share preliminary findings, asking participants for their thoughts and reflections. This practice not only validates participants’ voices but also highlights the dynamic relationship between researcher and subject.
Best Practices in Interview Data Analysis
Analyzing interview data isn't just about collecting thoughts and opinions; it's about structuring those insights methodically. Best practices in this domain serve as a compass, guiding researchers through the often intricate process of making sense of qualitative data. Why is it imperative? Without a solid framework, valuable insights could slip through the cracks. Think about it; when navigating a maze, having a map keeps you on the right path. Similarly, these best practices provide clarity and enhance the quality of your analysis.
Effective Analytical Frameworks
Employing an effective analytical framework provides the skeleton upon which the flesh of your analysis can be built. It’s like having a well-planned recipe before you start cooking. The framework should be flexible enough to adapt to various types of data, yet structured to ensure that every element is addressed diligently.
- Define your Goals: Set clear objectives for what you wish to achieve through the analysis. Are you looking to identify patterns, or do you want to derive specific themes?
- Select the Right Framework: Common frameworks like thematic analysis or grounded theory each have unique strengths. Match your choice to your research questions and data type.
- Iterative Process: Treat your analysis as a dynamic, ongoing process. Revisiting earlier steps can unearth additional insights; the hypothesis may evolve as new data emerges.
An example could be a researcher using thematic analysis in a study focusing on students' academic stress. After establishing clear goals and identifying themes such as 'peer pressure' or 'time management', they can continuously revisit their findings to ensure that all relevant aspects are integrated into the final analysis.
Data Management Techniques
Data management is akin to keeping your house tidy; without organization, you may find yourself lost among stacks of papers. Here are some techniques that ensure your data is handled effectively:
- Organize Early: From the start, categorize your data. This could mean creating folders on your computer or using note-taking software like Evernote or OneNote. It will save time during the analysis phase.
- Use Version Control: Keep track of changes with a version control system. This allows you to backtrack if necessary, avoiding the pitfall of losing crucial insights amid revisions.
- Document Everything: Maintain a record of your processes, decisions, and iterations. This is vital not just for your understanding, but it also enhances transparency if others need to review your work.
Documenting your analysis choices adds a layer of rigor and trustworthiness. It’s like building an academic footprint that others can follow.
By integrating these practices into your interview data analysis workflow, you not only empower yourself as a researcher but also contribute to the field by ensuring that your findings are backed by rigorous methodologies.
Software Tools for Data Analysis
In the realm of qualitative research, software tools for data analysis have become more than just a luxury; they are a necessity. These tools facilitate the organization, coding, and interpretation of interview data, allowing researchers to navigate complex datasets with ease. By utilizing software, users can streamline their workflows, promote efficiency, and ultimately enhance the rigor of their analyses. As the landscape of qualitative research evolves—fuelled by an increasing amount of data—embracing the right tools is crucial for anyone involved in this field.
Several key benefits stand out when discussing software tools for data analysis:
- Enhanced Efficiency: Manual coding of interview transcripts can be labor-intensive and time-consuming. Software can automate many of these tasks, giving researchers more time to focus on interpretation and insight.
- Improved Accuracy: By using these tools, it's easier to maintain consistency in coding, reducing human error. This is especially important in qualitative analysis, where nuances can be easily overlooked.
- Collaboration Capabilities: Many software options offer cloud-based solutions, thereby allowing teams to work collaboratively, even from different locations. This is crucial in interdisciplinary projects where diverse expertise is valuable.
- Rich Visualization Options: Visualization tools within these software programs can help in presenting findings in a compelling manner. Graphic representations of data can simplify complex insights, making it easier for stakeholders to grasp important concepts.
"Choosing the right software can be the difference between insightful analysis and a frustrating experience that leads to missed opportunities for understanding."
However, there are important considerations to keep in mind when selecting software tools. Some tools may come with steep learning curves or might not cater to specific qualitative methodologies. Research teams should weigh factors such as user-friendliness, compatibility with existing data structures, and the specific analytical needs of their project.
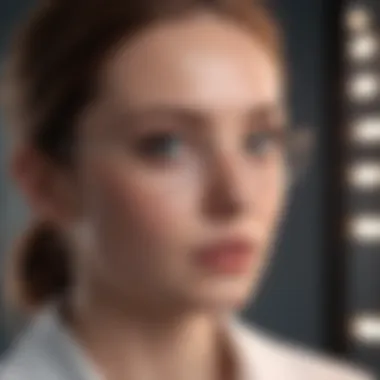
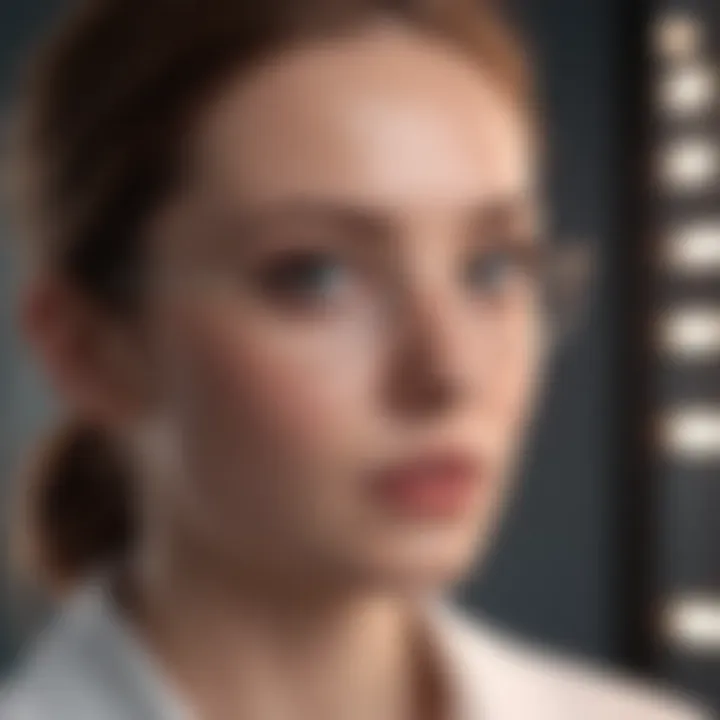
Intro to Qualitative Data Analysis Software
Qualitative data analysis software serves an essential role in transforming raw data into meaningful insights. Programs such as NVivo, MAXQDA, and ATLAS.ti allow researchers to process vast amounts of qualitative data while maintaining the depth of investigation that qualitative approaches are known for. This section sheds light on the critical functionalities of these tools:
- Coding Features: These programs often enable users to create and manage codes with categories, offering the capacity to connect themes across data sets.
- Memo Writing: Researchers can jot down thoughts and reflections as they analyze data, promoting a deeper engagement with the material.
- Search Capabilities: The ability to search transcripts quickly can reveal patterns or themes that aren’t immediately obvious, enhancing the quality of the analysis.
Comparative Analysis of Popular Software
When choosing between different qualitative data analysis software, a comparative approach can offer clarity. Each software package has its own strengths, and understanding these can help in making an informed choice:
- NVivo: This software is highly regarded for its ability to manage large volumes of data. It includes a variety of coding and visualization tools, making it suitable for complex qualitative projects.
- MAXQDA: Known for its user-friendly interface, MAXQDA also offers robust tools for mixed-methods analysis, making it versatile for qualitative and quantitative data integration.
- ATLAS.ti: This option focuses on creating a network of relationships between codes, which can be particularly useful in developing grounded theory. Furthermore, it emphasizes the use of visual representations.
When making a choice, researchers should consider their specific project demands and personal comfort with the software. It's often a good idea to trial a couple of options before settling on a singular software, ensuring that it meets both analytical needs and user experience preferences.
Interpreting Interview Data
Interpreting interview data is a pivotal aspect of qualitative research. After the lengthy, often intricate, process of collecting data through interviews, researchers find themselves at a critical junction. This phase not only involves making sense of what has been said but also requires synthesizing insights in a way that informs further research, practice, or policy. The richness of interview data comes with an inherent responsibility; hence, careful interpretation is key to unlocking its potential.
The importance of this stage can be summed up as follows:
- Building Meaning: Understanding the nuances behind responses helps in capturing the essence of participant experiences.
- Context Matters: Contextual factors often influence how data is understood and should shape interpretation.
- Avoiding Misinterpretation: Without a solid interpretation strategy, researchers can easily misrepresent findings.
Taking the time to interpret effectively can lead to better insights, aiding not only the immediate study but also contributing to broader fields of knowledge.
Strategies for Interpretation
Several strategies can be employed when interpreting interview data. Here are some that have proven useful:
- Thick Description: Strive for depth in reporting. This means providing rich detail about the context, setting, and background of participants’ responses.
- Triangulation: Use multiple data sources or methods to confirm findings. This helps to ensure that the insights drawn are robust and less likely to be biased.
- Member Checking: Returning to participants for validation of findings can clarify discrepancies and confirm the authenticity of interpretations, ultimately enhancing the credibility of the research.
- Thematic Approach: Identify recurring themes or patterns within the data. This can help in structuring the findings in a way that unearths underlying narratives.
- Narrative Techniques: Employ storytelling elements to present data in a more relatable format, allowing readers to connect on a human level with the findings.
- Example: Instead of stating respondents were "disappointed" with a service, would it not be more compelling to convey the story behind their disappointment?
- Strategies could include comparing interview data with observational data or archival materials.
"The role of context and lived experiences cannot be overstated in qualitative research; they shape the interpretation process significantly."
Linking Analysis to Theory
Connecting your analysis back to existing theories enriches the interpretation of interview data. When researchers bridge their findings with theoretical frameworks, it opens the door for broader implications and relevance.
Here’s how linking analysis to theory can enhance understanding:
- Establish Frameworks: By clearly articulating how findings relate to theoretical concepts, researchers can lend explanatory power to their results. This moves beyond mere description to offer critical insights into the phenomena under study.
- Identify Gaps: Linking interpretation to theory often helps in identifying gaps in existing research or areas requiring further study, allowing for a dynamic engagement with the literature.
- Encourage Discussion: The relevance of findings can stimulate debate within the academic community. An analysis grounded in theory resonates more with scholars and practitioners alike, prompting a dialogue that advances understanding in the field.
- Develop Practical Implications: Theories can offer recommendations for practice based on research findings, enhancing the contribution of qualitative studies to real-world applications.
- For instance, if interviewing participants about their motivation in the workplace, connecting it to Maslow's Hierarchy of Needs can ground findings in a powerful theoretical context.
Case Studies on Interview Data Analysis
Understanding case studies in the context of interview data analysis offers a unique lens for diving into practical applications of qualitative research methods. They serve as excellent real-world examples that underpin the theoretical frameworks discussed in previous sections. Case studies illuminate not only the effectiveness of various analysis techniques but also their limitations.
By exploring these focused investigations, researchers, students, and educators can witness how theoretical principles translate into actual practice and outcomes.
Highlighting Successful Applications
Many successful applications of interview data analysis stem from well-documented case studies. For instance, a research project investigating community health issues may rely heavily on semi-structured interviews with participants to grasp nuanced perspectives. Consider the following successful instances:
- Health Studies: In 2019, researchers focusing on mental health utilized semi-structured interviews in a case study aiming to explore the experiences of individuals in therapy. Their thematic analysis revealed recurring themes around stigma and personal growth.
- Education Research: A university's case study analyzed teachers’ experiences during the shift to online learning. By conducting unstructured interviews, the study garnered candid insights that led to improved training programs for faculty. The flexibility of the interview format facilitated rich detail in participants’ responses, offering depth missing in rigid surveys.
These cases highlight how employing appropriate interview techniques and analytical frameworks can significantly influence the richness of the findings. Furthermore, they offer a strong remind for researchers about the importance of adaptability in their methods.
Lessons Learned from Different Fields
Learning from diverse fields through case studies provides profound insights not just into methodologies but also into contextual challenges faced by researchers. With several studies across various domains, certain lessons emerge:
- Adapting Techniques: In sociology, case studies emphasize the adaptability of interview methods to fit cultural contexts. For example, in a study on community dynamics, researchers quickly learned that language and context significantly shaped participants' responses. This adaptability also applies to coding and thematic analysis, which should evolve in tandem with the interview processes.
- Reflexivity and Ethics: In psychology, case studies often stress the importance of reflexivity during analysis. Researchers in this field learned that their personal biases could inadvertently shape interpretations of interview data. Maintaining an ongoing dialogue about ethical considerations bolstered the integrity of findings.
- Interdisciplinary Insights: From business research, professionals applied narrative analysis to comprehensively understand consumer behavior. By detailing users' experiences with products, they found that storytelling offered invaluable insights into emotional connections with brands.
Through these diverse examples, we see how insights gained from each discipline not only enhance methodologies but also broaden perspectives in interview data analysis. Therefore, it is imperative to engage with case studies actively and extract lessons that can be applied across contexts.
"Case studies are not just stories; they're rich narratives that offer profound lessons in methodology, ethics, and adaptability."
In summary, case studies on interview data analysis serve as powerful instruments for illustrating the practicalities of qualitative research, reinforcing fundamental research principles and encouraging a reflective approach throughout the analytical process.
Future Directions in Interview Data Analysis
As the landscape of research continues to evolve, the way we analyze interview data must also transform. Future directions in interview data analysis promise not only to enhance the rigor of qualitative research but also to facilitate richer insights. With technological advancements and shifts in methodologies, professionals are presented with opportunities to refine their analytical approaches. This section delves into these emerging avenues and their significance in shaping qualitative research trajectories.
Emerging Trends and Innovations
In recent years, several trends have started gaining traction that can significantly impact how researchers engage with interview data.
- Increased Use of Mixed Methods: Researchers are now more inclined to combine qualitative and quantitative approaches. This hybrid strategy allows for a more comprehensive understanding, enhancing the credibility of findings. For instance, integrating demographic surveys with in-depth interviews can create a fuller picture of the subject matter being studied.
- Visual Data Representation: As researchers strive for ways to present data more engagingly, the use of visual aids has surged. Infographics, thematic maps, and video analysis tools are being utilized to depict complex relationships and findings. This innovation promotes higher accessibility and enhances communicative power.
- Focus on Participant Voices: There's a growing recognition of the need to prioritize participants' perspectives more directly within the analytical process. Techniques like participatory analysis, where participants are involved in the interpretation of findings, are being developed. This ensures that their experiences are accurately reflected in the analysis.
These trends illustrate a shift toward a more comprehensive, inclusive, and visually engaging approach to interview data analysis.
Integrating AI and Data Analysis
The integration of artificial intelligence in qualitative research opens a plethora of opportunities for interview data analysis. AI can enhance efficiency, accuracy, and even the depth of insights derived from qualitative data.
- Automated Coding Systems: Through natural language processing, AI can assist in coding interview transcripts. By automatically identifying themes and patterns, researchers can save considerable time. This allows them to focus on deeper analyses rather than mundane, repetitive tasks.
- Enhanced Analytical Tools: Sophisticated analytic software backed by AI can facilitate nuanced understanding of emotional tones and sentiments within interview transcripts. This level of analysis provides richer insights than traditional methods, allowing researchers to discover subtler nuances in participant responses.
- Predictive Analytics: By leveraging AI algorithms, researchers can analyze vast amounts of qualitative data to predict trends or behaviors. This predictive capability can be invaluable for driving strategic decisions in various fields, from healthcare policy to marketing strategies.
Integrating AI into interview data analysis not only empowers researchers with advanced tools but also pushes the boundaries of traditional qualitative research, fostering an environment ripe for innovation.
"The future of interview data analysis lies at the intersection of technology and human insight, creating a dynamic landscape for inquiry."
End
As we look ahead, it's clear that the ways we analyze interview data will continue to evolve. Emerging trends and AI integration not only enhance the efficiency of research but also deepen the richness of insights. By adapting to these changes, researchers can ensure their work stays relevant and impactful in an ever-changing academic landscape.
Finale
In the final stretch of our discussion on the various methods of analyzing interview data, it’s vital to stitch together the key threads that have been woven throughout this comprehensive overview. The importance of this topic underscores the intricate relationship between effective data analysis and the advancement of qualitative research. When researchers dissect interview data carefully, they illuminate the richness of human experience, often revealing insights that are not immediately apparent.
Summarizing Key Insights
To encapsulate, here are some of the cornerstones from our earlier sections:
- Variety of Techniques: Each method, be it thematic analysis or grounded theory, brings its unique flavor to the table, allowing researchers to approach data with different lenses.
- Ethical Dimensions: The ethical considerations, such as informed consent and participant confidentiality, are paramount. These elements ensure that research respects the rights and dignity of individuals involved.
- Impact of Technology: The integration of software tools for data analysis not only streamlines the processing phase but also enhances the accuracy of qualitative interpretations.
This collection of insights provides a solid foundation for any researcher or professional venturing into the realm of interview data analysis. Understanding these methods equips you with the necessary know-how to make informed decisions tailored to specific research questions or contexts.
Final Thoughts on Interview Data Analysis
As we wrap up, it’s clear that interview data analysis is not merely a box to check in the research process—it's a vital step in understanding complex sociocultural phenomena. The future of qualitative research looks promising, especially with the emergence of new analytical techniques and digital tools that continually reshape our approaches. Researchers should embrace these advancements while remaining grounded in ethical considerations that govern our research practices.
Remember, taking a nuanced approach towards interview data analysis not only enriches the research narrative but ultimately contributes to a more robust body of knowledge within the academic community. As you embark on your qualitative journeys, may your analyses be insightful, responsible, and transformative.