Exploring Pharmacokinetic-Pharmacodynamic Models
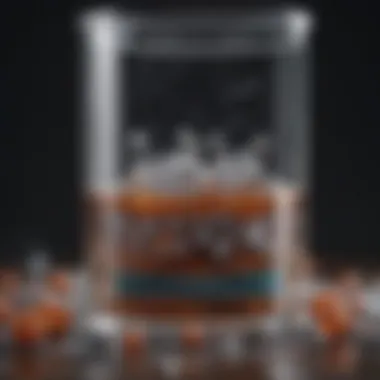
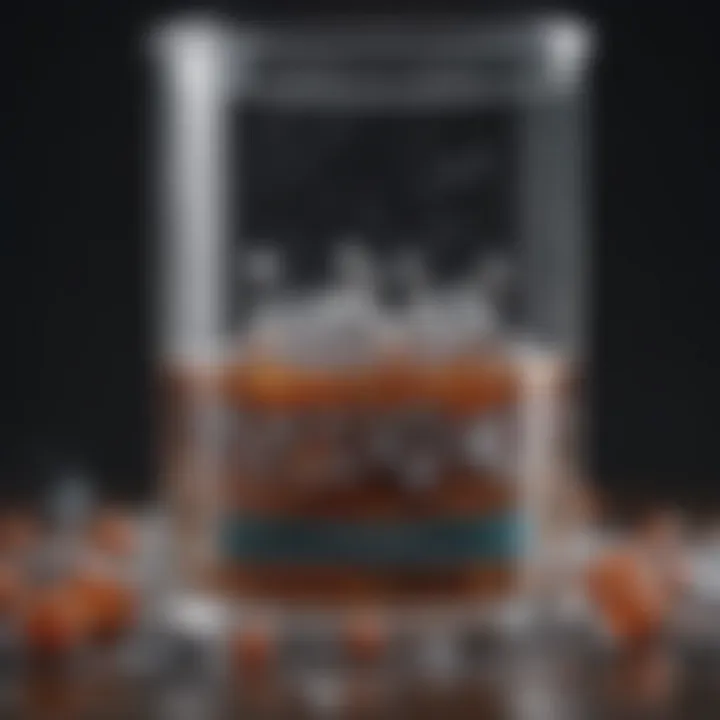
Intro
Pharmacokinetic-pharmacodynamic models play a crucial role in understanding how drugs behave in the body and their effects on biological systems. These models bridge pharmacokinetics, which focuses on drug absorption, distribution, metabolism, and excretion, with pharmacodynamics, which studies the biochemical and physiological effects of drugs. Understanding these models is essential for pharmacologists, clinicians, and researchers aiming to optimize drug therapy and enhance patient outcomes.
The landscape of drug development relies heavily on these models. They enable researchers to predict how different drugs will interact with the body and provide insights into the appropriate dosages required to achieve desired therapeutic effects. However, the complexity of biological systems poses significant challenges in accurately modeling these interactions. The incorporation of advanced statistical approaches and computational tools is transforming the field, allowing for more sophisticated models that better capture the nuances of drug action.
Throughout this article, we will examine foundational concepts of pharmacokinetic-pharmacodynamic models, the mathematical frameworks that support these models, and the implications for clinical practice. We will also explore the challenges researchers face in this domain, and consider future directions and innovations that may shape the field.
Key Findings
Major Results
The investigation into pharmacokinetic-pharmacodynamic models reveals several key insights:
- Importance of Accurate Modeling: Accurate representation of drug behavior is vital for effective treatment regimens. Models aid in predicting the onset, intensity, and duration of drug effects.
- Clinical Application: These models facilitate personalized medicine by allowing healthcare providers to tailor medications based on individual patient characteristics, thus improving therapeutic outcomes.
- Challenges in Modeling: Variability in patient responses, differences in drug formulations, and ethical considerations during clinical trials impose complexities that need to be resolved.
Discussion of Findings
The findings emphasize that pharmacokinetic-pharmacodynamic models are not merely theoretical constructs but have direct implications in real-world scenarios. Clinicians leverage these models for drug selection and dosing adjustments. However, ongoing refinement is needed to accommodate the dynamic nature of drug action. For instance, factors such as age, weight, genetic polymorphisms, and comorbid conditions can influence pharmacokinetic parameters, complicating the predictive capacity of traditional models.
"Pharmacokinetic-pharmacodynamic models serve as a cornerstone in developing effective therapeutic strategies, influencing clinical decisions and improving patient outcomes."
Methodology
Research Design
Research in the field typically employs quantitative methodologies, often utilizing in vivo and in vitro studies to gather relevant data. These studies help in evaluating how drugs behave over time within biological systems. Challenges in standardizing these methodologies continue to be a significant barrier to optimizing model accuracy.
Data Collection Methods
Data is often collected using sophisticated techniques including:
- Clinical Trials: Phase I-III trials provide direct insights into drug action in human populations, contributing to model refinement.
- Biomarkers Analysis: Monitoring specific biomarkers can give indications of drug efficacy and safety, which are integrated into models.
- Pharmacogenomics: Understanding how genetic factors influence drug metabolism and response can guide model adjustments for better predictive power.
By synthesizing information from various avenues, researchers can enhance their understanding of the drug action, allowing for better predictions and ultimately improved patient care.
Prelims to PK-PD Models
Pharmacokinetic-pharmacodynamic (PK-PD) models serve a critical role in the evaluation and understanding of drug behavior in the human body. These models amalgamate two key areas: pharmacokinetics, which examines how the body affects a drug, and pharmacodynamics, which studies how a drug impacts the body. The interrelation of these areas enables researchers and practitioners to predict the effects of drugs based on exposure levels and their subsequent impacts.
In this article, we will explore the complexities of PK-PD models through a detailed examination of their components and applications. Understanding the fundamental concepts of these models is essential for students, researchers, and professionals aiming to develop and optimize drug therapies.
Defining Pharmacokinetics
Pharmacokinetics involves the processes by which a drug is absorbed, distributed, metabolized, and excreted from the body. Each of these steps is influenced by various factors, including dosage, route of administration, and individual patient characteristics. For instance, the bioavailability of a drug, which denotes the proportion that enters systemic circulation, is crucial for determining its effective concentration in the bloodstream. Understanding these processes helps in predicting the drug's therapeutic levels and potential toxicity.
Key aspects of pharmacokinetics include:
- Absorption: How quickly and efficiently a drug enters the bloodstream.
- Distribution: The dispersion of the drug throughout body fluids and tissues.
- Metabolism: The chemical transformation of substances, mainly occurring in the liver, which can activate or deactivate drugs.
- Excretion: The elimination of drugs or their metabolites, predominantly through urine.
Each process must be carefully analyzed to design effective dosing regimens and to ensure patient safety.
Understanding Pharmacodynamics
Pharmacodynamics, in contrast, focuses on the relationship between drug concentrations at the site of action and the resulting effects on the body. This aspect is more concerned with the mechanisms of action, including how drugs bind to receptors and modulate biological responses. The concept of efficacy and potency is central in this area, emphasizing the drug's ability to produce a desired effect as well as the amount of drug required to achieve that effect.
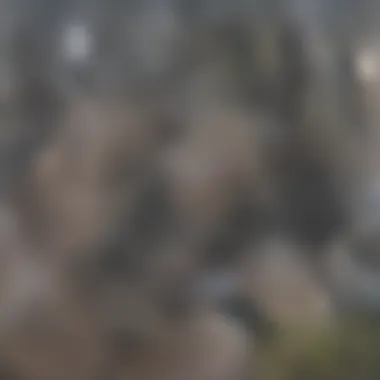
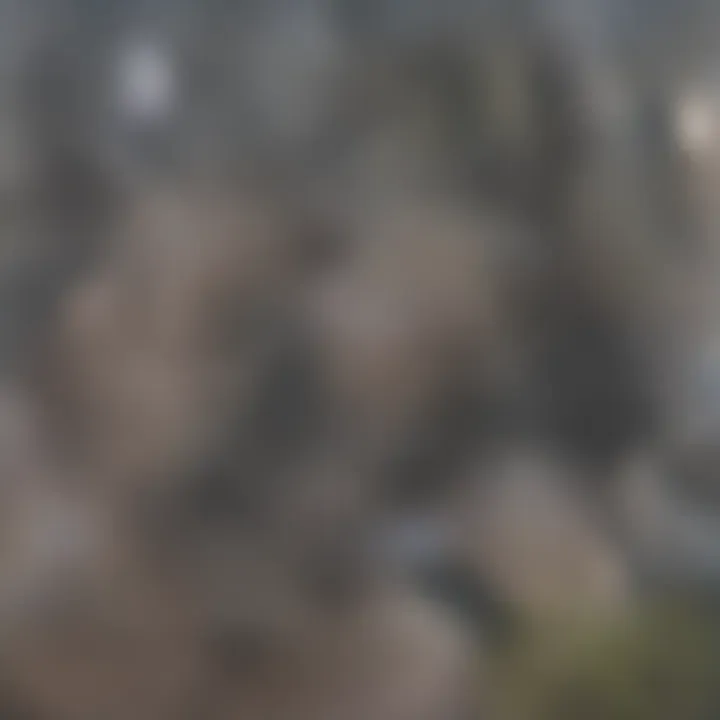
Factors influencing pharmacodynamics include:
- Receptor Binding: The interaction between drug molecules and their specific receptors.
- Dose-Response Relationship: The correlation between the amount of drug administered and the magnitude of its effect.
- Therapeutic Range: The concentration range within which the drug is effective without being toxic.
Understanding these dynamic interactions informs clinical decisions and helps tailor treatments to individual patient needs.
Importance of PK-PD Models
PK-PD models are invaluable in numerous aspects of drug development and clinical practice. They enable researchers to simulate drug behaviors and predict therapeutic outcomes under various conditions. By integrating pharmacokinetic and pharmacodynamic data, clinicians can optimize treatment regimens, thus improving therapeutic efficacy while minimizing adverse effects.
Moreover, with advancements in technology and data analytics, these models are evolving. They are increasingly incorporating real-world data to refine predictions and enhance personalized medicine approaches.
In summary, PK-PD models represent a fundamental framework for understanding drug actions and designing effective therapies. Their significance cannot be overstated, as they provide insights that are crucial for innovation and improvement in drug therapy.
Mathematical Foundations of PK-PD Models
Mathematical foundations play a crucial role in pharmacokinetic-pharmacodynamic (PK-PD) models. These models serve as the backbone for understanding how a drug behaves within the body and how it exerts its effects. By applying mathematical principles, researchers can accurately describe the processes of absorption, distribution, metabolism, and excretion of drugs, alongside their therapeutic effects. The integration of these elements ensures that PK-PD models not only reflect biological realities but also generate predictive insights that enhance clinical decision-making.
Basic Pharmacokinetic Equations
Pharmacokinetics involves a series of equations that quantify drug concentrations in various biological compartments over time. The fundamental equations include the one-compartment and multi-compartment models.
The one-compartment model assumes that the body acts as a single unit where the drug distributes uniformly. The main equations used here are:
- C(t) = C(0) e^-kt, where
C(t) is the concentration at time t,
C(0) is the initial concentration,
k represents the elimination rate constant.
In contrast, multi-compartment models are more complex as they consider different compartments such as the bloodstream and tissues. Here, the equations incorporate inter-compartmental clearance rates, allowing for a more nuanced understanding of the drugβs dynamics. The relevance of these equations lies in their ability to inform dosing regimens and understand potential drug interactions.
Development of Pharmacodynamic Models
Pharmacodynamics focuses on the mechanisms through which drugs exert their effects. It describes the relationship between drug concentration and effect, often emphasized through dose-response relationships. Development of pharmacodynamic models typically involves the use of sigmoidal functions or Emax models, where:
- E(max) = (E(max) * C)/(EC50 + C)
E(max) denotes the maximum effect achievable by the drug, C represents the concentration of the drug, while EC50 is the concentration that produces half of the E(max) response.
These models are not merely academic. They allow for predictions about how variations in drug dose will influence patient outcomes, thereby guiding effective treatment plans.
Differential Equations in PK-PD
Differential equations serve as the mathematical foundation for both PK and PD models. They describe the change in drug concentration over time through rates of processes such as absorption and elimination. For example, the following first-order differential equation characterizes drug elimination:
[ \fracdCdt = -kC ]
Where C is the concentration of the drug and k is the elimination rate constant. This equation forms the basis for much of the analysis in pharmacokinetics.
Additionally, systems of differential equations often capture the complex interactions inherent in multi-compartment models. These systems require numerical methods for solution, underscoring the need for computational tools in pharmacokinetics. The insight gained from these equations provides extensive predictive capabilities relevant to drug efficacy and safety.
Understanding the mathematical foundations of PK-PD models is essential for effective drug development and therapeutic advancements.
Types of PK-PD Models
Understanding the different types of PK-PD models is critical for the accurate simulation of drug actions within the body. These models allow researchers to predict how drugs influence physiological systems, guiding clinicians in their decision-making processes. Each model type comes with unique characteristics, advantages, and limitations, which influence their appropriateness in specific scenarios. This section will detail the primary types of PK-PD models, including linear versus non-linear models, compartment models, effect models, and population PK-PD models.
Linear versus Non-linear Models
Linear models assume a direct proportional relationship between drug concentrations and their effects. This means doubling the dose will result in a doubling of the response. Such simplicity facilitates straightforward calculations and easier interpretation. Linear models are often utilized in the early phases of drug action studies where the response is well-defined. However, they can fall short in complex scenarios where the response is influenced by biological factors.
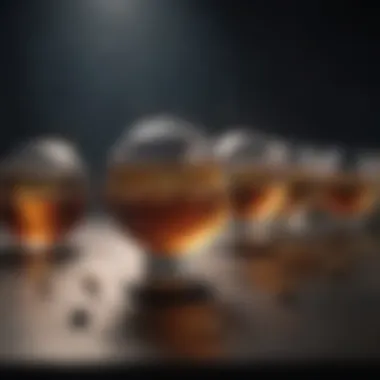
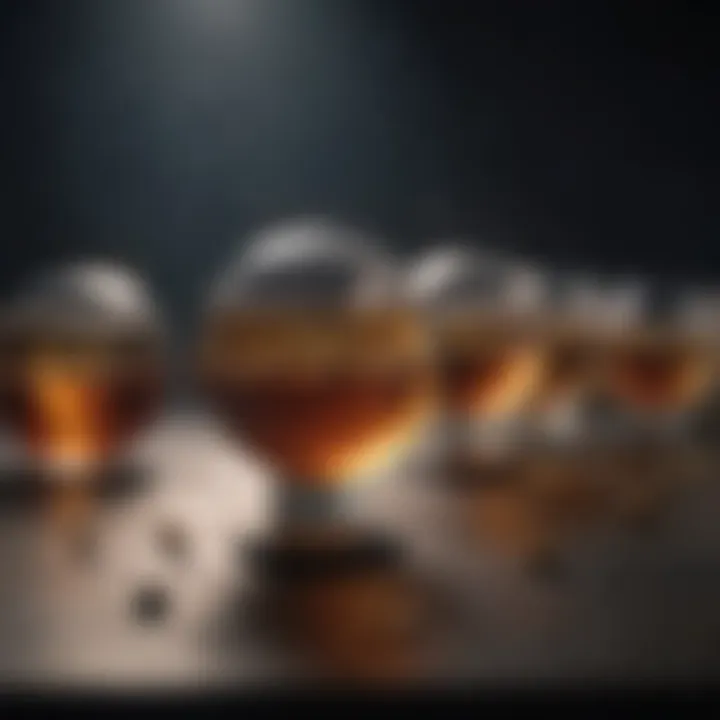
On the other hand, non-linear models can accommodate scenarios in which the relationship between drug concentration and effect varies. These models incorporate factors such as receptor saturation and can describe effects that do not correspond simply to drug dosage. Non-linear models might be essential for advanced pharmacodynamics, especially in drugs with saturable kinetics. They require complex mathematical formulations and may pose challenges in interpretation and validation.
Compartment Models
Compartment models are a foundational aspect of pharmacokinetic modeling. They divide the body into compartments that represent different physiological spaces, such as blood plasma, organs, and tissues. Each compartment can have distinct drug distribution and elimination properties.
A one-compartment model assumes uniform distribution and rapid equilibration between blood and tissues. In contrast, a multi-compartment model accounts for more nuanced dynamics, helping to describe drugs that are retained longer in specific tissues. Researchers favor compartment models for their ability to simplify complex biological processes, although they are limited by their assumptions of uniformity within compartments.
Effect Models
Effect models aim to link drug concentrations to their pharmacological effects. These models focus on the relation between dose and response, emphasizing the underlying mechanism of action. Common models include the Emax model, which describes the maximum effect a drug can achieve. This model captures the concept of potency and efficacy, helping to quantify how much concentration is needed to elicit a specific effect.
The development of these models is crucial for designing dosage regimens and understanding therapeutic windows. Each model has its own application scope, enabling researchers to determine optimal drug dosages and predict patient responses.
Population PK-PD Models
Population PK-PD models account for variability in drug responses across different patients. This variability might arise from genetic differences, age, weight, gender, and overall health status. By employing a population approach, researchers can develop more robust models that predict how specific groups of patients might respond to treatments.
Utilizing these models improves the understanding of how drugs behave in diverse populations. This also aids in optimizing dosage recommendations, enhancing drug safety and efficacy in clinical practice. Population models emphasize the need for a broader perspective in pharmacodynamics, embracing variability instead of neglecting it.
In summary, recognizing the different types of PK-PD models aids researchers in selecting the appropriate modeling approach based on their specific needs and contexts. Clear comprehension of these models contributes significantly to advancing pharmacological research and improving patient outcomes.
Applications of PK-PD Models
The applications of pharmacokinetic-pharmacodynamic (PK-PD) models are vast and significant in the realm of drug development and clinical practice. These models serve as essential tools in understanding drug behavior within the body and the effects they produce, thus informing numerous therapeutic decisions.
Clinical Pharmacology
In clinical pharmacology, PK-PD models provide a framework for optimizing drug therapy. They aid in predicting how different drug doses will affect plasma drug concentration over time. This understanding is crucial for determining the most effective dosing regimens to achieve the desired therapeutic effect without causing toxicity.
Furthermore, PK-PD models enable clinicians to understand drug interactions and their implications. For example, combining drugs can alter their pharmacokinetics or pharmacodynamics, resulting in enhanced or diminished drug efficacy. By utilizing these models, healthcare professionals can better manage such interactions, safeguarding patient safety while maximizing therapeutic benefits.
Drug Development Processes
During the drug development process, PK-PD models are indispensable. They guide researchers in the preclinical phase by predicting the drugβs behavior in human subjects based on animal studies. This prediction helps streamline the transition from laboratory settings to clinical trials, potentially reducing the time and costs involved in the drug approval process.
Moreover, integrating PK-PD models into clinical trials allows for more informed decisions about dosage and scheduling. These models can identify optimal dosing strategies that enhance efficacy while minimizing adverse effects. Consequently, companies can improve their drug formulations based on quantitative predictions from the models, leading to better outcomes in the market.
Therapeutic Drug Monitoring
Therapeutic drug monitoring (TDM) specifically benefits from the incorporation of PK-PD models. TDM involves measuring drug concentrations in a patient's body to ensure they remain within the therapeutic range. By applying PK-PD principles, clinicians can interpret these measurements more effectively, tailoring dosing adjustments to individual patient needs.
For instance, in patients with altered metabolism or excretion capacities, PK-PD models can help determine how the drug concentration might vary. This is vital for drugs with narrow therapeutic indices, where even small changes in drug levels can lead to efficacy losses or toxicity. By employing PK-PD models, healthcare providers can develop robust strategies for individualized medication management.
"The integration of PK-PD models into clinical practice enhances the efficacy and safety of drug therapies, making them a cornerstone of modern pharmacology."
Challenges in PK-PD Modeling
The field of pharmacokinetic-pharmacodynamic (PK-PD) modeling is significant, given its centrality in developing effective drug therapies. However, many challenges arise in the modeling process that can impact the accuracy and reliability of results. These challenges include issues surrounding data quality, model complexity, and regulatory constraints. Understanding these obstacles is essential for researchers and practitioners aiming to optimize drug development and therapeutic outcomes.
Data Quality and Availability
Data quality plays a critical role in PK-PD modeling. High-quality data ensures that models accurately reflect the behavior of drugs in the body and their effects on biological systems. Many models rely on clinical trial data which can vary significantly in terms of precision and completeness.
Some key factors affecting data quality include:
- Rigor of Experimentation: Data collected must be from well-designed studies with appropriate controls and sample sizes.
- Variability: Biological variability among individuals can skew results, leading to models that may not be universally applicable.
- Data Integrity: Proper management of data collection and curation processes is essential to prevent errors.
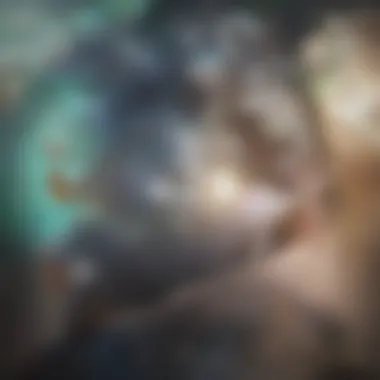
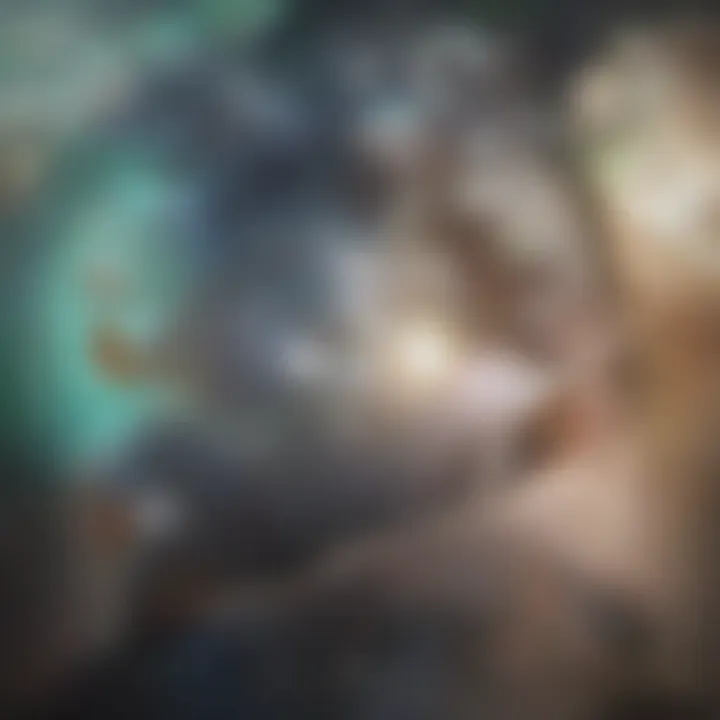
Moreover, there can be limitations in the availability of high-quality datasets for model development. This scarcity can hinder the ability to build robust models that can be applied broadly across patient populations. Collaboration between institutions and sharing of data can help alleviate this issue, but concerns around privacy and data security remain prominent.
Model Complexity and Validation
PK-PD models often involve intricate mathematical frameworks that can become quite complex. While sophisticated models can provide insightful outcomes, they also present challenges. One major concern is the balance between complexity and usability. More complex models may better capture real-world scenarios, but they can be difficult to validate and interpret.
Key considerations in this context include:
- Overfitting: Models that are too complex may fit the training data well but perform poorly on new data.
- Interpretability: Stakeholders must be able to understand the implications of models, which can be difficult if the model is overly intricate.
- Validation: Rigorous validation processes are necessary to confirm that models accurately predict drug behavior in various populations. This requires access to independent datasets for testing, ensuring models remain relevant despite continuous changes in science and methodology.
"Validation is not just a step; it is an ongoing process to ensure models stay aligned with reality."
Regulatory Constraints
Regulatory bodies play a crucial role in drug approval processes, and PK-PD models must adhere to their standards. These constraints can significantly impact how models are developed and used. Regulatory guidelines are often stringent, aiming to ensure that drug therapies are safe and effective for public use.
Considerations regarding regulatory constraints include:
- Compliance: Models must meet the requirements laid out by agencies such as the Food and Drug Administration (FDA) or the European Medicines Agency (EMA).
- Documentation: Adequate documentation is needed to support all phases of model development and application, including assumptions made during modeling.
- Flexibility: As regulatory science evolves, models must adapt to new guidelines, which can frequently change based on emerging evidence and technological advancements.
Overall, navigating the regulatory landscape is essential for model acceptance and successful drug approval. Addressing the challenges inherent in PK-PD modeling can lead to more effective therapeutic strategies and ultimately enhance patient care.
Future Directions in PK-PD Research
The field of pharmacokinetic-pharmacodynamic (PK-PD) modeling is continuously evolving. As we look ahead, several promising directions can reshape how we understand drug actions. It is crucial to explore these future trends, as they can lead to enhancements in drug development, efficacy, and patient care.
Integration of Systems Biology
Systems biology plays a fundamental role in advancing PK-PD modeling. By accounting for various biological components and interactions, researchers can achieve a more comprehensive understanding of drug dynamics.
- Holistic Approach: This integrative approach considers multiple pathways and variables, unlike traditional models that may oversimplify interactions.
- Data Utilization: Systems biology leverages high-throughput data from sources like genomics, proteomics, and metabolomics. This data infusion allows for more accurate predictions and modeling.
- Example Models: For instance, integrating cellular signaling responses with PK-PD models can reveal how cellular environment influences drug effects. Varied responses in a patient population can be better addressed.
Machine Learning Applications
Machine learning offers transformative potential for PK-PD research. By harnessing computational power and sophisticated algorithms, researchers can examine vast datasets efficiently.
- Predictive Analytics: Machine learning can predict pharmacokinetic parameters, which is crucial for identifying patient-specific dosing regimens. Algorithms work more accurately as more data becomes available.
- Pattern Recognition: Large datasets related to drug interactions can be analyzed to uncover previously unnoticed patterns in drug effects across diverse populations.
- Enhanced Precision: Implementing machine learning in model validation can improve predictive accuracy, ultimately leading to improved therapeutic outcomes.
Personalized Medicine Approaches
The move towards personalized medicine is another vital direction in PK-PD research. Individual variability in drug response is increasingly recognized, highlighting the need for tailored therapeutic strategies.
- Patient-Centric Models: By considering genetic, metabolic, and environmental factors, PK-PD models can help create more effective treatment plans. This is especially important in chronic disease management, where response varies.
- Targeted Therapies: Advanced modeling techniques can assist in designing drugs that target specific patient subgroups, enhancing efficacy and reducing adverse effects.
- Future Developments: Continuous advancements in biotechnologies and pharmacogenomics can further strengthen personalized approaches in PK-PD research.
Finale
In concluding this exploration of pharmacokinetic-pharmacodynamic models, it is essential to highlight the significant contributions these models make to the field of pharmacology. Understanding drug action is crucial for both researchers and clinicians, providing insights into how medications interact within biological systems. PK-PD models bridge the gap between drug concentration and its effects, ultimately guiding decision-making in drug therapy and development.
Summary of Key Insights
The discussion on PK-PD models has revealed several key insights:
- Foundation of Drug Action: We delved into the basic principles of pharmacokinetics and pharmacodynamics, illustrating how they interplay to affect drug efficacy and safety.
- Model Variability: Different types of PK-PD models cater to various scenarios, including linear and non-linear frameworks and population modeling, which can optimize treatment plans.
- Applications across Domains: The relevance of PK-PD models extends from clinical pharmacology to drug monitoring, highlighting their pivotal role in enhancing patient outcomes.
"A clear understanding of PK-PD models fosters advanced therapeutic strategies and promotes patient-centric care."
The Importance of Ongoing Research
The realm of pharmacokinetic-pharmacodynamic modeling is continuously evolving. With advancements in technology and methodology, ongoing research remains vital. Areas such as systems biology integration and machine learning applications hold promise for refining these models. Personalized medicine applications also emphasize the necessity for adaptive models that consider individual variability in drug response.
In summary, the continuous advancement of PK-PD research is not only beneficial but crucial for the progression of pharmacotherapy. By focusing on innovation and refining analytical techniques, the pharmaceutical field can ensure that medications are both effective and tailored to individual patient needs.
The depth of ongoing study in PK-PD modeling will ultimately lead to safer drug use and more effective clinical applications.