Exploring Experimental Design and Data Analysis Techniques
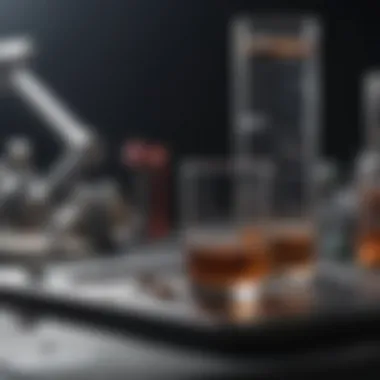
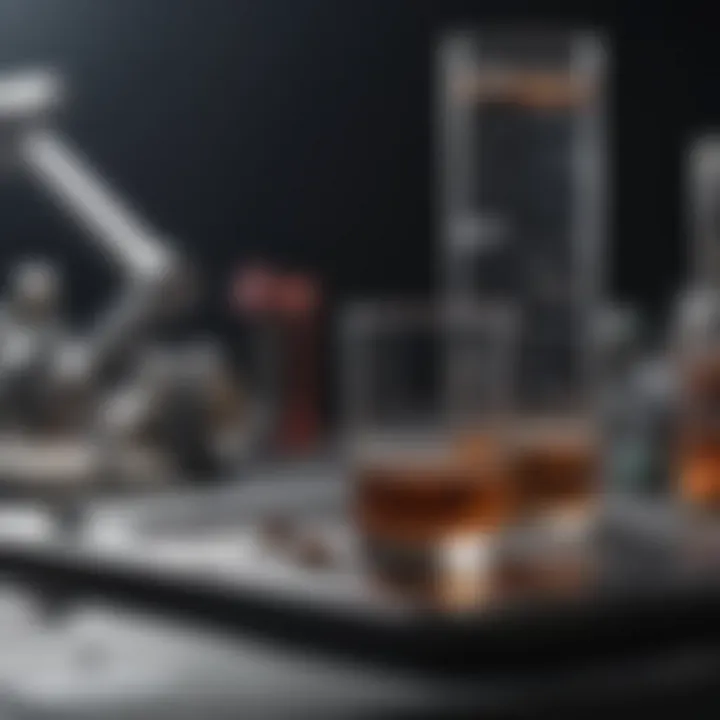
Intro
When it comes to research, the backbone of credible findings lies in the interplay between experimental design and data analysis. These two facets are not just necessary; they are deeply intertwined threads of the research tapestry. Establishing a sturdy experimental framework is essential, paving the way for effective data analysis which, in turn, can elevate the integrity of the outcomes.
In this intricate landscape, researchers must navigate through a myriad of choices. From formulating clear objectives and hypotheses to selecting suitable methodologies for data collection, each decision has its own ripple effects. A robust experimental design can enhance the reliability of results, while sound data analysis can unveil the true narrative behind the numbers. This article endeavors to take a closer look at this symbiotic relationship, offering insights that can empower scholars and professionals alike.
Key Findings
Major Results
Through this exploration of experimental design and data analysis, several critical findings emerge:
- The Importance of Clear Objectives: Researchers often falter at the starting line by selecting vague or overly broad objectives. It is crucial to have precise goals that guide every step of the research process.
- Diversity in Experimental Designs: Different experimental designs, such as completely randomized designs, factorial designs, and more, each come with their own sets of benefits and limitations. Understanding these can help in making informed decisions tailored for specific research questions.
- Significance of Sample Size: Larger sample sizes often lead to more reliable conclusions. However, this must be balanced against practical constraints, as smaller samples can sometimes still yield meaningful insights if approached wisely.
- Pitfalls in Data Interpretation: The nuance in data analysis requires careful consideration. Many fall into the trap of confirming existing biases rather than letting the data speak for itself. This article discusses ways to mitigate such biases.
"The design of an experiment is the map, while data analysis is the compass that helps the researcher navigate through uncertainty."
Discussion of Findings
The findings highlight the pivotal role that both experimental design and data analysis play in advancing science. By establishing clear objectives, researchers can streamline their approaches, leading to more relevant and interpretable data. Furthermore, by acknowledging the strengths and weaknesses of various methodologies, the article presents a framework for selecting the most impactful research designs. Understanding the intricacies of statistical methods can prevent common errors and lead to more accurate interpretations. This synthesis of insights adds substantial value to the investigative journey, nurturing a culture of rigorous and responsible scientific inquiry.
Methodology
Research Design
Research design forms the foundation of any study. Here are some common types:
- Descriptive Design: Focused on capturing the current state of a phenomenon without manipulation.
- Experimental Design: Involves controlled experiments where variables are manipulated to observe effects.
- Quasi-Experimental Design: Similar to experimental designs but lacks random assignment, allowing for the study of real-world scenarios without full control.
Each design is instrumental in answering different research questions, requiring a judicious selection based on the objectives.
Data Collection Methods
Data collection is pivotal for ensuring the accuracy and reliability of research outcomes. Various methodologies can be employed, including:
- Qualitative Techniques: Methods like interviews, focus groups, and observations that provide rich insights but may lack generalizability.
- Quantitative Techniques: Surveys and experiments that yield numerical data, allowing for statistical analysis and generalization.
- Mixed-Methods: Combining qualitative and quantitative approaches to leverage the strengths of both.
Choosing the right data collection method is not merely a task of preference but should align with the research design and objectives, further emphasizing the interconnected nature of these disciplines.
Understanding Experimental Design
Experimental design serves as the backbone of any research endeavor. It lays the groundwork for how studies are conducted and helps in generating reliable data. The essence of understanding this concept lies in the careful planning that enables researchers to explore hypotheses effectively, consider potential biases, and control for confounding factors. This ensures that findings are not just incidental or arising from poorly controlled environments.
A major benefit of grasping experimental design is its ability to inform and enhance the validity of research outcomes. When researchers design experiments thoughtfully, they provide a clear pathway to answering research questions. This clarity is vital in distinguishing between true effects and random variations. The careful thought put into experimental design is not just academic; it has real-world implications, informing practices across various fields such as psychology, medicine, and even engineering.
In this section, we will shed light on crucial aspects that define the principles of experimental design, delve into its significance, and analyze the key components that facilitate effective experimentation.
Defining Experimental Design
Experimental design can be defined as the structured approach to planning an experiment. It involves identifying the purpose of the study, the specific hypothesis being tested, and the methods that will be employed to gather and analyze data. A well-thought-out design mitigates biases and enhances the reliability of results. Essentially, it’s akin to drawing a blueprint for a house; without a clear plan, the outcome is uncertain at best.
Significance of Experimental Design
The significance of experimental design is twofold. Firstly, it provides a framework that enhances the clarity and objectivity of research. This clarity is paramount when drawing conclusions, as it distinguishes genuine findings from coincidental results. Secondly, a sound design allows for reproducibility, an essential aspect of scientific inquiry. In today's rapidly evolving research landscape, reproducible results lend credibility and foster trust among the scientific community and the public alike.
Key Components of Experimental Design
The elements that make up an effective experimental design are fundamental in determining the success of research outcomes. The first step is formulating clear objectives, followed by hypothesis formation and identifying the variables involved. Each key component plays a distinct role, which I will elaborate on below.
Objectives
Objectives outline what the researcher intends to achieve during the experiment. A well-defined objective allows for a focused approach and minimizes the chances of straying off-topic. It is the cornerstone of effective experimental design and ideally formulated to be specific, measurable, achievable, relevant, and time-bound (SMART). Having a robust objective ensures that the entire study remains on track and contributes significantly to the overarching goals of the research.
Hypothesis Formation
The hypothesis acts as a guiding light for the research study. It proposes a tentative explanation or prediction that the experiment aims to test. A strong hypothesis not only directs the focus of the experiment but also helps in hypothesis testing methods later on. Within this context, a well-formed hypothesis serves as a bridge connecting broad research questions to specific experiments. However, researchers must be cautious to avoid overstating claims based on weak or untested hypotheses, as this may lead to misleading interpretations.
Variables Identification
Identifying variables is crucial for minimizing confounding effects in experimental research. Variables refer to anything that can vary or change within the research context. It’s essential to distinguish between independent variables (which are manipulated) and dependent variables (which are observed for changes). Understanding how these variables interact contributes significantly to the integrity of the findings. Too often, neglecting to properly define and control for variables leads to compromised data integrity, which can undermine a study's overall reliability.
"Understanding experimental design is not just about structuring an experiment; it’s about fostering a clearer pathway to truth in research."
Types of Experimental Designs
Understanding the various types of experimental designs gives researchers essential tools to tailor their studies. Each type brings its nuances, catering to specific goals and contexts within research. The choice of design not only influences the data collected but also the inferences drawn from it. Here, we will elucidate four main types of experimental designs: Between-Subjects, Within-Subjects, Factorial, and Quasi-Experimental Designs.
Between-Subjects Design
Characteristics
In this design, different groups of subjects are exposed to different conditions. One of its key aspects is the simplicity in comparing groups. For instance, in a drug efficacy study, one group might receive the medication while the other gets a placebo. This design helps in determining the effect of a treatment without the risk of carryover effects, where the influence of one treatment impacts the subsequent treatment results. Its popularity stems from its straightforward approach in isolating treatment effects.
Advantages
The primary advantage of the Between-Subjects design is that it avoids concerns of order effects. This feature ensures that the responses from one group remain unaffected by previous treatments. Coupling this with random assignment means you have a sound footing for making causal claims regarding treatment differences. Due to its clarity, many preliminary studies often employ this design, as it simplifies both analysis and interpretation.
Disadvantages
However, this design isn’t all roses. A significant drawback is the need for a larger sample size to achieve robust results, as variations among individuals can obscure findings. Additionally, the inherent variability from one group to another may introduce noise into the data. Hence, researchers must balance the benefits and drawbacks carefully.
Within-Subjects Design
Characteristics
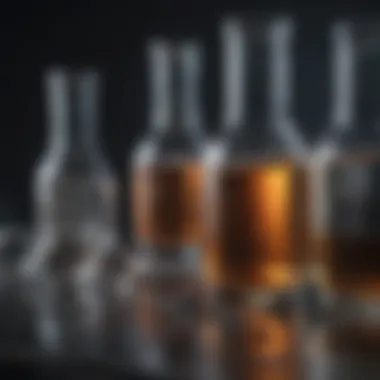
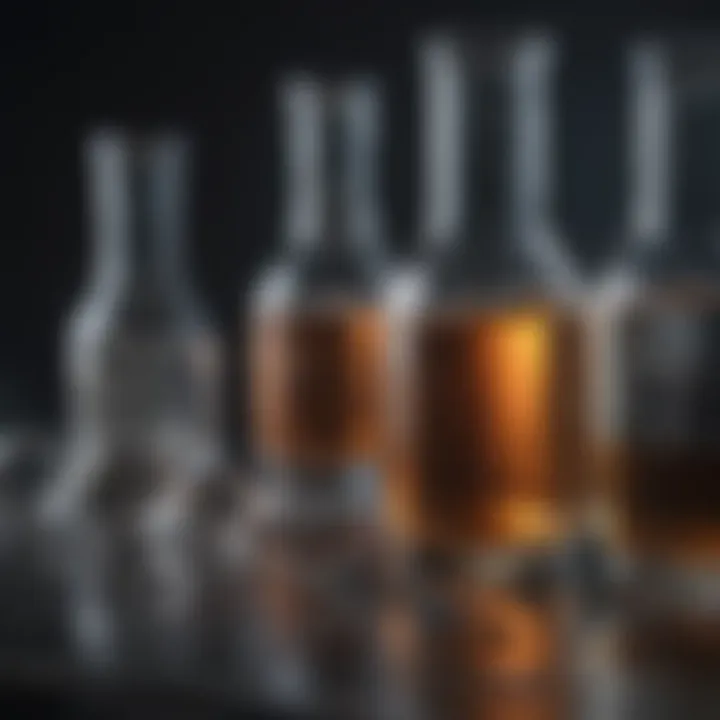
The Within-Subjects design, on the other hand, allows the same subjects to experience all conditions in the experiment. A crucial feature here is the reduction of variance since each participant is their control. For example, if studying reaction times under different lighting conditions, each participant tests under various lights, helping isolate the effect of lighting from individual differences. This method often results in more statistical power with fewer subjects.
Advantages
This design's biggest draw is the efficiency of fewer participants needed while still achieving strong results. It significantly reduces subject-to-subject variability since each subject acts as their control. When well executed, this means that researchers can draw more precise conclusions with less noise, giving clearer insights into behavior under diverse conditions.
Disadvantages
Yet, it’s not a free lunch. The main snag is the potential for carryover effects, where the effect of one condition spills over into another, potentially skewing results. Moreover, within-subjects designs often require more complex analyses and careful handling to ensure proper randomizations of trials.
Factorial Designs
Definition
Factorial Designs involve studying two or more independent variables simultaneously. This approach allows researchers to observe interactions between different factors, which is often critical in understanding multifaceted phenomena. For instance, a study could look at the effects of medication type and dosage simultaneously, providing a more rounded view of potential outcomes.
Complex Interactions
One of the most compelling aspects of factorial designs is the examination of complex interactions. They facilitate understanding of how two factors might interplay, influencing an outcome in ways that aren’t evident when each factor is considered in isolation. This can highlight unforeseen side effects or benefits of a treatment or intervention.
Usage Scenarios
This design is particularly beneficial in exploratory research where the interplay of factors is essential to answer pressing questions in the field. Common scenarios where factorial designs shine include agricultural studies on crop yield, psychological studies on stress, and clinical trials involving multiple treatments. However, they also require careful planning and sufficient resources to manage the extensive data generated.
Quasi-Experimental Designs
Definition
Quasi-Experimental Designs offer a pragmatic approach when random assignment isn’t feasible. Definition-wise, they aim to evaluate the effects of interventions without the rigorous controls of true experiments. For example, assessing the impact of an education program in schools might not allow for random assignment due to ethical or logistical reasons.
Challenges
One of the most prominent challenges with quasi-experimental designs is the potential for bias due to lack of randomization. Without random placement of subjects, pre-existing differences may confound results, making it harder to ascertain cause and effect reliably. Thus, careful consideration in interpreting results is crucial, often necessitating additional statistical controls to mitigate these biases.
Applications
Despite challenges, these designs are often applied in real-world settings. They are invaluable in fields like public health and education, where experimental manipulation is impractical. They offer versatile ways to assess programs' effectiveness, though the interpretations drawn from such designs often require more cautious framing alongside the acknowledgment of limitations.
Data Collection Methodologies
Data collection methodologies are the backbone of empirical research. Without a well-thought-out plan for gathering data, any conclusions drawn from a study may be flawed. Effective methodologies help ensure that researchers capture relevant information, enabling them to draw meaningful inferences from their work. Here, we delve into various approaches used for data collection, showcasing the strengths and challenges inherent in each method.
Qualitative Data Collection
Methods Overview
Qualitative data collection methods are deeply focused on understanding the nuances of human behavior and experience. These methods prioritize exploring context, meanings, and complexities rather that numerical figures. One prominent way to gather qualitative data is through interviews, where researchers engage with participants in an open dialogue. The beauty of this method lies in its flexibility; it allows researchers to probe further based on participants' responses, leading to a more thorough understanding of the subject matter.
However, qualitative methods can be time-consuming, requiring considerable effort in arranging interviews and analyzing the vast amounts of narrative data that arise from them.
Analysis Techniques
Analyzing qualitative data involves interpreting patterns and themes that emerge from collected information. Popular analysis techniques include thematic analysis, where researchers identify common themes or ideas that recur across various responses. This approach can be highly beneficial, as it provides insight into shared experiences and cultural significances.
Nevertheless, the challenge with qualitative analysis is its subjective nature. Different researchers may interpret the data in various ways, which can lead to inconsistencies or biases if not approached carefully.
Application Contexts
Qualitative data collection is especially valuable in exploratory research or when investigating less understood phenomena. For instance, understanding patient experiences in healthcare settings can glean rich, insightful data that surveys may miss. The key characteristic of this method is how it can capture the depth of human experience, offering a narrative that numbers simply cannot.
That said, researchers must be mindful that qualitative findings cannot be generalized to the wider population, which poses certain limitations.
Quantitative Data Collection
Methods Overview
Quantitative data collection provides numerical data that can be used for statistical analysis. By utilizing methods such as surveys with closed-ended questions, researchers can gather large volumes of data in a relatively short timeframe. This allows for a more straightforward means of data comparison and analysis.
The key benefit of quantitative methods is the ability to perform statistical tests and generate findings that hold greater generalizability across populations. However, one significant drawback is that these methods often lack depth and fail to capture underlying motivations or attitudes.
Statistical Relevance
The statistical relevance of quantitative data is significant, as it allows researchers to establish relationships between variables. By employing statistical techniques, like regression analysis, researchers can demonstrate how changes in one variable relate to changes in another, leading to more robust conclusions.
This strength, however, can turn into a double-edged sword. If the data collection methods are flawed or the sample isn’t representative, the statistical outcomes can mislead as easily as they can inform.
Data Integrity
Data integrity in quantitative research is paramount. Researchers must ensure that data is collected consistently and accurately, as any discrepancies can severely impact results. For example, adopting rigorous data management protocols can safeguard data integrity. However, the challenge often lies in the sheer volume of data that needs scrupulous checking, leading to potential oversights and errors.
Mixed Methods Approach
Rationale
Mixed methods research combines both qualitative and quantitative data collection to leverage the strengths of each. The rationale behind this approach is its potential to provide a more complete understanding of research questions. For example, a researcher might use quantitative surveys to determine trends, followed by qualitative interviews to explore those trends more deeply.
This approach is advantageous as it allows for richer data interpretation and nuanced insights. Yet, it also requires careful planning to integrate both data types effectively.
Data Triangulation
Data triangulation is an integral aspect of mixed methods research. It involves converging information gathered from different sources or methods to confirm findings. This practice adds credibility to the results, as it demonstrates consistency across various data types.
The unique feature of triangulation is that it can uncover contradictions or unexpected insights that might be overlooked when using a single data type alone.
Combining Insights
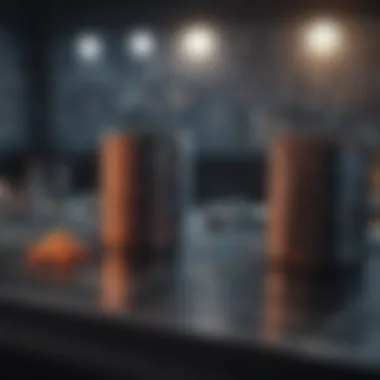
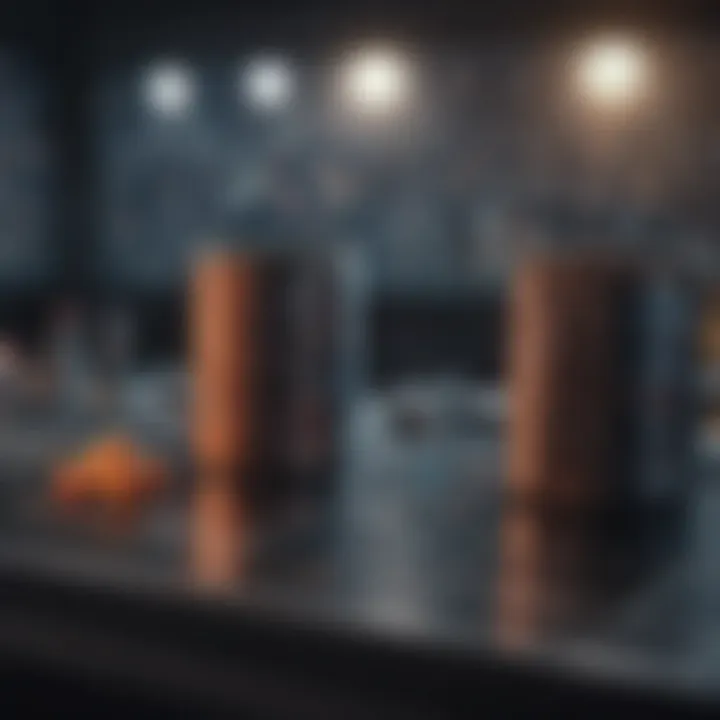
Combining insights from qualitative and quantitative research allows researchers to tackle complex research questions from multiple perspectives. This multifaceted view is particularly beneficial in fields like education or health sciences, where understanding both statistical trends and personal experiences enriches insights.
However, one must balance the depth with breadth. Overemphasis on one aspect can skew the analysis, so careful consideration needs to be given to how insights are synthesized.
"By acknowledging the limitations and strengths across different methodologies, researchers can significantly improve the quality and reliability of their findings."
Critical Aspects of Experimental Design
Understanding the critical aspects of experimental design is the backbone of any sound research. These fundamentals determine how reliable and valid the results of an experiment will be, serving as a roadmap for researchers. When diving into this topic, it’s vital to grasp not just the mechanics but the nuanced connections between design elements and the findings they yield. Here, we’ll discuss important sections like sample size, randomization, and controlling for confounding variables.
Sample Size and Power Analysis
Importance of Sample Size
The sample size selected for an experiment plays a crucial role in the outcomes of any study. In an ideal world, researchers would want their sample to be as large as possible, but practical constraints often dictate otherwise. The larger the sample, the more likely it is to represent the population accurately, reducing the margin of error. In this context, the benefit of a well-chosen sample size lies in its ability to generate meaningful data that reflects true trends, rather than random noise.
A larger sample size helps in achieving more statistical power, which means the likelihood of detecting an effect, if there is one, is significantly higher. This creates a stronger foundation upon which conclusions can be drawn. However, excessive sample sizes can lead to wasted resources and inflated expectations, which can then skew the interpretation of results.
Calculating Statistical Power
Calculating statistical power allows researchers to determine the probability of correctly rejecting the null hypothesis. The power of a test is influenced by several factors, primarily sample size, effect size, and significance level. Knowing how to calculate this can be invaluable. With adequate power, the chances of missing a true effect diminish, thereby enhancing the reliability of the findings.
A robust power analysis gives insight into whether the study is adequately equipped to address its hypotheses. If not, adjustments to sample size or study parameters may need to be made. However, while power calculations can safeguard against Type II errors, focusing solely on power can sometimes overshadow other important considerations, like study ethics or logistical feasibility.
Methodological Implications
Understanding the methodological implications of experimental design is essential for achieving meaningful results. This encompasses not only the design’s theoretical attractiveness but also its practical feasibility and relevance in real-world scenarios. Choosing the right methodology could be the difference between obtaining actionable insights and ending up with inconclusive data.
It's often the case that a design that’s theoretically sound may fall short in practice due to unforeseen complications. Researchers must keenly evaluate these methodological concerns to align design choices with research goals. Balancing method with feasibility can sometimes constrain idealistic approaches but ultimately guides researchers toward reliable outcomes.
Randomization Techniques
Purpose of Randomization
The primary purpose of randomization in experimental design is to eliminate bias. When participants are randomly assigned to various groups, each group is expected to be similar in terms of known and unknown variables, thereby isolating the effects of the treatment being tested. This simple yet powerful technique lays a critical foundation for interpretation, making any observed differences more likely attributable to the treatment rather than confounding factors.
In this way, randomization enhances the validity of the study by safeguarding against selection biases that might skew results. However, failing to implement randomization could lead to questionable conclusions, undermining the trustworthiness of the research.
Types of Randomization
There are various ways to implement randomization, such as simple random sampling, stratified random sampling, or block randomization. Each type offers unique advantages and may be preferable based on the study's specifics. Simple random sampling ensures every individual has an equal chance of being selected, while stratified sampling can ensure that specific subgroups are represented appropriately.
For larger scale trials, block randomization might be used to ensure balanced group sizes. Understanding these techniques provides researchers with tools to craft experiments that are not only valid but also relevant for their intended investigations. Nevertheless, the complexity introduced by different types of randomization can sometimes be overwhelming and may deter researchers from fully utilizing its potentials.
Impact on Validity
The impact of randomization on validity can’t be understated. When properly executed, randomization can dramatically increase the internal validity of an experiment while also elevating external validity by ensuring the sample truly represents the broader population. This dual advantage is crucial for shaping the applicability of research findings, allowing for broader generalizations beyond just the test subjects.
However, it’s worth noting that randomization alone does not guarantee validity—other factors, including sample size and measurement reliability, play significant roles too. Without a comprehensive understanding of the interplay between these elements, researchers may find themselves grappling with unexpected interpretations of their findings.
Controlling for Confounding Variables
Identification of Confounders
Claiming a solid experimental design must involve recognizing confounding variables can be a slippery slope; they lurk in the background, threatening the integrity of research outcomes. A confounder is an extraneous variable that correlates with both the independent and dependent variables, potentially leading to misleading conclusions about relationships. Identifying these confounders is critical; if not, findings might suggest relationships that simply do not exist.
Popular strategies for identifying confounders include literature reviews and statistical techniques such as regression analysis. These tools help in pinning down potential disruptors that could throw a wrench in your results. Yet, hunting down confounders is more complex than merely listing variables—such analysis requires a deep understanding of the context and methodology.
Strategies for Control
Once confounders are identified, researchers must employ strategies to control for them. Various approaches exist, such as matching participants based on confounding characteristics, utilizing statistical control, or stratifying samples. Each of these techniques serves to mitigate the identified biases that could affect study outcomes.
This control is not without its trade-offs; for example, matching might limit the diversity of the sample, while statistical controls require robust data, which might not always be feasible. Therefore, a careful balancing act is crucial to maintain the experimental design's integrity while managing confounding factors effectively.
Impact on Research Integrity
The integrity of any research hinges on a careful balance—between adequately addressing confounding variables and maintaining adaptability in design. The way confounders are managed speaks volumes about the study's credibility. Failing to control for these variables can lead to inflated or distorted findings, ultimately impacting the trustworthiness of the research completely.
By acknowledging and addressing confounding variables with considered strategies, researchers not only enhance their studies' validity but also contribute to the overall credibility of scientific discourse. In a world where data integrity is increasingly scrutinized, making these efforts is essential for maintaining the respect of both academic and lay communities.
Data Analysis Techniques
Data analysis techniques form the backbone of interpreting research findings and placing experimental results in context. Their importance stretches far beyond just crunching numbers; they offer researchers tools to draw meaningful conclusions and facilitate deeper insights into their data. Effectively using data analysis techniques can streamline the research process and bolster the overall validity of findings. In the following sections, we will delve into descriptive statistics, inferential statistics, and advanced analytical techniques.
Descriptive Statistics
Descriptive statistics serve as the foundation for summarizing and understanding data sets. The key aspect of descriptive statistics lies in its ability to present large amounts of data in a comprehensive manner, making it easier for researchers to decipher trends and patterns.
Measures of Central Tendency
In the realm of measures of central tendency, we find three primary figures: the mean, median, and mode. Each of these measures holds its own significance, contributing uniquely to data interpretation. The mean, or average, is often the go-to choice because it considers all values, providing a holistic view of the data. However, its sensitivity to outliers can skew interpretations, leading to possibly misleading conclusions.
The median is an alternative that comes with its own advantages. It is simply the middle value in a sorted dataset, which remains unbothered by extreme values. This makes it especially advantageous in skewed distributions, allowing researchers a more stable view of the data. The mode, although less frequently utilized, represents the most common value and can be particularly useful in categorical datasets. In summary, central tendency measures present a simplified overview of complex data, aiding in establishing the groundwork for further analysis.
Measures of Variation
Measures of variation, including range, variance, and standard deviation, go hand in hand with measures of central tendency. They provide insight into the spread of data, offering a more comprehensive view when evaluating results. The standard deviation, in particular, gauges how spread out the data points are around the mean, offering a clearer perspective on data distribution.
This detail can help to establish whether results are generally consistent or erratic—an invaluable insight in research settings. Variance, while similar to standard deviation, expresses variability in its square units. The choice between these measures often hinges on the data type and its suitability for a particular analysis. Overall, understanding these aspects of variation aids researchers in interpreting data more reliably and effectively.
Data Visualization
Data visualization acts as a bridge between raw numbers and understanding. Through graphs, charts, and plots, researchers can portray complex data sets clearly. The notable characteristic of data visualization is its ability to simplify data interpretation, highlighting trends at a glance.
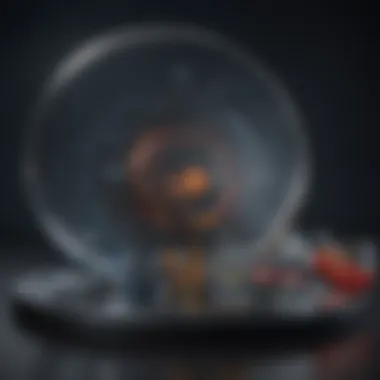
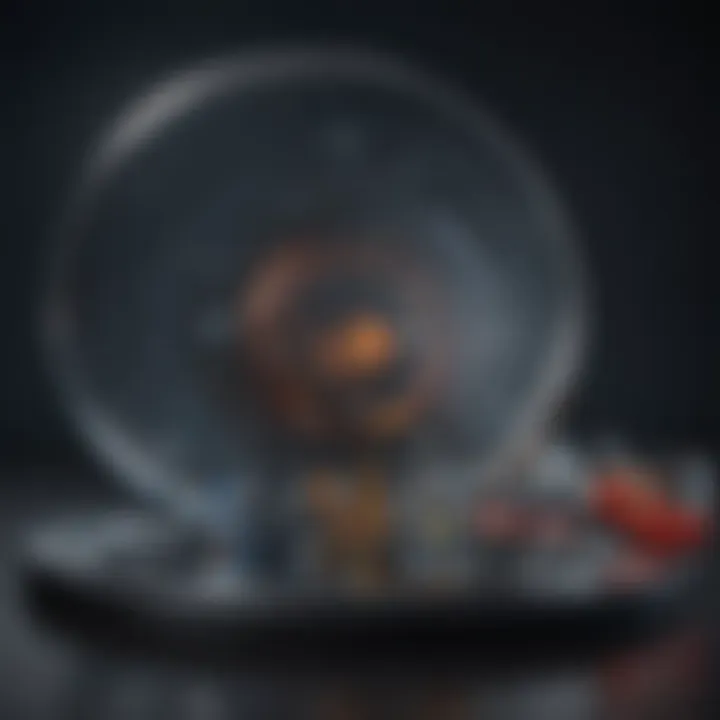
However, creating effective visual representations requires careful consideration of design elements—too much clutter can obscure the message. Well-designed visuals promote insight and comprehension, transforming abstract statistics into intuitive narratives.
Inferential Statistics
Inferential statistics expands the scope of analysis beyond the immediate data set, allowing researchers to make predictions and draw conclusions about larger populations based on sample data. This ability is critical as it aids in generalizing findings and establishing the broader relevance of a study.
Prelude to Hypothesis Testing
An essential aspect of inferential statistics is hypothesis testing. This process involves formulating a null hypothesis to test against an alternative hypothesis. Essentially, it assists researchers in determining whether observed data reflects true effects within the population or if they are merely due to chance. This systematic approach allows researchers to establish statistical significance and contributes significantly to validating findings.
The unique feature here is its structured methodology, which provides a framework for rigorous analysis. Nevertheless, the reliance on predefined significance levels can sometimes lead to contentious interpretations, especially in fields with less clear-cut criteria.
Common Statistical Tests
Numerous common statistical tests come into play within this sphere, including t-tests, chi-square tests, and ANOVA. Each test serves a particular purpose—t-tests compare means, chi-square tests assess categorical variables, and ANOVA explores differences among multiple groups. Selecting the right test is crucial as it directly influences the validity of conclusions drawn.
Statistical tests not only validate research but also establish a pathway for replicability in scientific inquiry.
Interpreting Results
The interpretation of results encapsulates the heart of the analysis process. It's not just about achieving statistical significance; it’s about understanding what an outcome means in the context of the hypothesis. This requires a critical eye and an understanding of the nuances involved.
A key characteristic of result interpretation is how it links back to the original research questions and hypotheses, creating a cohesive narrative throughout the research journey. The unique challenge faced here is distinguishing between correlation and causation, which often trips up even seasoned researchers. Proper interpretation ensures the findings are communicated effectively and responsibly.
Advanced Analytical Techniques
As research becomes increasingly sophisticated, advanced analytical techniques gain attention. These methods offer deeper insights into data, uncovering complex relationships that standard methods might miss.
Regression Analysis
Regression analysis helps establish connections between variables, offering predictive insights that standard approaches may overlook. Its primary feature is the ability to model relationships, enabling researchers to understand how one variable can affect another.
This method is immensely beneficial for various fields, allowing for thorough data interpretations and policy recommendations. However, while regression can highlight trends, it’s essential to remember that correlation does not definitively imply causation, stressing the importance of context.
ANOVA
ANOVA, or Analysis of Variance, is a robust technique that compares the means across multiple groups to ascertain if at least one differs significantly. This method enhances the ability for researchers to draw contrasts in experimental outcomes, adding depth to discussions around treatment effects.
One unique quality of ANOVA is its capability to assess interactions among factors, which can reveal intricate relationships in complex datasets. However, interpreting ANOVA results involves careful consideration of assumptions underlying the technique.
Multivariate Analysis
Multivariate analysis embraces the complexity of data involving multiple variables simultaneously. This approach is designed to uncover patterns and relationships that univariate approaches may miss, offering a broader scope of analysis.
The unique feature of this technique lies in its potential to analyze interrelations among different variables, providing comprehensive insights. But, with increased complexity also comes higher demands for interpretation and understanding, as results can be multifaceted.
Common Pitfalls in Data Interpretation
Data interpretation is a critical process that demands both accuracy and caution. Missteps in this area can lead to oversized conclusions that don't do justice to the data at hand, undermining research credibility. In this section, we will discuss common pitfalls that researchers often encounter, focusing on how these pitfalls can skew findings, mislead stakeholders, and potentially derail ongoing research efforts.
Overgeneralization of Results
One of the most prevalent errors in data interpretation is the overgeneralization of results. This occurs when findings from a specific sample are applied too broadly to the general population. An example would be stating that a drug is effective for all age groups based solely on a study conducted with a small group of young adults.
Such over-enthusiastic claims can be perilous. Researchers must acknowledge that findings derived from a particular study might not extend to different demographics, settings, or contexts. It’s critical to emphasize the limitations of the study sample and the potential biases that may exist. To navigate this issue, one could diagram the characteristics of their sample, contrasting it with the larger population to offer clarity. The practice of diligence in reporting can mitigate the chances of making sweeping statements that generalize findings without due consideration.
Misinterpretation of Correlations
Research often relies on correlations to reveal relationships between variables. However, a common stumbling block is misinterpreting these correlations as indicative of causation. For instance, if a study finds a correlation between ice cream sales and shark attacks, it would be erroneous to conclude that buying ice cream leads to shark encounters. Instead, understanding the underlying variables—such as seasonal changes—can provide a clearer picture.
To avoid this pitfall, researchers must drill down into their data, investigating and providing context for any observed correlations. Properly articulating the differences between correlation and causation is vital. By employing a rigorous approach to data analysis and emphasizing the distinctions in reports, researchers can foster a deeper understanding of their findings. In the world of statistics, as they say, “Correlation does not imply causation.”
Neglecting Effect Size
While statistical significance is often highlighted in research findings, neglecting effect size is an oversight that can misrepresent the implications of the data. Effect size provides insight into the magnitude of a relationship or difference. For instance, a study might find a statistically significant effect of a teaching method, but if the effect size is marginal, it could indicate that the method may not be practically relevant.
Ignoring effect size can lead to the prioritization of statistically significant results without understanding their real-world impact. A good practice would be to report both p-values and effect sizes in publication, ensuring that the audience grasps the fuller narrative behind the numbers.
In summary, the interpretation of data is an intricate dance that requires finesse and rigor. By remaining vigilant against overgeneralization, misinterpretation of correlations, and neglecting effect size, researchers can enhance the integrity of their work, thereby enriching the scientific dialogue. It is paramount to convey the limitations and nuances of findings clearly, steering clear of hasty conclusions and fostering a culture of thoughtful inquiry.
"In research, caution is as essential as curiosity; both are required to navigate the complex landscape of data interpretation."
Adopting a careful and considered approach to data interpretation not only benefits individual researchers but also the broader academic community.
Finales and Future Directions
The discussion surrounding experimental design and data analysis is crucial for advancing scholarly research and ensuring valid interpretations of data. The conclusions drawn from any scientific endeavor should not only summarize findings but also illuminate potential pathways for future exploration. By understanding the interplay between these two elements, researchers can create sturdy frameworks that guide their inquiries, allowing for more accurate conclusions and innovative applications.
Summary of Key Insights
To encapsulate the key takeaways from this exploration, it's essential to recognize the significance of meticulously structured experimental designs. The emphasis on clear objectives, hypothesis development, and rigorous methodologies lays the groundwork for robust data collection and analysis. Here are the core insights:
- Experimental Design Matters: The choice of design affects the validity and generalizability of the research outcomes.
- Data Collection Approaches: A blend of qualitative and quantitative methods can provide a fuller picture.
- Statistical Techniques: Familiarity with various analytical methods is vital for drawing sound conclusions.
- Avoiding Pitfalls: Awareness of common flaws in data interpretation is crucial for enhancing research quality.
Overall, the insights serve as a springboard for further inquiry and adaptation in research designs to keep pace with evolving scientific demands.
The Importance of Continued Research
Research is not a one-and-done affair. Continuous studies help to refine existing theories and challenge established norms. The rapidly changing nature of science—especially with emerging technologies and methodologies—demands that researchers remain vigilant, inquisitive, and ready to adapt. Increased scrutiny and discussions around experimental design and data interpretation can lead to breakthroughs in understanding complex phenomena.
"Science is a way of thinking much more than it is a body of knowledge." – Carl Sagan
Moreover, continued research facilitates the discovery of biases or systematic errors in previously accepted methods. Over time, updating and expanding methodologies ensures that research remains relevant to real-world issues, addressing contemporaneous challenges.
Emerging Trends in Experimental Design and Analysis
Looking towards the horizon brings a number of exciting trends that signal the evolution of experimental design and data analysis. Some noteworthy trends include:
- Increased Use of Technology: The integration of artificial intelligence and machine learning in data analysis is reshaping how experiments are designed and interpreted.
- Adaptive Designs: Flexibility in experimental designs allows researchers to modify their approaches based on interim results, thus enhancing efficiency.
- Open Science Movement: Transparency in research encourages collaboration and replication of studies, which improves the overall integrity of scientific work.
- Focus on Real-World Applications: There's a growing emphasis on correlating laboratory results with field data to address practical challenges effectively.
These trends reflect a shifting landscape in research, where interdisciplinary approaches and technological advancements create opportunities for richer discussions and more impactful findings. As researchers engage with these emerging dynamics, the cycle of inquiry, design, and analysis will evolve, pushing the boundaries of what is known toward what is yet to be discovered.