Cognitive Psychology Meets AI: Exploring Their Connection
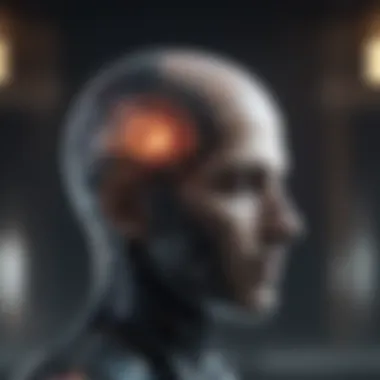
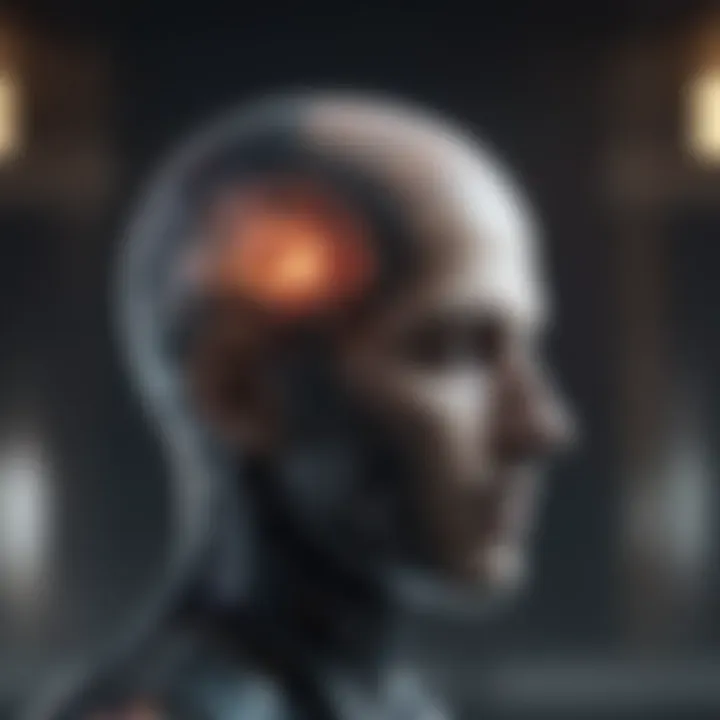
Intro
Cognitive psychology and artificial intelligence are two fields that at first glance might seem distant from each other, yet they are deeply intertwined. Cognitive psychology studies the mind and its processes, including how people think, learn, and remember. On the contrary, artificial intelligence aims to create systems that can perform tasks that typically require human intelligence. Understanding how these fields influence one another can reveal significant insights into both human cognition and the development of AI technologies.
The relationship between these areas is complex and reciprocal. Cognitive psychology provides essential theories that guide the creation of AI algorithms, while AI systems can offer valuable tools for investigating cognitive theories. The convergence of these disciplines holds the promise of advancing our grasp of human and machine learning alike. This article seeks to unravel this intricate relationship and emphasize the importance of integrating knowledge from both domains.
Preface to Cognitive Psychology and AI
Cognitive psychology and artificial intelligence are two domains that, while distinct, share a significant connection. The study of cognitive psychology focuses on understanding mental processes such as perception, memory, and reasoning. On the other hand, artificial intelligence aims to replicate human intelligence through machine learning and computational models. Recognizing the intersection of these fields is essential, as it helps in leveraging insights from one area to advance the other.
The relevance of this introduction lies in its ability to outline the foundational concepts that will be explored in detail later in the article. By examining cognitive psychology, we can better appreciate the principles that guide AI development. Likewise, understanding AI's capabilities allows for a deeper reflection on cognitive processes in humans. This intersection offers unique benefits, including more effective AI development and enhanced insights into mental functions.
Defining Cognitive Psychology
Cognitive psychology is a branch of psychology that studies how people think, learn, and remember. It delves into the processes underlying cognition and the ways these processes can be influenced by both internal and external factors. Cognitive psychologists often explore mental functions such as problem-solving, language acquisition, and decision-making. This field combines theories and empirical research to form a comprehensive understanding of how the mind operates.
- Key areas of focus in cognitive psychology include:
- Perception: Understanding how we interpret sensory information.
- Memory: Studying how information is encoded, stored, and retrieved.
- Attention: Investigating how we select and concentrate on specific aspects of our environment.
- Language: Examining the processes related to understanding and producing language.
- Problem Solving: Exploring how individuals approach and resolve challenges.
Cognitive psychology relies heavily on experimental methods to substantiate its theories. It seeks to create models that predict cognitive function and behavior.
Overview of Artificial Intelligence
Artificial intelligence refers to the simulation of human intelligence in machines programmed to perform tasks normally requiring human intelligence. These tasks encompass various applications, including visual perception, speech recognition, decision-making, and language translation. AI technologies can be broadly classified into two categories: narrow AI, which is designed for specific tasks, and general AI, which seeks to mimic human cognitive abilities across a range of activities.
AI development involves several core components:
- Machine Learning: A method that allows systems to learn from data and improve their performance over time.
- Natural Language Processing: Enabling machines to understand, interpret, and respond to human language.
- Computer Vision: Allowing machines to interpret and understand visual information from the world.
- Robotics: Integrating AI into physical machines to perform tasks in real-world environments.
The potential of AI to understand and process information at scale offers invaluable resources for researchers in cognitive psychology. This synergy encourages the exploration of various interdisciplinary applications.
Theoretical Foundations of Cognitive Psychology
The theoretical foundations of cognitive psychology are essential for understanding how cognitive processes such as thinking, memory, and problem-solving work. This area of study offers valuable insights that directly influence the design and functionality of artificial intelligence systems. Cognitive psychology emphasizes the systematic study of mental processes, helping researchers and practitioners create better models of human behavior. These models are pivotal in the development of AI, as they provide the frameworks necessary for creating algorithms that can mimic, predict, and enhance cognitive behaviors.
Incorporating cognitive psychology into AI not only helps in refining machine learning techniques but also informs ethical implications. As AI systems become more integrated into daily life, the importance of understanding human cognitive processes becomes even more critical. This understanding aids in developing systems that not only operate effectively but are also aligned with human values and behaviors.
Information Processing Model
The information processing model is a central concept in cognitive psychology. It likens the human mind to a computer, one which takes input from the environment, processes the information, and produces output. This model is crucial for researchers because it helps in visualizing how humans perceive, store, and retrieve information. For AI, this model provides a baseline for developing algorithms that simulate human-like processing.
Key aspects of the information processing model include:
- Input: Perceptual processes gather information from the environment.
- Processing: Cognitive processes interpret and manipulate the information.
- Output: Responses are generated based on the processed information.
Understanding this model aids in the design of AI systems that engage in similar processes. It allows for enhanced user experiences through adaptive interfaces that respond to human input intelligently.
Cognitive Development Theories
Cognitive development theories offer insights into how individuals construct knowledge over time. Jean Piaget, for example, identified stages of cognitive development that illustrate how thinking evolves from childhood to adulthood. These theories are significant because they inform the design of educational AI tools. By understanding how learning occurs, AI systems can be tailored to support users at various stages of cognitive maturity.
Considerations in cognitive development include:
- Stages of Learning: Recognizing different cognitive growth phases which affect how information is absorbed.
- Constructivist Approaches: Encouraging active involvement in learning processes.
AI applications can integrate these concepts to provide personalized learning experiences, adapting challenges to the userβs developmental level.
Cognitive Neuroscience Integration
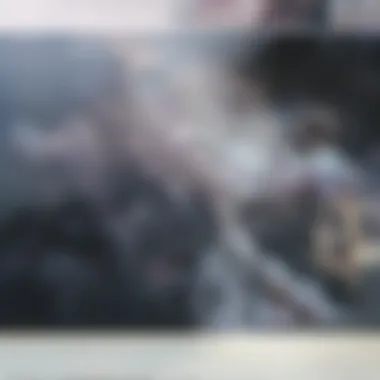
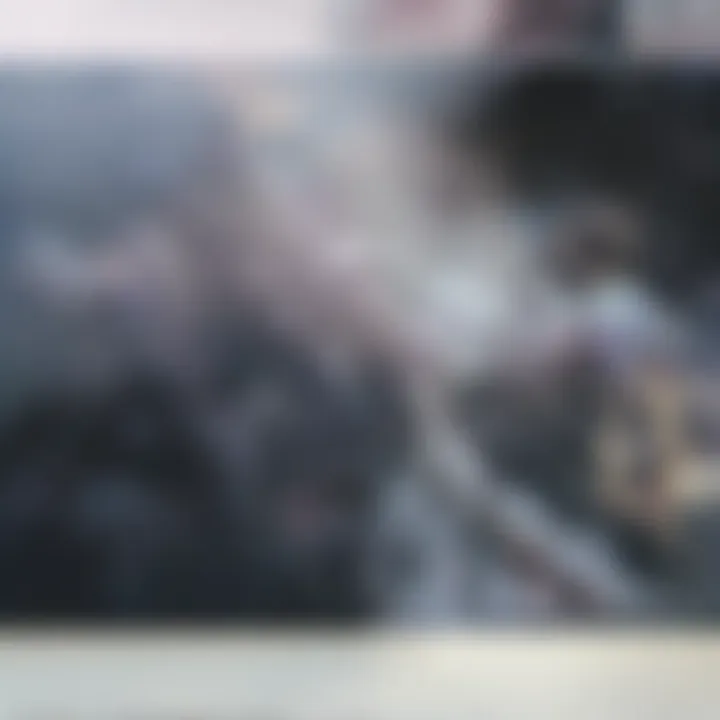
Cognitive neuroscience bridges the gap between cognitive psychology and biology. It examines how brain function influences cognitive processes. This integration is important for AI because it enables the development of systems that consider the neural underpinnings of human thinking.
Key areas of focus in cognitive neuroscience include:
- Neuroimaging Techniques: Tools like fMRI that allow researchers to visualize brain activity while performing cognitive tasks.
- Brain-Computer Interfaces: These systems create a direct communication pathway between the brain and external devices, informing AI applications about real-time cognitive states.
Understanding how the brain supports cognition allows AI developers to construct more sophisticated models for simulating cognitive functions. This integration fosters the development of AI systems that can predict and respond to user needs more effectively, bridging the gap between human and machine interactions.
AI Algorithms Inspired by Cognitive Psychology
In the realm of artificial intelligence, the influence of cognitive psychology cannot be overstated. AI algorithms inspired by cognitive psychology provide a framework for understanding not only how machines can learn but also how they can mimic human-like reasoning. This intersection fosters the development of more sophisticated AI systems that demonstrate enhanced problem-solving abilities and adaptive learning processes.
AI algorithms derive principles from cognitive psychology to create models that imitate human thought processes. By understanding the nuances of cognition, researchers can design algorithms that better replicate how humans interpret information, make decisions, and solve problems. This alignment between cognitive principles and AI development not only informs the algorithms themselves but also provides insights into user interaction and system behavior.
Neural Networks and Biological Plausibility
Neural networks stand out as a primary example of AI methodologies that draw from cognitive psychology. Designed to simulate the human brainβs functioning, these models consist of interconnected nodes that process information much like neurons. The foundational idea stems from an understanding of how human brains function in learning and memory. Thus, creating networks that reflect biological processes is essential for the credibility and performance of these systems.
The practicality of using neural networks lies in their ability to learn from vast amounts of data. The training process involves adjusting connection weights based on error correction. This mimics how humans learn from experience, tightening the connection with correct answers and weakening erroneous ones. As a result, neural networks excel in tasks like image recognition and natural language processing. However, while significant progress has been made, the biological plausibility of these models remains a topic of ongoing research.
Bayesian Models of Cognition
Bayesian models are another facet of AI that draws parallels with cognitive psychology. These models utilize Bayesian inference to quantify uncertainty in decision-making, representing how humans process information in the face of uncertainty. This aligns closely with cognitive theories that explain human reasoning as a probabilistic process, rather than a deterministic one.
For AI systems, Bayesian approaches enable machines to update their beliefs based on new evidence dynamically. This flexibility allows AI to function effectively in real-world situations where data is incomplete or ambiguous. The capability to adapt, revise, and enhance predictions based on new data makes Bayesian models vital for tasks such as recommendation systems and spam filtering. In essence, incorporating Bayesian principles into AI promotes a deeper understanding of cognitive processes and allows for more human-compatible systems.
Cognitive Architectures: ACT-R and Soar
Cognitive architectures, such as ACT-R and Soar, represent a structured approach to integrating theories of human cognition into AI systems. ACT-R (Adaptive Control of Thought-Rational) is a robust cognitive architecture that combines aspects of human thinking into a computational model. It focuses on alternating between declarative and procedural memory, mirroring how humans use knowledge in different contexts. On the other hand, Soar emphasizes general intelligence and problem-solving abilities, encompassing a variety of cognitive tasks.
Both ACT-R and Soar offer frameworks to investigate and simulate cognitive processes, serving researchers and developers in understanding the dynamics of human thought. The applications of these architectures extend to intelligent tutoring systems, where they provide personalized learning pathways based on cognitive models. As such, they not only advance AI capabilities but also foster a deeper understanding of human cognition itself.
Applications of AI in Cognitive Psychology Research
The intersection of artificial intelligence and cognitive psychology presents intriguing applications that can enhance our understanding of mental processes. Recognizing the impact of AI in this field is crucial for both practical and theoretical advancements. By leveraging AI, researchers can analyze large datasets, simulate cognitive behaviors, and refine psychometric tests. These aspects not only contribute to current research but also pave the way for innovative approaches in understanding human cognition.
One of the most significant benefits is the capability to process data at an unprecedented scale. Traditional methods often fall short due to the volume and complexity of data, but AI systems can efficiently identify patterns that might elude human analysts. This accelerates research and allows for richer insights into cognitive functions.
Additionally, AI tools in cognitive psychology must be approached carefully. Ethical considerations regarding data privacy and algorithmic bias are paramount. Awareness and mitigation of these issues will ensure the responsible use of technology in psychological research.
Data Analysis and Pattern Recognition
The rise of AI technologies has transformed how data is analyzed in cognitive psychology. Researchers now use machine learning algorithms to parse through extensive datasets to uncover subtle patterns in human behavior. This process involves training algorithms to recognize these patterns, which makes it easier to identify correlations that may not be immediately obvious.
For example, by analyzing data from various psychological studies, AI can help in predicting outcomes related to cognitive decline or improved learning processes.
Benefits of AI in data analysis include:
- Improved accuracy in findings
- Faster processing times for large datasets
- Ability to identify complex patterns
Ultimately, the integration of AI tools in this analytical phase enhances the reliability of cognitive research.
Psychometric Testing and AI
Psychometric testing is pivotal for assessing various aspects of human cognition, including personality and intelligence. AI has the potential to revolutionize how these tests are designed and evaluated. By utilizing AI, researchers can implement adaptive testing methods that dynamically adjust to a participant's responses, thereby providing a more accurate measurement of their cognitive abilities.
AI can also assist in scoring these tests by minimizing human error, thereby increasing consistency. Furthermore, neural networks can be trained to analyze patterns in responses, allowing for insights that could tailor psychological assessments to individual needs.
Concerns to consider with AI in psychometric testing:
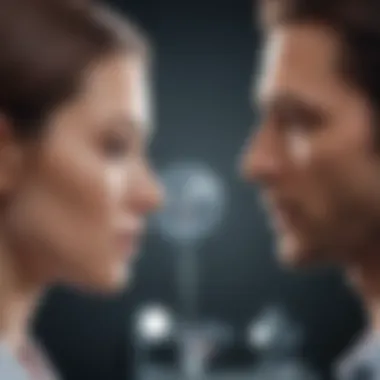

- Transparency in scoring algorithms
- Ensuring fairness and reducing bias
Attention to these factors is essential to maintain the integrity of psychometric evaluations.
Simulation of Cognitive Processes
Simulating cognitive processes through AI is an area that offers vast potential for deeper understanding. Computational models can mimic human cognition, allowing researchers to test hypotheses about cognitive function without the constraints of traditional methods. For instance, simulations can demonstrate how memory formation and retrieval work in real-time.
These simulations can also reveal the effects of various factors, such as distractions or stress, on cognition. This leads to richer hypotheses that can be further explored in practical experiments.
Key advantages of cognitive simulations include:
- Validating cognitive theories
- Experimenting with interventions in a virtual environment
- Exploring atypical cognitive processes, such as in dyslexia or autism
The synthesis of AI and cognitive psychology in simulations provides a canvas for broadening our perspective on human cognition.
Cognitive Models Used in AI Systems
Cognitive models serve as essential frameworks that guide the development and functioning of artificial intelligence systems. These models encapsulate theories derived from cognitive psychology, offering insights into how humans process information and make decisions. By understanding these cognitive functions, AI developers can design systems that are more aligned with human behavior and thinking patterns. Additionally, leveraging cognitive models contributes to the enhancement of user experiences and interfaces.
Understanding User Behavior through AI
Utilizing cognitive models in AI helps in understanding user behavior. AI systems can analyze vast amounts of data regarding how individuals interact with technology. For instance, platforms like Facebook employ algorithms that track user engagement and adapt to their preferences. This alignment with cognitive psychology allows for an improved grasp of decision-making processes in users. These insights enable the creation of more intuitive interfaces that respond dynamically to user needs, fostering a better connection between the user and the machine.
- Example insights from user behavior analysis:
- Frequency of engagement with specific content.
- Patterns in navigation and interaction.
- Preferences based on previous experiences.
The emphasis is on how cognitive models convert behavioral data into actionable insights that inform AI design, making the technology more responsive to user needs.
Enhancing Human-Machine Interaction
Cognitive models provide valuable guidance in enhancing human-machine interaction. By applying principles from human cognition, developers can create more effective and user-friendly AI systems. For instance, when systems utilize natural language processing, they can better comprehend user queries and respond appropriately.
A few key aspects of enhancing interaction include:
- Natural language understanding: AI can comprehend and respond to the complexity of human language.
- Contextual awareness: Systems can adapt their responses based on the situational user context.
- Feedback loops: AI learns from user interactions, improving outcomes over time.
These factors contribute to a more seamless interaction between users and technology, enabling AI systems to feel more like intuitive extensions of the users themselves.
Personalization through Cognitive Insights
Personalization in AI is significantly informed by cognitive models that offer insights into user experiences. By understanding individual cognitive styles, preferences, and behaviors, AI systems can deliver tailored content and recommendations. For example, streaming services like Netflix use algorithms which consider user behaviors to curate individualized viewing suggestions.
- Benefits of personalization:
- Improved user engagement, as content aligns closely with individual interests.
- Enhanced satisfaction as users navigate a more relevant array of options.
- Increased loyalty and retention due to customized experiences.
Through these cognitive insights, AI systems not only adapt to user preferences but also play a pivotal role in elevating the overall experience, making technology more relevant and user-centric.
"The integration of cognitive models in AI systems is crucial for creating technology that resonates with human users, ultimately bridging the gap between human cognition and machine learning."
Ethical Considerations in AI and Cognitive Psychology
The integration of artificial intelligence and cognitive psychology brings vital ethical considerations to the forefront. This intersection is marked not only by opportunities for innovation but also by profound implications for society. Addressing ethical concerns is essential in ensuring that AI applications respect human rights and enhance psychological well-being.
Bias in AI Algorithms
Bias in AI algorithms poses a significant ethical dilemma. Many AI systems are trained on data that reflect existing societal biases. For example, if a psychological assessment tool is trained on a non-diverse dataset, it may lead to unfair treatment of certain groups. This not only raises questions about equality but also affects the validity of the psychological insights gained from AI.
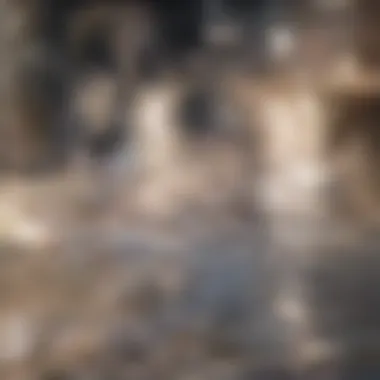
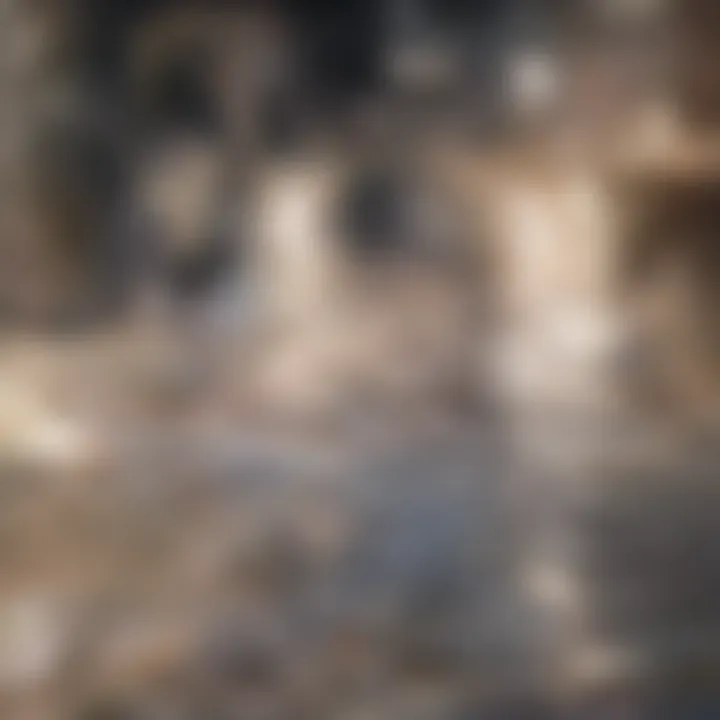
Algorithms designed without appropriate oversight can perpetuate stereotypes and reinforce societal prejudices. Researchers and developers must be mindful of this bias throughout the AI lifecycle, from design to deployment. Incorporating diverse data sets and implementing checks against bias should be priorities in developing AI-driven psychological tools.
Privacy and Data Security
Privacy is an integral component of ethical AI implementation. Sensitive personal data is often required to develop AI systems that analyze cognitive behaviors. The collection, storage, and use of such data raise legitimate concerns regarding how individuals' information is handled. There are cases where data breaches can lead to significant harm.
Organizations must establish robust policies to safeguard user information. Transparency about data usage is also crucial. People should be informed about how their data contributes to AI system development and given options to opt-out if they desire. Ensuring data security encompasses both technological advances and ethical commitments.
"The ethical implications surrounding AI and cognitive psychology must not only address how technology is used but also who it benefits and how inclusively."
Implications for Mental Health Monitoring
The use of AI in mental health monitoring offers tremendous potential but also entails significant ethical considerations. Tools that assess emotional states or predict mental health issues can enhance treatment options. However, they must be used responsibly to avoid stigmatization of those who may seek help.
AI systems must ensure accurate representation and not give rise to misdiagnosis or unwarranted alarms. This relates to the responsibility of professionals in interpreting AI outputs in conjunction with other evaluative measures. Collaboration between AI developers and mental health professionals is essential to maintain ethical standards.
In summary, as AI continues to evolve within the domain of cognitive psychology, ethical considerations are paramount. By acknowledging bias, emphasizing privacy, and considering implications for mental health monitoring, researchers and practitioners can work towards responsible advancements in this rapidly evolving field.
Future Directions of Research
Interdisciplinary Collaboration Opportunities
Collaboration between cognitive psychologists and AI researchers is essential for deeper insights. By joining forces, researchers can create frameworks that benefit both fields. In particular, these partnerships can lead to the development of tools that are more aligned with human cognitive processes. Some potential collaboration opportunities include:
- Joint Research Projects: Collaborative studies can explore the effectiveness of various AI models in simulating human behavior.
- Workshops and Conferences: Regular academic gatherings can facilitate knowledge exchange. Shared data and insights can lead to innovative approaches.
- Cross-disciplinary Training Programs: Educators can develop programs that empower students to grasp both cognitive psychology and AI, fostering a new generation of researchers.
"Collaboration is not just an option; it is a necessity for driving innovation at the intersection of cognitive psychology and AI."
Advancements in AI Technology
The rapid evolution of AI technologies greatly influences cognitive psychology. Advancements in machine learning, particularly deep learning algorithms, provide tools for modeling cognitive processes. Leveraging these technologies can lead to the following benefits:
- Improved Cognitive Models: The implementation of neural networks can simulate complex cognitive tasks, helping researchers understand how the human mind works.
- Enhanced Data Processing: AI technology allows for analyzing large datasets efficiently. This can uncover patterns in cognitive behavior that were previously undetectable.
- Real-world Applications: Enhanced AI systems can be applied in diverse fields, including education, mental health, and user experience research, ultimately offering a deeper understanding of cognitive processes in various contexts.
The Evolving Understanding of Cognition
Research in AI contributes significantly to our evolving understanding of cognition. As AI systems learn and adapt, they mirror cognitive processes. This relationship leads to numerous insights:
- Insights into Human Learning: AI models can reflect how humans learn, enabling researchers to refine learning theories in cognitive psychology.
- Testing Cognitive Theories: AI systems can be used to validate cognitive theories through simulation. This iterative process helps evaluate the robustness of different psychological frameworks.
- Expanding the Definition of Cognition: The collaboration can stretch traditional definitions of cognition, incorporating how machines think alongside humans.
The End
The conclusion serves a pivotal role in this article, encapsulating the essential insights gleaned from the interplay between cognitive psychology and artificial intelligence. By synthesizing the findings discussed, it highlights the significance of understanding cognitive principles when developing AI systems. This intersection not only illuminates the ways in which AI can function effectively, but it also offers a lens through which we can assess the broader implications for cognitive science and psychological research.
A few key elements emerge as particularly noteworthy:
- Integration of AI and Cognitive Psychology: The relationship between these fields fosters a more nuanced understanding of both human cognition and machine learning. Principles from cognitive psychology not only influence the design of AI models but also enhance the interpretability of these systems.
- Benefits of Interdisciplinary Research: Collaborative efforts between cognitive psychologists and AI researchers can lead to innovative approaches in studying cognitive processes. This collaboration bridges gaps and encourages the development of more robust models.
- Considerations for Ethical Practice: The ethical implications of deploying AI in psychological contexts cannot be overlooked. Concerns regarding bias in algorithms, data privacy, and the potential impact on mental health monitoring must be critically examined.
"Understanding the intersection of cognitive psychology and AI fosters advancements not just in technology, but also in ethical considerations of using such technology in real-world applications."
In summary, the conclusion encapsulates the relevance of ongoing dialogue between cognitive psychology and AI, emphasizing how advancements in both can enrich our understanding of human cognition and improve the ethical framework guiding AI applications.
Summary of Key Insights
The insights gathered through this article reveal several critical aspects of the relationship between cognitive psychology and artificial intelligence. Throughout the sections, we have seen that:
- Cognitive Foundations: The theories and models from cognitive psychology provide essential guidance for AI development. The principles of human cognitive processes are foundational to creating systems that can learn and adapt more effectively.
- AI's Role in Experimental Psychology: AI technologies facilitate research methodology, offering tools for data analysis, pattern recognition, and even simulating cognitive tasks.
- Ethical Implications: Continuous attention to biases within AI algorithms and the potential impacts on user mental health is crucial for guiding responsible AI development.
This intersection holds promise not only for technological advancement but also for rethinking approaches to understanding human thought and behavior.
Implications for Ongoing Research
The fusion of cognitive psychology and artificial intelligence leads to numerous opportunities for ongoing research in both fields:
- Exploration of New Cognitive Models: Researchers can delve into cognitive models that explain human behaviors, which in turn can enhance AI learning mechanisms.
- Advancements in Monitoring and Treatment: AI technologies can provide significant advantages in mental health research, allowing for more precise assessments and tailored interventions.
- Emphasis on Ethical AI: Future research must prioritize the identification and mitigation of biases in AI systems, investigating their implications for society. As AI continues to evolve, understanding its effects on cognitive processes should remain a key focus.