Big Data's Impact on Electronic Health Records
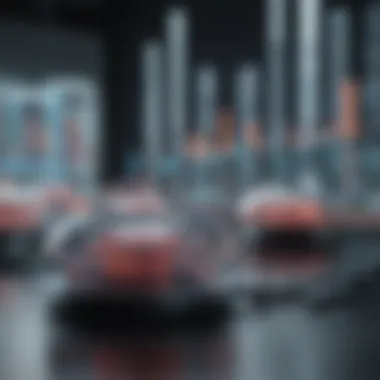
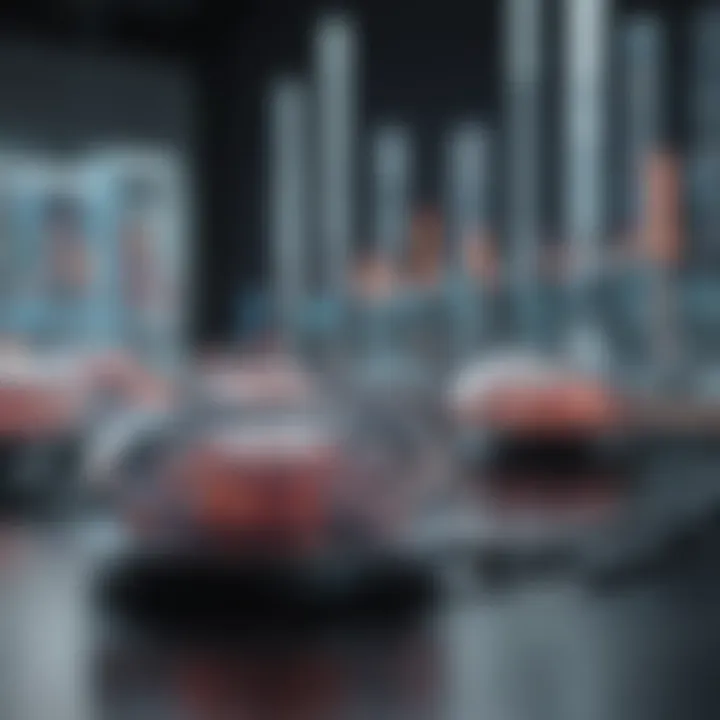
Intro
The field of healthcare is undergoing a significant transformation, substantially driven by the convergence of big data and electronic health records (EHR). As healthcare facilities increasingly rely on comprehensive data systems, understanding the implications of this integration becomes essential. Big data technologies facilitate an unprecedented depth of analysis concerning patient information, treatment outcomes, and operational efficiency. This exploration will shed light on key points of interest in this domain, focusing on the benefits and challenges that accompany such advancements.
The ability to manage vast quantities of health data is not merely beneficial but transformational. It can enhance patient care, inform public health decisions, and streamline operational processes. However, the integration of big data solutions also presents significant hurdles, particularly in areas such as data privacy, interoperability, and predictive analytics. This overview aims to provide a solid foundation for readers, equipping them with the understanding necessary to navigate this complex yet exciting landscape.
With these themes in mind, the subsequent sections will delve into the key findings related to big data in EHR, the methodologies employed to analyze these intersections, and future directions that clinical practice might take using predictive modeling.
Understanding Big Data
As healthcare continues to evolve, the integration of big data into health informatics is increasingly vital. Understanding big data is foundational for grasping how it influences electronic health records (EHR). The implications are profound, and they encompass various facets like enhanced decision-making, operational efficiency, and improved patient outcomes. In this article, we will thoroughly explore the intricacies of big data, particularly in the context of healthcare.
Definition of Big Data
Big data refers to extremely large data sets that become complex and challenging to process using traditional data processing techniques. The breadth of this data spans various formats—structured and unstructured—and covers extensive domains, including demographics, medical history, clinical data, and treatment outcomes. As such, big data signifies not only volume but also the velocity and variety of how information is generated and analyzed. In healthcare, this could include real-time data from wearables, social media interactions, genomic data, and even data from online health forums.
Characteristics of Big Data
To understand big data, one must appreciate its defining characteristics:
- Volume: The sheer amount of data that is produced is staggering, often measured in zettabytes.
- Velocity: Data flows in at an unprecedented speed, requiring real-time processing capabilities.
- Variety: Data comes in numerous formats—from videos and images to text and structured data.
- Veracity: The reliability and accuracy of data can often vary, needing close scrutiny and validation.
- Value: Extracting meaningful insights from big data is essential for decision-making.
These characteristics are crucial when considering how they impact EHR systems.
Sources of Big Data in Healthcare
Big data in healthcare arises from multiple sources, including:
- Electronic Health Records: Digital records serve as a primary source, containing comprehensive patient information.
- Clinical Trials: Data collected during trials contribute significant insights for health research.
- Wearable Devices: Gadgets like fitness trackers relay real-time health information, enriching existing datasets.
- Social Media: Posts and interactions on platforms can provide valuable patient feedback and trends.
- Genomic Data: The explosion of genomic sequencing has resulted in vast amounts of genetic information.
Through these sources, the integration and utilization of big data drive advancements in patient care and health management. By thoroughly understanding big data, stakeholders in the healthcare system can make informed decisions, ultimately enhancing the effectiveness of EHRs and patient outcomes.
Electronic Health Records Overview
The importance of electronic health records (EHR) in healthcare cannot be overstated. They serve as a digital version of a patient's paper chart, streamlining patient data management and improving the overall quality of care. EHR systems not only enhance the accuracy and clarity of patient information but also facilitate efficient communication among healthcare providers. This overview delves into the definition, purpose, components, and historical context of EHR. By understanding these elements, readers can appreciate the role EHR plays within the broader context of big data in healthcare.
Definition and Purpose
Electronic Health Records are comprehensive digital records that collect and store a patient's medical history. The primary purpose of EHR systems is to provide healthcare professionals with immediate access to patient data. This instant access supports better clinical decisions, reduces errors, and enhances patient safety.
EHR simplifies history-taking during consultations. Clinicians can review past diagnoses, past treatments, and medications. In addition, EHR enables healthcare providers to track improvements in patient health through digital means, allowing for long-term monitoring. Furthermore, it supports efficient billing processes by maintaining simultaneous access to clinical and financial records.
Components of EHR
EHR systems consist of several key components that work together to function effectively. These components include:
- Patient Demographics: Basic information about the patient like name, address, contact info, and insurance details.
- Medical History: Comprehensive records encompassing diagnoses, previous treatments, allergies, and immunizations.
- Medication Records: Details regarding current prescriptions, dosages, and prescribing history.
- Laboratory and Imaging Results: Access to blood work, x-rays, and other diagnostic results.
- Progress Notes: Documentation made by healthcare providers detailing patient visits and treatment plans.
- Billing Information: Information that integrates with insurance and finance for billing purposes.
These components are crucial in creating a complete view of a patient’s health status, enabling healthcare professionals to deliver informed and effective care.
History and Evolution of EHR
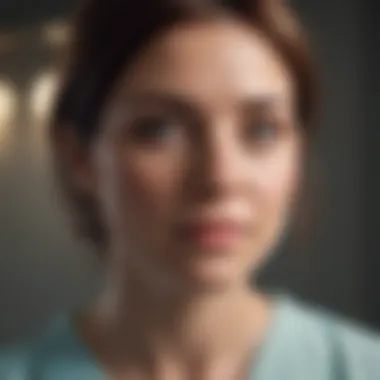
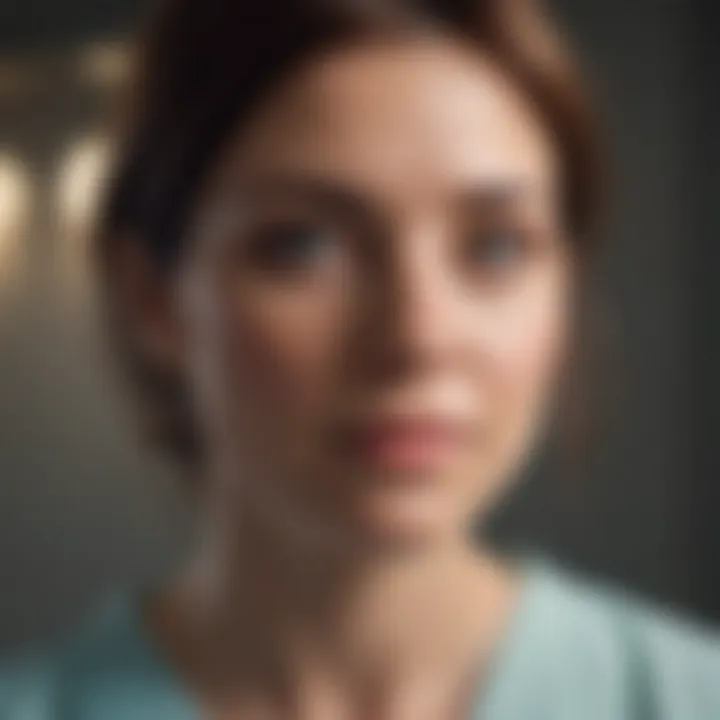
The evolution of EHRs has occurred over several decades. Initially, patient data was kept in paper files managed by individual practices. This made information retrieval both slow and prone to error. The shift towards electronic records began in the 1960s, coinciding with advancements in computer technology.
In the 1980s, pilot programs were developed to explore the benefits of EHR. By the early 2000s, governmental and private sector interests pressed for wider adoption through mandates and incentives, such as those outlined in the Health Information Technology for Economic and Clinical Health (HITECH) Act.
The current landscape witnesses integration of advanced data analytics with EHR. As a result, healthcare practitioners can leverage big data insights to improve patient care and operational outcomes. EHR continues to evolve with further advancements in technology, aiming to provide more personalized care in the future.
In summary, electronic health records have transitioned from traditional paper methods to comprehensive digital systems that enhance patient care through better data management and accessibility.
The Intersection of Big Data and EHR
The integration of Big Data into Electronic Health Records (EHR) represents a significant advancement in the realm of healthcare. This intersection facilitates more informed decision-making processes, enhances operational efficiencies, and ultimately optimizes patient care. By leveraging the vast amounts of data generated within healthcare systems, professionals can extract valuable insights that can guide clinical practices and improve health outcomes. The importance of this topic is multifaceted. First, it addresses the need for effective data integration that enhances accessibility for healthcare providers. Second, it explores how sophisticated analytics tools derived from Big Data can support better clinical decisions. Lastly, it evaluates the overall impact on patient health, emphasizing the shift towards data-driven healthcare.
Data Integration and Accessibility
Big Data technologies enable the integration of various data sources, which is crucial for EHR systems. Effective integration allows for the seamless aggregation of patient data from diverse sources, such as laboratory results, clinical notes, and imaging studies. The result is a comprehensive view of an individual's health that providers can access in real-time.
- Challenges exist in standardizing data formats and ensuring interoperability between different EHR systems. The lack of uniformity can hinder data accessibility, thus impacting patient care.
- To achieve successful data integration, healthcare organizations may implement platforms that support application programming interfaces (APIs) to facilitate data exchange between systems.
As a result, data accessibility enhances the potential for more coordinated care delivery, enabling healthcare teams to work collaboratively and respond to patient needs swiftly.
Enhanced Decision-Making Tools
The deployment of analytical tools within EHR systems profoundly affects decision-making. Big Data analytics can categorize patient information and analyze it to uncover patterns that might not be evident through traditional methods. For instance, predictive analytics can anticipate potential health issues before they escalate.
- Descriptive analytics summarizes historical data, making it easier to understand patient trends and outcomes.
- Predictive analytics uses historical data to forecast future health scenarios, allowing practitioners to implement preventative measures.
- Prescriptive analytics goes a step further by recommending specific actions based on data analysis.
The blend of these analytics provides healthcare professionals with comprehensive insights that ultimately lead to improved patient management and more targeted treatments.
Improving Patient Outcomes
The utilization of Big Data within EHR systems is largely aimed at improving patient outcomes. When healthcare providers can access integrated data and enhanced decision-making tools, the likelihood of achieving better health results increases.
- Data-driven insights empower clinicians to tailor treatments to individual patients, fostering a more personalized approach to care.
- Monitoring health trends through big data analytics allows for the identification of at-risk populations. Consequently, targeted interventions can be designed and implemented.
By prioritizing both preventive and responsive care strategies, the healthcare system can transition towards a model that supports holistic patient health over mere symptoms management. In summary, the intersection of Big Data and EHR not only enhances the efficiency of healthcare practices but also significantly uplifts the standards of patient care.
Challenges and Limitations
Understanding the challenges and limitations in the integration of big data and electronic health records (EHR) is essential for any stakeholders in the healthcare sector. These challenges are interconnected and can significantly impact the efficacy of data utilization for improving patient outcomes. Awareness of these constraints allows healthcare practitioners and policy makers to devise solutions that enhance data management and application while safeguarding patient interests.
Data Privacy and Security Concerns
The issue of data privacy and security markedly influences how big data is used within EHR systems. Healthcare data is sensitive, containing personal details that require stringent security measures. Breaches can lead to severe consequences for individuals and organizations, prompting the necessity for effective cybersecurity protocols.
Due to the vast volume of data being collected, traditional methods of security are often inadequate. Therefore, healthcare facilities must employ advanced encryption techniques and access controls. Regulatory frameworks such as the Health Insurance Portability and Accountability Act (HIPAA) serve to protect patient information. However, these rules must evolve continually to address emerging threats in the digital landscape.
The challenge is not only technical. Staff training is also essential to maintain a culture of privacy awareness. Thus, implementing a holistic strategy that covers both technology and human factors is crucial for minimizing risks in data handling.
Interoperability Issues
Interoperability remains a core challenge when integrating big data into EHR. This concept refers to the ability of different IT systems, devices, and applications to communicate and exchange data seamlessly. In many instances, healthcare systems are built on disparate technologies that do not support easy data sharing.
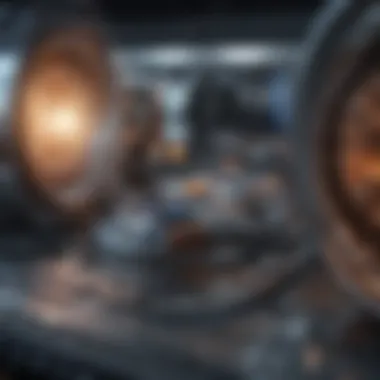
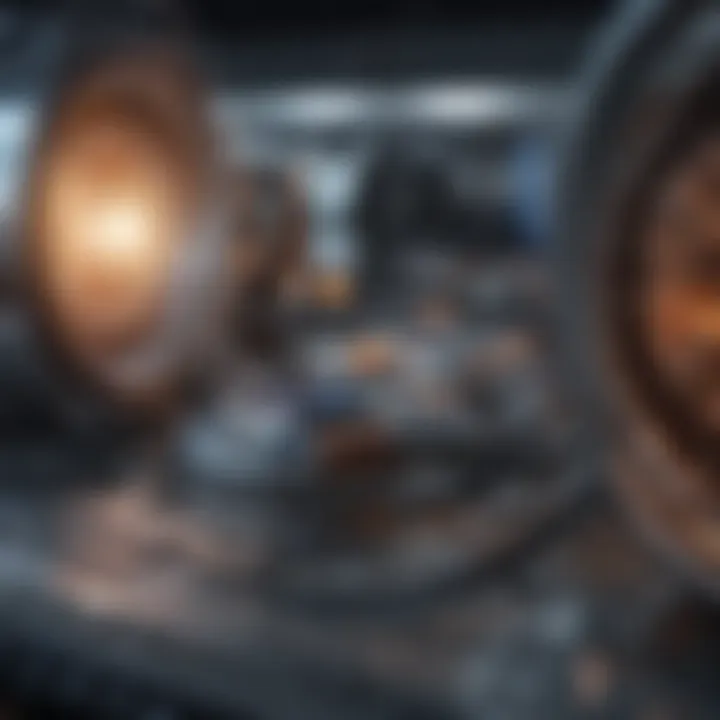
The consequences of poor interoperability can be significant. Fragmented data can lead to inaccurate patient information, which in turn can negatively affect clinical decision-making. The absence of standardized formats exacerbates this issue, creating obstacles for data integration from multiple sources such as laboratories, pharmacies, and other healthcare providers. Developing and adhering to industry-wide standards is therefore imperative.
Efforts for creating interoperable systems involve investments in new technologies and collaborative frameworks among stakeholders. This is a long-term process but is essential for realizing the full potential of big data in enhancing patient care and operational effectiveness.
Data Quality and Standardization
Data quality and standardization are closely related to the effectiveness of big data analytics in EHR systems. The quality of data directly impacts analysis outcomes, which further affects treatment plans and healthcare decisions.
Healthcare data can be inconsistent, incomplete, or outdated. For instance, when patient information is entered manually, there is a heightened risk of human error. Furthermore, variations in how data is recorded across systems can lead to challenges in aggregation and analysis.
Establishing uniform data standards is critical to ensuring that the information collected is accurate and reliable. This involves collaboration among providers, technology vendors, and regulatory bodies to create comprehensive guidelines. Data cleaning practices should also be in place to regularly assess and refine the datasets being utilized.
Utilizing Big Data Analytics in EHR
Big data analytics has emerged as a crucial component in optimizing electronic health records (EHR). The ability to analyze vast amounts of data allows healthcare professionals to derive valuable insights that can enhance patient care. This segment explores the different types of analytics utilized within EHR systems, examines real-world case studies, and discusses the role of machine learning in transforming healthcare outcomes.
Types of Analytics: Descriptive, Predictive, and Prescriptive
In the realm of healthcare, three primary types of analytics can be identified: descriptive, predictive, and prescriptive.
- Descriptive analytics focuses on summarizing historical data. This includes parameters such as patient demographics, treatment outcomes, and readmission rates. By interpreting this data, healthcare providers can identify trends that might inform practice improvements.
- Predictive analytics goes a step further by employing statistical algorithms to forecast future outcomes based on historical data. For instance, predictive models can estimate which patients are at higher risk for certain conditions, allowing for targeted interventions.
- Prescriptive analytics is the most advanced form, recommending actions based on predictive insights. This type of analytics not only identifies potential future outcomes but also suggests specific actions to optimize patient care and resource allocation.
Understanding these types of analytics is essential for leveraging big data effectively within EHR frameworks.
Case Studies in Big Data Analytics
Several case studies highlight the successful application of big data analytics in EHR systems. For example, a study at Mount Sinai Health System showed reduced hospital readmission rates through data-driven discharge planning. By analyzing readmission patterns, health professionals developed tailored care plans.
Another notable instance is the use of analytics at Intermountain Healthcare, where predictive modeling helped in early identification of patients at risk for complications. This proactive approach led to improved patient outcomes and optimized resource utilization.
These cases illustrate how big data analytics can drive effective decision-making within EHR environments.
Role of Machine Learning in Healthcare
Machine learning plays a transformative role in healthcare, particularly within EHR systems. Unlike traditional programming, machine learning algorithms can learn from data and make decisions with minimal human intervention.
- For example, natural language processing allows for sifting through unstructured data in EHRs, such as clinical notes or patient histories, aiding in better understanding patient conditions.
- Furthermore, machine learning models enhance predictive analytics, allowing healthcare organizations to identify at-risk patients more accurately. These models adapt and refine themselves based on new data, ensuring continuous improvement over time.
The integration of machine learning with big data analytics presents vast opportunities for enhancing patient care and improving operational efficiency.
Regulatory and Ethical Considerations
In the context of Big Data and Electronic Health Records (EHR), regulatory and ethical considerations are critical. These elements help ensure that patient data is handled responsibly while maximizing the benefits of data analytics. As healthcare continues to evolve towards digital solutions, understanding the legal frameworks and ethical obligations becomes paramount for practitioners and organizations alike.
The integration of vast amounts of data in healthcare requires clear regulations to govern the ways in which this data is collected, used, and shared. Compliance with such regulations not only protects patient privacy but also fosters trust in healthcare systems. Incorporating ethical consideration into the handling of big data further seeks to balance innovation with patient rights. It is essential for healthcare professionals to navigate these frameworks effectively to avoid potential legal repercussions and ethical dilemmas.
Health Insurance Portability and Accountability Act (HIPAA)
The Health Insurance Portability and Accountability Act, or HIPAA, is a cornerstone of patient privacy law in the United States. Enacted in 1996, it establishes standards for protecting sensitive patient information while allowing for necessary information sharing among healthcare providers.
Key aspects of HIPAA relevant to big data and EHR include:
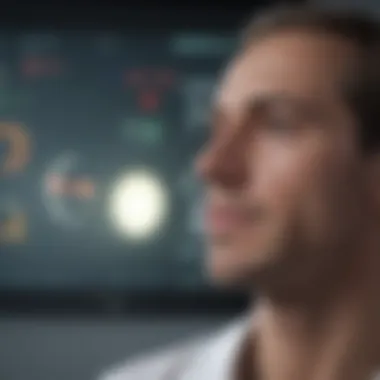
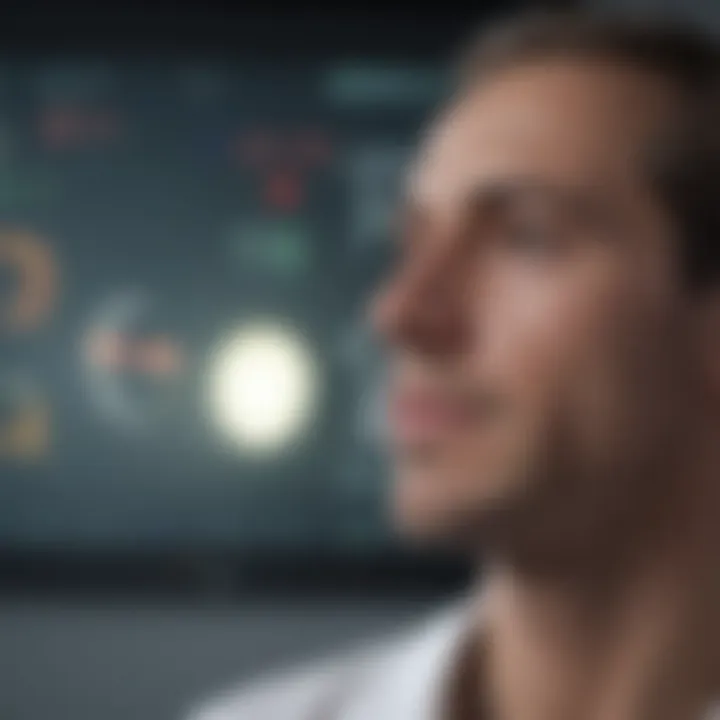
- Privacy Rule: This rule mandates how personal health information (PHI) should be protected. It ensures that patients have rights over their health information and outlines how it can be disclosed.
- Security Rule: This component requires healthcare organizations to implement safeguards to protect electronic health information against unauthorized access.
- Transaction and Code Sets Rule: This rule standardizes the coding and format of health data for electronic transactions.
Organizations utilizing big data analytics must comply with HIPAA when processing personal health data. Failure to do so can result in significant penalties, both financial and reputational, undermining the trust patients place in their healthcare providers.
Ethical Implications of Big Data Use
The application of big data analytics in EHR presents several ethical implications. Balancing innovation with ethical responsibilities is essential to ensure that patient care remains the primary focus. Some ethical considerations include:
- Patient Consent: Patients should be informed about how their data will be used. Consent processes need to be clear, enabling patients to make informed choices regarding their information.
- Data Security: With increasing reliance on big data, organizations face heightened risk of breaches. Ensuring robust cybersecurity measures is not only an ethical responsibility but a legal obligation under HIPAA.
- Fairness and Bias: Algorithms used in analytics can inadvertently perpetuate biases present in training data. It is essential for healthcare organizations to actively work towards eliminating bias to ensure equitable care for all patients.
Efforts to address these ethical issues involve ongoing education, transparent practices, and adherence to legal standards.
"Ethical data use can enhance the overall quality of healthcare while safeguarding the rights of patients."
Consistent evaluation of ethical frameworks is necessary as technology progresses. By adhering to these regulatory and ethical standards, healthcare professionals can harness the power of big data responsibly, ultimately improving patient outcomes.
The Future of Big Data in EHR
The advent of big data technologies marks a new era for electronic health records (EHR). These technologies are not just tools; they redefine how healthcare professionals interact with patient data. Understanding how big data can integrate with EHR is essential for improving patient care and operational efficiency.
One of the main advantages of utilizing big data in EHR is the potential to enhance decision-making. As more data becomes available, healthcare providers can access a wide array of information. This leads to more informed choices. Big data analytics can process and analyze massive datasets in real-time, allowing for quicker diagnoses and targeted treatments. As such, it significantly impacts patient outcomes by enabling more personalized care paths.
However, implementing these technologies requires a clear understanding of various considerations. Professionals must evaluate the costs and benefits associated with integrating big data into existing systems. They also need to consider training staff to utilize these tools effectively and ethically.
"The capacity to harness patient data through advanced analytics allows for a deeper understanding of healthcare needs and trends."
Trends in Big Data Technologies
Several trends in big data technologies are reshaping the future of EHR. Key among these is the rise of cloud computing. The cloud offers scalable storage solutions for healthcare providers. This means that vast amounts of data can be stored and processed without the need for extensive on-site infrastructure. Moreover, cloud technologies can facilitate collaboration between various stakeholders in healthcare, including doctors and researchers, thereby leading to better information sharing and coordination.
Another trend is the increased use of Artificial Intelligence and Machine Learning in big data analytics. These technologies streamline processes by identifying patterns in data that would otherwise remain unnoticed. Predictive analytics generated through machine learning can foresee patient events, such as hospital readmissions, resulting in preventive measures. This capability showcases the intersection of big data and EHR, focusing on improving patient management and operational workflows.
The integration of real-time analytics is another prominent development. Tools that allow for the examination of data as it flows into the system can enable healthcare providers to make immediate decisions. This speed is crucial in emergencies and can drastically impact life-saving measures.
Potential for Personalized Medicine
Personalized medicine is a burgeoning field that relies heavily on big data. By analyzing genetic information and previous patient data, healthcare providers can tailor treatment plans specifically to individual patients. This contrasts sharply with the traditional one-size-fits-all approach to medicine.
The potential for personalized treatment extends to many areas, such as oncology and chronic disease management. For instance, oncology treatments can be customized based on a patient's unique genetic profile, improving effectiveness and minimizing side effects. Similarly, chronic conditions like diabetes can be managed with real-time data from wearable devices, adjusting treatment protocols based on daily metrics.
Personalized medicine not only improves patient outcomes but also enhances patient satisfaction. When patients feel that their care is tailored to their needs, their engagement increases. This engagement leads to better adherence to treatment protocols and a proactive approach to health management.
Finale
The integration of big data into electronic health records (EHR) represents a pivotal change in healthcare management. This section underscores the importance of understanding the implications of combining these elements. The intersection of big data with EHR involves a multitude of benefits, such as enhanced data-driven decision-making, improved patient care, and streamlined operational efficiency.
In this article, we have examined how big data analytics can transform traditional healthcare practices. The potential for predictive modeling, improved patient outcomes, and tailored treatments cannot be overstated. Importantly, the challenges associated with data privacy, interoperability, and data quality must also be discussed. Balancing the vast opportunities presented by big data with the ethical and regulatory frameworks governing health data is crucial for sustainable progress in healthcare.
"Big data will continue to shape the future of healthcare, but careful consideration of its ethical use and potential impacts remains essential."
Overall, summarizing the key insights can guide stakeholders in making informed decisions regarding the future use of big data in EHR systems.
Summary of Key Insights
- Impact on Patient Care: Big data greatly enhances the capacity for personalized medicine, tailoring patient care based on data-driven insights.
- Operational Efficiency: The utilisation of big data streamlines healthcare operations, resulting in cost savings and better allocation of resources.
- Challenges: Addressing ethical and regulatory concerns, particularly around privacy, is crucial to fully leverage the capabilities of big data without compromising patient trust.
- Future Trends: Emerging technologies such as machine learning will likely play a significant role in further integrating big data into healthcare practices.
Recommendations for Healthcare Practitioners
- Embrace Data Literacy: It is vital for healthcare practitioners to develop a strong understanding of data analytics, fostering a culture of evidence-based decision-making.
- Invest in Training: Regular training sessions on big data tools and compliance protocols can help healthcare teams adapt to changing technology landscapes.
- Prioritize Data Security: Establish robust security measures to protect patient information and ensure compliance with regulations such as HIPAA.
- Promote Interoperability: Advocate for standards that enhance the interoperability of EHR systems, which can facilitate smoother data integration from various sources.
- Stay Informed: Keeping abreast of the latest advancements in big data technologies can help practitioners make informed choices that improve patient care.